Voice Biomarkers in Healthcare Triage
Discover how voice biomarker technology analyzes speech patterns to detect clinical indicators, improve triage efficiency, and transform early diagnosis across a range of medical conditions.
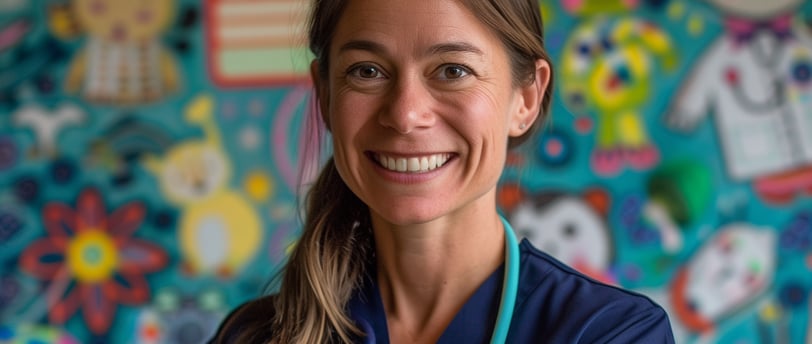
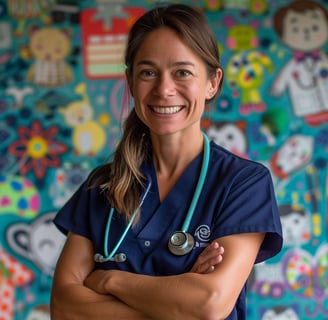
A healthcare system that can detect illness from the way you speak might sound like science fiction, but voice biomarker technology is rapidly turning this concept into reality. The subtle nuances in our speech—variations in pitch, rhythm, articulation, and even microsecond pauses—contain a wealth of information about our physical and mental health that trained human ears might miss. These voice biomarkers are revolutionizing the triage process, allowing healthcare providers to detect clinical indicators through speech patterns with remarkable accuracy and minimal patient effort. As artificial intelligence and machine learning continue to advance, voice analysis is becoming an increasingly powerful tool in the healthcare arsenal, offering non-invasive, rapid, and cost-effective screening for a wide range of conditions. This article explores the fascinating intersection of voice technology and healthcare triage, examining how the words we speak—and the way we speak them—may soon become as diagnostically valuable as laboratory tests or physical examinations.
Understanding Voice Biomarkers: Definition and Science
Voice biomarkers are measurable and objective indicators within speech patterns that correlate with specific health conditions or physiological states. These biomarkers encompass a broad spectrum of vocal characteristics, including acoustic features (pitch, volume, resonance), linguistic elements (word choice, sentence structure, speech rate), and paralinguistic aspects (emotional tone, hesitations, breathiness). The science behind voice biomarkers lies in the intricate connection between our neurological, respiratory, and muscular systems, all of which contribute to the complex process of speech production. When disease or dysfunction affects any of these systems, subtle but detectable changes emerge in the voice. For instance, reduced dopamine levels in Parkinson's disease affect the muscles involved in speech, resulting in characteristic vocal patterns that can be identified through sophisticated analysis. Similarly, the cognitive load associated with mental health conditions can manifest in altered speech timing, prosody, and linguistic choices that reveal underlying psychological states. This scientific foundation has enabled researchers to develop algorithms that can identify specific voice signatures associated with various clinical conditions, creating a new frontier in diagnostic medicine.
The Technological Framework Behind Voice Analysis
The technological infrastructure that powers voice biomarker analysis represents a convergence of several cutting-edge fields, including digital signal processing, machine learning, natural language processing, and clinical informatics. At its core, the process begins with high-quality audio capture, often through specialized applications on smartphones or dedicated recording devices that minimize background noise and optimize sampling rates. Once captured, the raw audio undergoes pre-processing to normalize volume levels, remove artifacts, and segment speech into analyzable units. Feature extraction algorithms then identify hundreds or even thousands of parameters within the voice sample, ranging from fundamental frequency variations to spectral characteristics, jitter, shimmer, and harmonic-to-noise ratios. These extracted features serve as inputs for sophisticated machine learning models—typically deep neural networks or ensemble classifiers—that have been trained on large datasets of voices from both healthy individuals and those with specific conditions. The models identify patterns that discriminate between clinical states with increasing accuracy as they process more data. The final component involves clinical integration, where the analysis results are presented in an interpretable format for healthcare providers, often accompanied by confidence intervals and comparative norms. This technological sophistication enables the detection of subtle vocal changes imperceptible to the human ear, transforming ordinary speech into a powerful diagnostic medium.
