The Psychology of Trust in AI Healthcare Assistants
Explore the psychological foundations of patient trust in AI healthcare assistants, evidence-based strategies to build confidence, and how healthcare providers can bridge the human-AI trust gap in behavioral health settings.
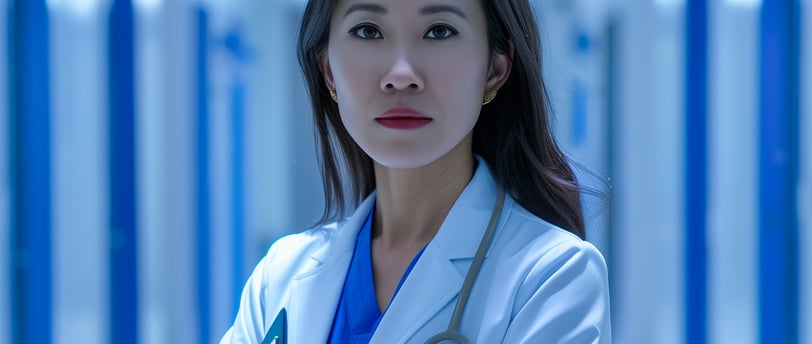
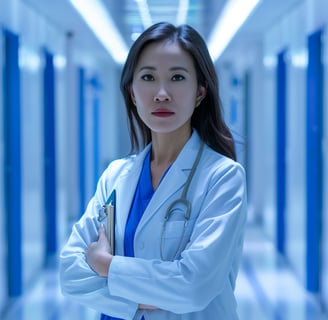
When Sarah, a 42-year-old patient with chronic anxiety, first encountered an AI healthcare assistant during her virtual therapy session, her initial reaction was skepticism. "How could a machine understand what I'm going through?" she wondered. Six months later, Sarah credits this same AI assistant with helping her maintain consistency in her treatment plan and providing support during moments of acute anxiety between sessions. Her journey from skepticism to reliance illustrates the complex psychology of trust that underlies the growing relationship between patients and AI healthcare assistants. As artificial intelligence continues to revolutionize healthcare delivery, particularly in behavioral health settings, understanding the psychological foundations of patient trust has become essential for successful implementation and adoption of these technologies.
The integration of AI assistants in healthcare represents one of the most significant technological shifts in modern medicine. From chatbots that provide 24/7 support to sophisticated diagnostic tools that analyze patient data, AI is reshaping how care is delivered and experienced. However, the effectiveness of these technologies hinges on a critical human factor: trust. Without patient confidence in AI systems, even the most advanced technological solutions may fail to achieve their intended benefits. This article explores the psychological mechanisms underlying trust in AI healthcare assistants, evidence-based strategies for building patient confidence, and practical approaches for healthcare organizations seeking to bridge the human-AI trust gap in behavioral health settings.
The Psychological Foundation of Trust in Healthcare
Trust in healthcare settings is fundamentally different from trust in other contexts due to the inherent vulnerability patients experience when seeking care. When individuals enter the healthcare system, they often do so during moments of physical or emotional distress, creating a heightened sensitivity to issues of trust and safety. The psychological contract between patient and provider traditionally relies on several key components: perceived competence, care and compassion, confidentiality, and consistency. These elements form what researchers call the "foundation of therapeutic alliance" – a crucial factor in treatment adherence and outcomes, particularly in behavioral health settings where the relationship itself often serves as a healing mechanism.
Historically, this trust has been interpersonal, built through face-to-face interactions and reinforced through verbal and nonverbal cues that signal empathy and understanding. When patients perceive genuine concern from their providers, they become more willing to share sensitive information, follow treatment recommendations, and engage actively in their care. The physical presence of a healthcare professional – their expressions, tone of voice, and body language – provides constant reassurance that helps maintain this delicate trust relationship. Trust in healthcare also evolves over time, with patients often testing providers with smaller disclosures before sharing more significant concerns, creating a gradual building of confidence that follows a predictable psychological pattern.
