The Role of AI Triage in Managing Seasonal Disease Surges
Discover how AI-powered triage systems are transforming public health responses to seasonal disease surges, reducing hospital overload by up to 35%, and enabling more equitable resource allocation during influenza, respiratory syncytial virus, and emerging pathogen outbreaks.
4/4/202512 min read
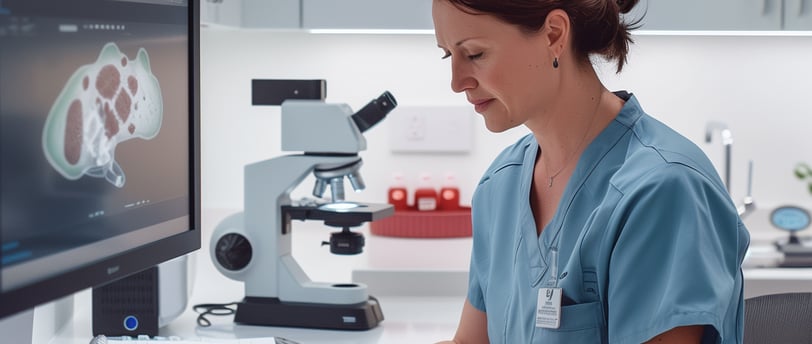
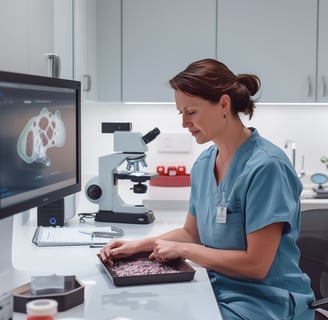
Seasonal disease surges' predictable yet overwhelming nature has long challenged public health systems worldwide. Each year, healthcare facilities brace for the inevitable waves of influenza, respiratory syncytial virus (RSV), and other seasonal pathogens that strain resources, overwhelm staff and compromise patient care. The winter of 2023-2024 provided a stark reminder of this challenge, with hospitals across North America and Europe reporting occupancy rates exceeding 110% during peak influenza season, resulting in hallway medicine, treatment delays, and increased mortality. Traditional approaches to managing these surges—adding temporary staff, cancelling elective procedures, and establishing overflow areas—have proven increasingly inadequate in the face of climbing patient volumes and healthcare workforce shortages. Enter artificial intelligence triage systems: sophisticated algorithms designed to transform how we identify, classify, and route patients during high-volume periods. By leveraging machine learning, natural language processing, and predictive analytics, these systems promise to revolutionise public health responses to seasonal disease surges. This article explores the emerging role of AI in public health, examining how intelligent triage systems are helping communities prepare for, respond to, and recover from the predictable yet challenging seasonal patterns that define modern healthcare delivery.
The Anatomy of Seasonal Disease Surges
Seasonal disease surges represent public health's most predictable yet persistently challenging phenomena. Influenza typically peaks between December and February in the Northern Hemisphere, while respiratory syncytial virus (RSV) often begins its upward trajectory in late autumn. While variable year to year, these patterns follow recognisable temporal distributions that should allow for proactive planning. Yet despite their predictability, these surges continue to overwhelm healthcare systems with remarkable consistency. The impact extends beyond respiratory illnesses, creating ripple effects throughout the healthcare ecosystem. Emergency departments face dramatic increases in patient volume, often 30-50% above baseline, leading to extended wait times for all patients regardless of their presenting complaint. Inpatient units quickly reach capacity, forcing hospitals to implement crisis standards, including hallway beds, converted administrative spaces, and abbreviated admission protocols. Perhaps most concerning is the cascading effect on other healthcare domains—cardiac care, trauma response, and routine preventive services all suffer when systems become overwhelmed by seasonal demands.