Clinical Applications of Voice Biomarkers in Triage
In the high-pressure environment of medical triage, where rapid assessment directly impacts patient outcomes, voice biomarker technology offers unprecedented advantages. Emergency departments are beginning to implement voice analysis systems that can quickly screen incoming patients for conditions requiring immediate attention, such as respiratory distress, cognitive impairment, or severe pain, based solely on their verbal responses to standard intake questions. Primary care settings are utilizing voice biomarkers during telephone consultations or telehealth visits to gather preliminary diagnostic information before patients even arrive at the clinic. This pre-screening capability allows for more efficient allocation of healthcare resources and prioritization of cases based on objective severity indicators rather than subjective reporting alone. In remote healthcare scenarios, particularly in underserved or rural areas, voice biomarker technology enables preliminary assessment without requiring specialized equipment or in-person evaluation. The technology also shows promise in monitoring chronic conditions, with periodic voice samples revealing subtle disease progression or treatment efficacy that might otherwise go undetected between scheduled clinical visits. As integration with electronic health records advances, voice biomarker data can be longitudinally tracked to establish personalized baselines and detect meaningful deviations that warrant clinical intervention. This multifaceted utility in triage contexts demonstrates how voice analysis is transcending its novelty status to become an essential component of modern healthcare delivery systems.
Voice Biomarkers for Mental Health Assessment
The domain of mental health presents both unique challenges for traditional diagnostic approaches and remarkable opportunities for voice biomarker applications. Depression, anxiety, post-traumatic stress disorder, and other psychiatric conditions often manifest in characteristic vocal patterns long before patients may recognize or report their symptoms. Voice analysis can detect the flattened affect and reduced prosodic variation typical of depression, the increased rate and tension markers common in anxiety disorders, or the distinctive speech patterns associated with psychotic states. In crisis intervention settings, real-time voice analysis can help triage workers assess suicide risk through identification of specific linguistic and acoustic features correlated with acute suicidal ideation. For conditions like bipolar disorder, voice biomarkers can track subtle shifts between manic and depressive states, potentially alerting clinicians to impending mood transitions before they become clinically apparent. The non-intrusive nature of voice analysis is particularly valuable in mental health contexts, where traditional assessment methods rely heavily on self-reporting and where stigma may prevent patients from fully disclosing their experiences. Early research has demonstrated that some voice-based algorithms can identify markers of depression with accuracy comparable to standard clinical questionnaires, but with the additional benefit of detecting subclinical states that might warrant preventive intervention. As these technologies continue to evolve, they offer the prospect of more objective mental health assessment tools that complement clinical judgment and potentially reduce diagnostic disparities across different demographic groups.
Voice Patterns in Neurological Disorder Detection
Neurological disorders frequently manifest in speech long before other symptoms become apparent, making voice biomarkers particularly valuable for early detection and monitoring. Parkinson's disease, often diagnosed years after neurodegeneration has begun, produces characteristic changes in speech including reduced volume (hypophonia), imprecise articulation, and monotone quality that can be detected through voice analysis at subclinical stages. Alzheimer's disease and other forms of dementia reveal themselves through linguistic patterns such as reduced vocabulary complexity, increased use of filler words, and distinctive pause patterns that reflect underlying cognitive processing difficulties. Multiple sclerosis can cause subtle dysarthria (difficulty articulating) that sophisticated voice analysis can identify before it becomes perceptible to untrained listeners. In the aftermath of stroke, voice biomarkers can track rehabilitation progress by measuring improvements in articulation precision and prosodic control that correlate with neural recovery. For conditions like amyotrophic lateral sclerosis (ALS), voice degradation patterns provide valuable prognostic information and help guide timely interventions to maintain communication abilities. The sensitivity of voice biomarker technology to subtle neurological changes offers new possibilities for earlier intervention, more personalized treatment approaches, and more accurate monitoring of disease progression or treatment response. As longitudinal datasets grow larger, researchers are identifying vocal precursors that may appear years before clinical diagnosis, potentially opening windows for preventive therapies during presymptomatic stages of neurological disease.