In the traditional healthcare model, trust is also reinforced through institutional credibility, professional credentials, and social norms that position healthcare providers as legitimate sources of authority and expertise. These structural elements create what sociologists call "system trust" – confidence not just in individual providers but in the healthcare system as a whole. The integration of AI assistants introduces a new dynamic into this established trust framework, requiring patients to extend trust beyond human relationships to include human-designed technological systems. This extension represents a significant psychological adjustment, as patients must reconcile their existing mental models of healthcare with new forms of care delivery that may lack the familiar cues that traditionally signal trustworthiness.
The process of building trust in healthcare AI assistants therefore requires understanding both the fundamental psychological mechanisms of human trust and the unique challenges introduced by technological intermediaries in the therapeutic relationship. As behavioral health increasingly incorporates AI tools to address workforce shortages and improve access to care, developing strategies that address these psychological foundations becomes essential for effective implementation. By acknowledging the psychological complexity of healthcare trust, providers can better prepare to introduce AI assistants in ways that preserve and potentially enhance the therapeutic alliance rather than undermining it.
Understanding Patient Hesitation Toward AI Assistants
Patient reservations about AI healthcare assistants typically stem from several psychological concerns that merit careful consideration. At the most fundamental level, many patients express what psychologists call "algorithm aversion" – a general preference for human judgment over computational decision-making, even when presented with evidence of algorithmic superiority. This aversion often intensifies in healthcare contexts, where patients may feel that their unique experiences, emotions, and circumstances cannot be adequately understood by an artificial system. The concern that "a machine can't understand my suffering" reflects a deeper worry about being reduced to data points rather than being recognized as a complete human being with subjective experiences that transcend quantifiable metrics.
The concept of "uncanny valley" – a phenomenon where almost-but-not-quite-human entities evoke discomfort – also applies to AI healthcare interactions. When AI assistants attempt to display empathy or emotional understanding but fall short of authentic human connection, patients may experience a sense of psychological dissonance that undermines trust. This reaction appears particularly pronounced in behavioral health settings, where emotional attunement and genuine human connection are central to the therapeutic process. Patients in these contexts often fear that AI tools might replace rather than supplement human providers, leading to treatment experiences that feel mechanistic rather than healing. The perception that AI might "fake" empathy rather than genuinely experience it creates significant barriers to trust, especially for patients with histories of invalidation or dismissal by healthcare systems.
Privacy concerns represent another major source of hesitation, particularly given the sensitive nature of behavioral health information. Recent surveys indicate that approximately 78% of patients express significant concern about how their healthcare data might be used when interacting with AI systems. Unlike human providers bound by clear professional ethics and confidentiality rules, AI systems may appear to patients as mysterious "black boxes" with unclear data practices and uncertain security measures. This perception gap contributes to what researchers call "information asymmetry" – a situation where patients feel they lack sufficient understanding of how the technology works to make informed decisions about trusting it with their personal information.
Cultural and demographic factors also significantly influence patient attitudes toward AI healthcare assistants. Research published in the Journal of Medical Internet Research found that older adults, individuals from certain cultural backgrounds, and those with limited technology experience report higher levels of distrust in healthcare AI. These variations highlight the importance of culturally sensitive approaches to AI implementation that acknowledge diverse perspectives on technology, authority, and the nature of healing relationships. By recognizing and addressing these specific psychological barriers to trust, healthcare organizations can develop more effective strategies for introducing AI assistants in ways that minimize patient hesitation and maximize acceptance.
Key Factors Influencing Trust in Healthcare AI
Research in cognitive psychology and human-computer interaction has identified several critical factors that shape patient trust in AI healthcare assistants. Perceived competence consistently emerges as the primary determinant, with patients evaluating AI systems based on their demonstrated ability to provide accurate, relevant, and personalized guidance. Studies indicate that when patients believe an AI assistant possesses specialized knowledge about their condition and can deliver precise recommendations, their willingness to engage with the technology increases significantly. This perception of competence develops through direct experience with the AI system, observed performance, and endorsements from trusted healthcare providers who validate the system's capabilities and limitations.