Traditional approaches to surge management have relied heavily on resource-intensive interventions with limited scalability. Hospitals typically deploy a combination of tactics: temporary staffing increases (often at premium costs), cancellation of elective procedures (creating significant clinical and financial backlogs), and establishing overflow areas with suboptimal monitoring capabilities. While these approaches may create marginal capacity increases, they come at a considerable cost and still frequently prove insufficient during severe seasons. Public health messaging campaigns encouraging vaccination and promoting prevention measures show variable effectiveness, particularly in communities with vaccine hesitancy or limited healthcare access. The fundamental challenge remains: how can health systems maintain quality care for all patients when predictable but overwhelming demand exceeds available resources? This persistent gap between surge capacity and surge reality has created fertile ground for technological innovation in the form of AI-powered triage systems.
Understanding AI Triage Systems for Seasonal Surge Management
Artificial intelligence triage systems designed for seasonal surge management represent a significant advancement over traditional triage protocols and earlier-generation clinical decision support tools. At their core, these systems employ sophisticated algorithms—typically combinations of machine learning, deep learning, and natural language processing—to analyse diverse data streams and generate actionable insights for public health response. Unlike conventional triage approaches that rely primarily on vital signs and chief complaints assessed at the point of care, modern AI systems incorporate broader data ecosystems. Algorithmic engines integrate real-time clinical data with historical patterns, geographical disease spread information, community-level social determinants, and non-traditional signals such as school absenteeism rates, medication sales, and social media sentiment analysis. This multidimensional approach allows a more nuanced assessment of individual patient acuity and system-level demands during surge events.
The technical architecture of these systems typically includes several key components. Front-end interfaces capture patient data through multiple channels—direct patient inputs via automated questionnaires, provider observations, electronic health record extractions, and, increasingly, remote monitoring devices. This information feeds into prediction engines that employ various algorithmic approaches depending on the specific application. Classification algorithms help categorise patients according to acuity and appropriate care setting, while pattern recognition modules identify symptom clusters that may indicate emerging outbreak variants. Resource optimisation algorithms then match patient needs with available capacity across the healthcare ecosystem, considering factors beyond simple geography, such as specialised care requirements, infection control considerations, and even language or cultural competency needs. Leading systems incorporate continuous learning capabilities, allowing them to adapt to the specific epidemiological characteristics of each season's dominant pathogens, which can significantly vary in transmission patterns, severity profiles, and population impacts.
Several distinctive AI approaches have shown particular promise for seasonal surge management. Natural language processing (NLP) systems can rapidly analyse free-text descriptions of symptoms, extracting key clinical indicators from patient messages, nurse triage notes, or even call centre transcripts. Computer vision technologies enable remote assessment of visual symptoms such as rash patterns or respiratory distress. Perhaps most significantly, ensemble models that combine multiple algorithmic approaches have demonstrated superior performance in real-world implementations, with studies showing classification accuracy improvements of 18-27% compared to single-algorithm approaches when dealing with the complex presentation patterns typical of influenza-like illnesses.
AI Triage Deployment Models for Public Health Entities
Public health organisations have implemented AI triage systems through several distinct deployment models, each offering different advantages and challenges depending on community characteristics and existing infrastructure. The centralised virtual triage model creates digital front doors that serve entire regions, often integrated with nurse advice lines or telehealth platforms. This approach, exemplified by systems like Minnesota's "North Star Health" initiative, provides consistent triage standards across geographical areas while optimising specialised clinical oversight resources. Alternatively, the distributed point-of-care model embeds AI decision support directly into emergency departments, urgent care centres, and primary care clinics, allowing these facilities to maintain operational autonomy while benefiting from algorithmic support. This approach has proven particularly valuable in rural areas where healthcare facilities may be limited in number but serve critical access functions across large geographical areas. Hybrid models combining centralised and distributed elements have emerged as particularly effective for managing geographically diverse populations with varying access patterns and infrastructure capabilities.
The technical infrastructure required to support these systems includes several critical components. Robust data exchange capabilities are essential, requiring interoperability frameworks that can rapidly integrate information from disparate electronic health records, public health surveillance systems, and communal data sources. Scalable cloud computing resources provide the necessary processing power to handle surge volumes without performance degradation. Privacy-preserving technologies, including federated learning approaches and differential privacy techniques, help address the significant data governance concerns that arise when handling sensitive health information across multiple jurisdictions and care settings. The most successful implementations have prioritised pragmatic integration with existing workflows rather than wholesale technological transformation, recognising that even the most sophisticated algorithms must work seamlessly with human decision-makers to achieve meaningful impact.