Respiratory Condition Assessment Through Voice Analysis
The intimate connection between the respiratory system and voice production makes speech analysis particularly effective for detecting and monitoring pulmonary conditions. In COVID-19 triage scenarios, voice biomarkers have demonstrated promising results in identifying characteristic patterns associated with infection, including subtle respiratory effort changes, phonation alterations, and distinctive cough signatures. Chronic obstructive pulmonary disease (COPD) manifests in voice through reduced breath support, altered rhythmic patterns, and increased breathiness that can be quantified and tracked over time to assess disease progression or treatment efficacy. Asthma exacerbations produce detectable changes in vocal quality, particularly during periods of bronchoconstriction, allowing for potential early warning systems based on regular voice samples. In sleep apnea assessment, voice biomarkers collected during waking hours have shown correlations with disorder severity, potentially offering a simpler screening method than traditional sleep studies. For pneumonia and other acute respiratory infections, changes in vocal resonance related to fluid accumulation in the lungs can be detected through sophisticated acoustic analysis. The non-invasive nature of voice sampling makes it particularly valuable for vulnerable populations like the elderly or those with compromised immune systems, where traditional respiratory assessment might pose infection risks. As respiratory health continues to receive heightened attention in the post-pandemic era, voice biomarker technology offers a scalable approach to population-level respiratory monitoring that could help identify emerging threats and guide public health responses with unprecedented speed and precision.
Implementation Challenges and Ethical Considerations
Despite its promising applications, the integration of voice biomarker technology into mainstream healthcare faces substantial implementation hurdles and ethical concerns. Technical challenges include ensuring consistent audio quality across different recording environments, devices, and demographic groups to prevent systematic biases in analysis. Algorithm validation requires large, diverse datasets that represent the full spectrum of linguistic variations, accents, and cultural speech patterns to avoid performance disparities across different populations. Privacy concerns are particularly acute with voice data, which can contain identifiable information beyond the acoustic features relevant to clinical assessment, necessitating robust anonymization protocols and transparent data governance frameworks. Regulatory uncertainty remains regarding the classification and validation requirements for voice-based diagnostic tools, with health authorities worldwide still developing appropriate frameworks for this emerging technology. Patient acceptance varies significantly, with some individuals embracing the convenience of voice-based assessment while others express discomfort with the perceived intrusiveness of continuous voice monitoring. There are also legitimate concerns about potential unintended consequences, such as the risk of overdiagnosis or inappropriate clinical actions based on false positive results from voice analysis. Healthcare systems must address workflow integration challenges to ensure that voice biomarker data enhances rather than complicates clinical decision-making processes. These multifaceted challenges underscore the need for thoughtful implementation strategies that prioritize clinical validity, equity, transparency, and patient autonomy as voice biomarker technology continues to evolve from research innovation to clinical reality.
The Future of Voice Biomarkers in Healthcare
The trajectory of voice biomarker technology points toward a future healthcare landscape where speech analysis becomes as routine as checking vital signs. Emerging research is expanding beyond isolated voice samples to continuous passive monitoring through smart devices, potentially enabling the detection of subtle health changes in daily life before they become clinically apparent. Multimodal integration represents another frontier, with voice biomarkers combined with facial expression analysis, movement patterns, and physiological sensors to create comprehensive digital phenotypes that provide holistic health insights. Personalized medicine approaches are being enhanced through longitudinal voice tracking that establishes individual baselines and detects meaningful deviations specific to each patient's unique vocal signature. Federated learning techniques are enabling collaborative algorithm development across institutions without compromising patient privacy, accelerating the validation of voice biomarkers for increasingly specific clinical applications. As artificial intelligence continues to advance, the granularity of voice analysis is reaching new levels of sophistication, potentially enabling differentiation between subtypes of conditions or prediction of treatment response based on vocal characteristics. The democratization of healthcare through smartphone-based screening tools powered by voice analysis could dramatically improve access in underserved regions, particularly for conditions where traditional diagnostics are prohibitively expensive or unavailable. While significant work remains to fully validate and implement these technologies, the convergence of increasing computational power, expanding datasets, and refining algorithms suggests that the voice will soon take its place alongside established biomarkers as an essential diagnostic tool in the healthcare arsenal.