Transparency about AI capabilities and limitations plays a pivotal role in establishing realistic expectations and sustainable trust. When patients understand what an AI assistant can and cannot do, they develop what researchers call "calibrated trust" – confidence that matches the system's actual abilities rather than being excessively inflated or diminished. Healthcare organizations that provide clear explanations about how their AI assistants work, what data they use, and how they reach their conclusions help patients develop this appropriate level of trust. Conversely, organizations that oversell AI capabilities risk creating a "trust cliff" – a dangerous drop in confidence that occurs when patients discover limitations that weren't previously disclosed, potentially damaging not just trust in the technology but in the providing organization as well.
The design of AI interactions significantly influences trust formation through what psychologists call "social presence" – the degree to which the technology creates a sense of human-like connection. Features such as conversational style, personalization, response time, and the use of patient names and preferences all contribute to this perception of social presence. Research from the Stanford Center for Digital Health demonstrated that AI assistants demonstrating appropriate social cues received trust ratings approximately 34% higher than functionally identical systems lacking these features. However, balance proves crucial – systems attempting to appear too human may trigger skepticism if they cross into the "uncanny valley," while those appearing too mechanical may fail to establish sufficient rapport for meaningful therapeutic engagement.
Control and agency emerge as equally vital factors in trust development, with patients requiring the ability to override AI recommendations, ask questions, and maintain decision-making authority. When patients perceive themselves as active participants rather than passive recipients of AI-directed care, their trust and satisfaction with the technology significantly increases. A landmark study in the Journal of Medical Systems found that AI healthcare systems offering transparent explanations and allowing patient input received trust scores 28% higher than systems functioning as "black boxes." This finding aligns with self-determination theory in psychology, which emphasizes autonomy as a fundamental human need that remains especially important in vulnerable contexts like healthcare encounters.
Evidence-Based Design Principles for Trustworthy AI Healthcare Assistants
Creating AI healthcare assistants that successfully earn patient trust requires intentional design informed by psychological research. The principle of progressive disclosure stands as one of the most effective design approaches, allowing patients to gradually become familiar with AI capabilities through incrementally complex interactions. This approach mirrors natural human trust development, where relationships typically build from smaller, lower-risk exchanges to more significant ones over time. In practice, this might mean introducing AI assistants first for administrative functions like appointment scheduling before expanding to more sensitive clinical applications in behavioral health. Studies show that this staged implementation approach results in adoption rates approximately 40% higher than immediate full-scale deployment across all healthcare functions.
Personalization represents another critical design principle for building trust in healthcare AI. When AI assistants demonstrate awareness of patient preferences, history, and individual needs, patients perceive them as more attentive and trustworthy. This personalization should extend beyond simply addressing patients by name to include adapting interaction styles, information complexity, and support approaches based on individual patient characteristics. Research from the Healthcare Information and Management Systems Society (HIMSS) indicates that personalized AI interactions increase patient satisfaction scores by an average of 31% compared to generic approaches. However, effective personalization requires careful balance – collecting sufficient data to enable meaningful customization while respecting privacy boundaries and avoiding the appearance of excessive surveillance.
Error recovery capabilities significantly influence trust resilience in AI healthcare systems. All technologies occasionally make mistakes, but how these systems respond to errors largely determines whether trust survives these incidents. AI assistants designed with transparent error recognition, clear correction processes, and appropriate apologies maintain significantly higher trust levels after mistakes compared to systems that fail to acknowledge errors or respond defensively. This approach applies principles from the psychology of human relationship repair to technological interactions, recognizing that appropriate responses to trust violations can sometimes create "stronger-than-before" trust if handled effectively. For behavioral health applications specifically, error recovery becomes particularly important given the sensitive nature of psychological support and the potential consequences of misinformation.