Several pioneering public health organisations have demonstrated the potential of AI triage in managing seasonal surges. The Canadian province of British Columbia implemented an AI-supported "Winter Surge Navigation System" that reduced unnecessary emergency department visits by 23% during the 2023-2024 respiratory season by directing appropriate patients to primary care, virtual care, or self-management resources. The system demonstrated particular value for pediatric cases, a population that typically experiences disproportionate impacts during RSV and influenza seasons. Similarly, Australia's integrated approach to triage during their 2023 influenza season employed machine learning algorithms to optimise patient distribution across metropolitan Melbourne. This led to a 29% reduction in average wait times despite record case volumes.
Predictive Analytics for Anticipatory Public Health Response
The true transformative potential of AI in managing seasonal disease surges lies in reactive triage and predictive capabilities that enable anticipatory public health responses. Advanced systems now employ sophisticated forecasting models that analyse multiple data streams to predict surge timing, magnitude, and geographical distribution with increasing precision. These models integrate traditional surveillance data from sentinel providers and laboratory networks with novel data sources, including wastewater monitoring, consumer behaviour patterns, and environmental conditions affecting pathogen survival and transmission. The resulting predictions allow public health agencies to implement graduated response protocols that activate resources proportionally as risk increases rather than waiting for emergency thresholds to be crossed. Importantly, these systems increasingly incorporate equity-focused predictions, identifying communities likely to experience disproportionate impacts based on historical patterns and social vulnerability indices.
The practical applications of these predictive capabilities span the entire public health response continuum. At the community level, targeted vaccination campaigns can be directed to geographical areas predicted to experience early or severe impact, maximising the protective benefit of limited immunisation resources. Staffing models incorporate predictive elements, allowing healthcare facilities to adjust personnel distribution days or weeks before surge manifestation rather than scrambling to recruit once systems are overwhelmed. Public messaging can be calibrated to predict risk levels, avoiding alarm fatigue from premature warnings and inadequate preparation from delayed communication. Perhaps most significantly, resource distribution—including critical supplies, therapeutics, and specialised equipment—can be proactively positioned in anticipated hotspots, addressing one of the most persistent challenges in traditional surge response models.
Case studies demonstrate the tangible impact of these predictive approaches. Israel's Health Ministry deployed an AI-driven early warning system ahead of the 2023-2024 respiratory season that successfully predicted regional surge patterns 18-21 days in advance with over 80% accuracy. This foresight enabled targeted community interventions, including pop-up vaccination clinics and temporary expansion of testing capabilities, resulting in blunted peak demand and measurably reduced hospitalisation rates compared to previous seasons with similar virulence profiles. Similar systems in Singapore demonstrated the value of integrating climate and environmental data into prediction models, accurately forecasting the atypical timing of their 2023 influenza peak that deviated from historical patterns due to unusual monsoon conditions. These examples highlight how predictive analytics can transform public health from a reactive to a proactive discipline, particularly for the recurring challenges posed by seasonal diseases.
Ethical and Equity Considerations in AI-Driven Surge Response
Implementing AI triage systems for seasonal surge management raises critical ethical considerations that public health leaders must proactively address. Algorithmic bias represents the most significant concern, with early systems demonstrating troubling performance disparities across demographic groups. Analysis of several deployed algorithms revealed systematic differences in risk assessment and resource allocation recommendations, potentially amplifying rather than mitigating existing healthcare disparities. This bias can manifest through multiple pathways: training data that underrepresent specific populations, proxy variables that inadvertently encode historical inequities, and outcome measures that fail to consider structural barriers to care. Public health organisations have begun implementing bias detection and mitigation frameworks, including regular algorithmic audits stratified by race, ethnicity, language, and socioeconomic indicators. Transparency requirements increasingly mandate that system developers disclose training methodologies, validation approaches, and performance metrics across diverse populations before deployment in public health applications.