Conclusion
The evolution of voice biomarker technology represents a significant paradigm shift in healthcare triage and diagnostic approaches. By harnessing the rich clinical information embedded within our speech patterns, clinicians are gaining access to an entirely new dimension of patient assessment that is simultaneously non-invasive, cost-effective, and remarkably informative. The applications across mental health, neurology, respiratory medicine, and general triage demonstrate the versatility of this emerging technology. However, as with any transformative innovation, progress must be tempered with careful consideration of implementation challenges, validation requirements, and ethical implications. Voice biomarkers will not replace traditional medical assessment but rather complement existing approaches, providing additional data streams that enhance clinical decision-making and potentially identify conditions at earlier, more treatable stages. As artificial intelligence continues to advance and datasets grow more comprehensive, we can anticipate even greater precision in voice-based diagnostics and expanded applications across the healthcare spectrum. The human voice—our most natural form of expression—may soon become one of medicine's most valuable diagnostic tools, enabling a healthcare system that quite literally listens more attentively to patients and derives deeper meaning from what it hears.
Frequently Asked Questions
What exactly are voice biomarkers?
Voice biomarkers are measurable characteristics in speech patterns—such as changes in pitch, rhythm, articulation, or linguistic choices—that correlate with specific health conditions or physiological states. These objective indicators can be detected and analyzed through advanced algorithms to provide insights into a person's health status without invasive testing.
How accurate are voice biomarkers compared to traditional diagnostic methods?
The accuracy of voice biomarkers varies by condition, but studies have shown promising results with some voice analysis algorithms achieving diagnostic accuracy comparable to standard clinical tests for certain conditions. For example, voice-based detection of Parkinson's disease has reached accuracy levels above 90% in controlled studies, though real-world implementation typically shows more moderate performance.
Can voice biomarkers detect conditions before symptoms are noticeable to patients?
Yes, in many cases voice changes precede other symptoms or patients' awareness of their condition. Research has demonstrated that subtle vocal alterations can be detected by algorithms months or even years before clinical diagnosis for conditions like Parkinson's disease, cognitive impairment, and certain mental health disorders.
What types of conditions can be detected through voice analysis?
Voice biomarkers have shown utility across a wide spectrum of conditions, including neurological disorders (Parkinson's, Alzheimer's), mental health conditions (depression, anxiety, PTSD), respiratory diseases (COPD, COVID-19), cardiovascular issues, and even metabolic disorders like diabetes that affect multiple body systems.
How is my privacy protected when using voice biomarker technology?
Responsible implementation of voice biomarker technology includes robust data security measures, clear consent processes, and often involves analyzing only the relevant acoustic features rather than storing actual voice recordings. Many systems process data locally on devices rather than transmitting full recordings to external servers.
Will voice biomarkers replace my doctor?
No, voice biomarkers are designed to augment rather than replace clinical judgment. They provide an additional layer of objective information to support healthcare professionals in making more informed decisions, particularly in triage settings where rapid assessment is crucial.
Do accents or speaking different languages affect voice biomarker accuracy?
Yes, linguistic and cultural variations can impact voice analysis results, which is why developers are increasingly training algorithms on diverse datasets. Most advanced systems now account for linguistic differences, though this remains an active area of development to ensure equitable performance across all populations.
Can I use voice biomarker technology at home?
Consumer applications are emerging that allow for at-home voice analysis through smartphones or smart speakers, though these typically have more limited capabilities than clinical-grade systems. As the technology matures, more sophisticated home monitoring options are likely to become available with appropriate medical oversight.
How long does it take to get results from voice biomarker analysis?
Analysis time varies by application, but most modern voice biomarker systems deliver results within seconds or minutes after a voice sample is recorded. This rapid assessment capability is one of the key advantages in triage settings compared to laboratory tests that may take hours or days.
Are voice biomarkers covered by insurance?
Insurance coverage for voice biomarker technology is still evolving. While some applications have received regulatory approval and established reimbursement pathways, many are still considered experimental. As clinical validation strengthens and cost-benefit advantages become clearer, insurance coverage is expected to expand.
Additional Resources
"Voice as a Biomarker of Health: Using Advanced Speech Analysis for Early Detection of Neurological and Psychiatric Conditions" - Journal of Medical Internet Research (2023)
"Emerging Applications of Voice Analysis in Clinical Practice" - American Medical Association Digital Health Report (2024)
"The Voice as a Window to Health: Ethical Frameworks for Speech Analysis in Clinical Settings" - Hastings Center for Bioethics (2023)
"Mayo Clinic Voice Biomarker Study: Longitudinal Analysis of Speech Changes in Chronic Disease Management" - Mayo Clinic Proceedings (2024)
"Voice Technology in Healthcare: A Comprehensive Review" - World Health Organization Digital Health Initiative (2023)