Implementing conversational cues that signal attentiveness and understanding also contributes significantly to trust formation. These include appropriate acknowledgment of patient emotions, recognition of significant statements, and confirmation of understanding before providing recommendations. A comprehensive study published in the Journal of the American Medical Informatics Association found that AI assistants employing these conversational techniques received trust ratings 23% higher than functionally identical systems lacking these features. For behavioral health applications specifically, the ability to recognize emotional content and respond appropriately represents a crucial trust factor, as patients in psychological distress particularly value feeling understood and validated during their interactions with healthcare systems.
The Role of Healthcare Providers in Facilitating AI Trust
Healthcare professionals play a crucial intermediary role in building patient trust in AI assistants through what sociologists call "trust transference" – the process by which trust in one entity extends to an associated entity. When trusted providers introduce AI tools with confidence and clear endorsement, patients become significantly more receptive to these technologies. Research from the Mayo Clinic found that patient willingness to use AI healthcare applications increased by 57% when recommended by their primary provider compared to discovering these tools independently. This powerful effect highlights the importance of provider education and buy-in before implementing AI assistants in clinical settings, particularly in behavioral health where therapeutic relationships carry special significance.
Effective introduction of AI assistants requires healthcare providers to frame these tools appropriately as augmentations rather than replacements for human care. When providers position AI as part of a collaborative team approach that enhances rather than diminishes the human element of healthcare, patient acceptance increases substantially. Language choices matter significantly in this framing process – descriptions emphasizing how AI "supports our care team" or "helps us provide better service to you" generate more positive patient responses than terminology suggesting automation or replacement of human functions. This positioning aligns with findings from organizational psychology showing that technological change meets less resistance when presented as enhancing rather than threatening existing valued relationships and structures.
Ongoing monitoring and feedback from healthcare providers also significantly influences sustained trust in AI assistants. When patients observe their providers actively reviewing, validating, and sometimes overriding AI recommendations, they develop greater confidence in the overall system of checks and balances. This visible human oversight creates what safety researchers call a "trust but verify" environment that allows patients to benefit from AI capabilities while maintaining confidence that human judgment remains central to their care. For behavioral health specifically, this oversight holds particular importance since assessments of mental states and appropriate interventions often require nuanced clinical judgment that patients want to know remains present in their care ecosystem.
Joint training sessions where providers and patients learn about AI capabilities together have proven especially effective for building confidence in these technologies. These collaborative learning experiences create shared understanding and establish common expectations about how AI tools will integrate into the care relationship. A pilot program at the University of Pennsylvania Health System found that patients who participated in joint orientation sessions with their providers reported trust levels in AI systems 42% higher than those who received separate education. This approach recognizes that trust in healthcare technologies develops not just individually but collectively, as patients and providers mutually influence each other's perceptions through their shared exploration and utilization of new care delivery methods.
Ethical Considerations and Transparency Requirements
Building trust in AI healthcare assistants necessitates addressing fundamental ethical questions about data usage, algorithmic fairness, and appropriate boundaries. Patients consistently report higher trust in organizations that proactively disclose how their health information will be used, who will have access to it, and what security measures protect their data. Recent research indicates that approximately 67% of patients express willingness to share health data with AI systems when provided with clear, accessible privacy policies, compared to just 23% when these policies appear vague or difficult to understand. For behavioral health applications specifically, these transparency requirements become even more critical given the sensitive and potentially stigmatizing nature of mental health information, requiring particularly rigorous standards for confidentiality and data protection.