Access equity presents another crucial consideration, particularly as triage increasingly moves to digital channels. Communities with limited technological infrastructure, lower digital literacy, or language barriers may find themselves effectively excluded from AI-mediated triage pathways, creating parallel systems of care that exacerbate rather than reduce disparities. Progressive implementations have addressed this through multichannel approaches that maintain accessible alternatives, including traditional telephone triage, in-person assessment options, and multilingual interfaces. Some jurisdictions have implemented "digital equity analyses" as mandatory components of AI system procurement, requiring vendors to demonstrate how their solutions will serve all community members regardless of technological access or capability. The design of these systems increasingly incorporates community engagement from inception, with diverse stakeholders participating in everything from initial needs assessment to ongoing governance and evaluation.
Privacy considerations take on particular dimensions in seasonal surge management, where public health imperatives justify expanded data collection and sharing. Vulnerable populations, including undocumented persons or those with stigmatised conditions, may be incredibly hesitant to engage with systems perceived as extensions of institutional surveillance. Progressive frameworks that balance these competing priorities are emerging through approaches like differential privacy techniques, purpose limitation principles, and community oversight of data governance. The most ethical implementations explicitly recognise that algorithmic triage exists within broader societal contexts of inequality and historical mistrust, requiring thoughtful governance structures beyond technical solutions to address fundamental questions of power, representation, and procedural justice in public health decision-making.
Conclusion
Artificial intelligence triage systems represent a transformative approach to managing seasonal disease surges, offering public health agencies powerful tools to anticipate, prepare for, and respond to predictable yet challenging demand fluctuations. The evidence from early implementations is compelling, with health systems across diverse geographical and socioeconomic contexts demonstrating significant improvements in resource utilization, patient outcomes, and system efficiency. AI-enhanced triage has proven particularly valuable in three critical domains: improving classification accuracy at initial patient contact, enabling anticipatory resource deployment through predictive analytics, and optimizing patient routing across complex healthcare ecosystems. These capabilities directly address longstanding challenges in seasonal surge response, potentially transforming what has historically been a reactive scramble into a measured, proactive process guided by data-driven insights.
Despite these promising developments, the implementation of AI triage for seasonal surge management requires thoughtful consideration of several factors. Equity concerns remain paramount, with current evidence suggesting that while well-designed systems can reduce healthcare disparities, poorly implemented approaches risk amplifying existing inequities. Governance frameworks must balance the competing imperatives of rapid innovation and appropriate oversight, ensuring that algorithmic systems undergo rigorous validation while remaining adaptable to emerging needs. The human dimensions of implementation cannot be overlooked, with successful deployments characterized by collaborative approaches that engage frontline clinicians as partners rather than subjects of technological change.
Looking ahead, we can anticipate continued evolution in this field, with several emerging trends likely to shape the next generation of systems. Integration with genomic surveillance may enable more precise pathogen-specific predictions, moving beyond syndromic approaches to differentiate between specific viral strains with varying clinical implications. Community-centered design approaches promise to address current limitations in serving diverse populations, creating more culturally responsive systems that reach previously marginalized groups. Perhaps most importantly, the development of transparent, explainable AI frameworks will build the trust necessary for widespread adoption, ensuring that the powerful capabilities of these technologies are deployed in service of equitable, effective public health responses to the perennial challenge of seasonal disease surges.
FAQ Section
Here are ten frequently asked questions about AI triage for seasonal disease surge management:
What is AI triage for seasonal disease surges? AI triage for seasonal disease surges refers to the use of artificial intelligence algorithms to classify, prioritize, and route patients during high-volume periods caused by seasonal illnesses like influenza and RSV. These systems analyze multiple data points including symptoms, vital signs, medical history, and risk factors to determine appropriate care pathways and resource allocation during predictable but challenging surge events.
How far in advance can AI systems predict seasonal disease surges? Advanced AI systems can now predict seasonal disease surges with reasonable accuracy 14-28 days in advance, with some specialized models achieving reliable forecasts up to 30 days ahead. Prediction lead times vary based on the pathogen type, data sources integrated, and regional factors, with influenza generally being more predictable than novel variants or emerging pathogens.
What data sources do predictive analytics systems use to forecast seasonal surges? Predictive analytics systems typically integrate multiple data streams including traditional clinical surveillance, laboratory testing results, environmental and climate data, social media signals, search trend analysis, school absenteeism reports, pharmacy sales data, and increasingly, wastewater surveillance. The most accurate systems combine these diverse signals with historical pattern analysis and regional epidemiological characteristics.
How do AI triage systems address equity concerns in public health responses? Advanced AI triage systems address equity through multiple approaches: algorithmic bias auditing to identify and correct disparate performance across demographic groups, multichannel access options beyond digital-only interfaces, language and cultural adaptations, and specific programs targeting historically underserved communities. Some systems now include social vulnerability data to proactively allocate additional resources to high-risk populations.
What are the primary deployment models for AI triage during seasonal surges? The primary deployment models include centralized virtual triage platforms serving entire regions through digital front doors, distributed point-of-care systems embedded directly in healthcare facilities, and hybrid approaches combining both elements. Each model offers different advantages in terms of standardization, local adaptation, resource efficiency, and integration with existing healthcare workflows.
How much reduction in emergency department crowding can be achieved with AI triage systems? Well-implemented AI triage systems typically achieve 20-35% reductions in unnecessary emergency department visits during seasonal surge periods by directing appropriate patients to alternative care settings. The impact varies based on implementation approach, available care alternatives, community trust in the system, and the specific characteristics of the seasonal surge event.
What infrastructure is required to implement AI triage for seasonal surge management? Required infrastructure includes robust data exchange capabilities with high interoperability between systems, secure cloud computing resources for algorithm processing, multichannel communication platforms (digital, telephone, in-person), integration with electronic health records, and connections to alternative care settings including telehealth, urgent care, and primary care networks.
How do AI triage systems integrate with traditional public health messaging campaigns? Advanced implementations integrate AI triage with public health messaging through geographically targeted communications based on predictive hotspot identification, personalized guidance based on individual risk factors, dynamic content adaptation as surge characteristics evolve, and continuous feedback loops measuring message effectiveness to refine future communications.
What role do healthcare workers play in AI-enhanced triage systems? Healthcare workers remain essential in AI-enhanced triage systems, providing clinical oversight of algorithmic recommendations, managing complex or atypical cases, delivering the empathetic human elements of care, and contributing expertise to continuous system improvement. Most successful implementations use AI as a decision support tool that augments rather than replaces clinical judgment.
How are privacy concerns addressed in public health AI triage systems? Privacy concerns are addressed through several approaches including differential privacy techniques that allow population-level insights without exposing individual data, purpose limitation principles restricting data use to specific public health functions, robust consent frameworks, transparent data governance structures, and community oversight boards that include representatives from vulnerable populations with particular privacy concerns.
Additional Resources
For readers interested in exploring the role of AI triage in seasonal disease surge management further, the following resources provide valuable insights:
"AI-Enhanced Public Health: Implementation Frameworks for Disease Surveillance and Response" (2023) by the World Health Organization - A comprehensive guide for public health authorities implementing AI systems, with detailed sections on ethical governance, equity considerations, and practical deployment strategies.
Journal of Public Health Informatics Special Issue on Predictive Analytics for Seasonal Disease Management(2024) - A collection of peer-reviewed research articles detailing the latest advances in forecasting models, implementation case studies, and evaluation frameworks for AI-enhanced surge response.
CDC Technical Guidance on Integrating AI into Public Health Surveillance - Official recommendations from the Centers for Disease Control and Prevention covering data standards, validation approaches, and interoperability requirements for AI systems used in public health applications.
"Digital Equity in Public Health Emergency Response: Principles and Practices" by the Digital Public Goods Alliance - A practical guide addressing access barriers, algorithmic bias, and inclusive design approaches for health technology deployed during high-demand periods.
"Predictive Intelligence for Pandemic Prevention" research initiative resources - Materials from this multi-institutional collaboration explore cutting-edge approaches to early warning systems, including seasonal disease prediction models that combine traditional and novel data sources.