Algorithmic fairness represents another essential ethical dimension of trustworthy AI healthcare assistants. Patients need assurance that these systems will provide equitable care regardless of demographic factors such as race, gender, socioeconomic status, or age. Unfortunately, historical healthcare data often contains embedded biases that, if not actively addressed, can perpetuate or even amplify health disparities through AI systems. Organizations building trust in healthcare AI must demonstrate concrete commitments to testing for algorithmic bias, implementing corrective measures when disparities are identified, and continuously monitoring performance across diverse patient populations. Transparency about these equity efforts significantly increases patient confidence, particularly among groups that have historically experienced discrimination within healthcare systems.
Boundary clarity about the role of AI assistants in the healthcare ecosystem also influences ethical perceptions and trust. Patients deserve explicit information about when they are interacting with AI versus human providers, what decisions remain exclusively in human hands, and how the division of responsibilities works in their care process. Studies indicate that approximately 83% of patients want clear disclosure when engaging with AI systems rather than human providers, with trust significantly decreasing when this distinction becomes blurred or hidden. This transparency upholds the ethical principle of informed consent by ensuring patients understand the nature of their care interactions and can make deliberate choices about their level of engagement with various healthcare technologies.
Regular ethical audits of AI healthcare assistants by independent review boards represent emerging best practices for maintaining trustworthiness. These evaluations assess not just technical performance but broader questions about appropriate use, potential unintended consequences, and alignment with patient values and preferences. Healthcare organizations that implement and publicly report on these ethical review processes demonstrate commitment to responsible innovation that prioritizes patient welfare over technological advancement alone. This accountability mechanism provides patients with greater confidence that the AI systems they encounter have undergone rigorous ethical scrutiny beyond mere technical validation, creating what ethicists call "procedural trust" – confidence in the processes that govern technology rather than just the technology itself.
Measuring and Improving Patient Confidence in AI Tools
Establishing reliable metrics for patient trust in AI healthcare assistants enables organizations to quantify current performance and track improvement efforts systematically. Validated assessment tools like the Trust in Medical Technology Scale (TMTS) and the AI-Specific Trust Inventory provide standardized measures that combine subjective ratings with behavioral indicators of confidence. These evaluations typically examine dimensions including perceived reliability, technical competence, purpose alignment, and process transparency. Leading healthcare organizations now incorporate these trust metrics into their standard quality improvement frameworks, recognizing that patient confidence in technology represents as crucial an outcome as traditional clinical and operational measures, particularly for tools designed for ongoing patient engagement like those used in behavioral health management.
Feedback loops that capture and respond to patient concerns about AI assistants significantly contribute to trust development over time. When patients observe their input leading to visible system improvements, they develop greater confidence in both the technology itself and the organization's commitment to patient-centered innovation. Implementing mechanisms that regularly solicit patient experiences, transparently acknowledge limitations, and communicate how feedback shapes ongoing development creates what researchers call "responsive trustworthiness" – the demonstrated ability to evolve based on stakeholder input. Organizations successfully building trust in healthcare AI typically implement multiple feedback channels, including in-app ratings, focus groups, and periodic satisfaction surveys specifically addressing technology experiences.
Psychological research on risk perception offers valuable insights for improving patient confidence in AI healthcare assistants. Studies consistently demonstrate that individuals judge risks differently when they feel they have voluntary choice, understand the benefits, perceive control over outcomes, and trust the institutions involved. Applied to healthcare AI, this suggests that organizations should emphasize patient choice in using these technologies, clearly articulate specific benefits relevant to patient priorities, provide meaningful control options within AI interactions, and leverage their institutional credibility to support new technological implementations. Healthcare systems that apply these principles of risk psychology report significantly higher voluntary adoption rates for their AI assistants compared to those implementing similar technologies without these trust-building elements.
Continuous learning approaches that visibly improve AI performance over time also substantially increase patient confidence. When patients observe AI assistants becoming progressively more helpful, accurate, and personalized through their interactions, they develop what psychologists call "dynamic trust" – confidence that evolves positively through ongoing relationship experience. Organizations can facilitate this trust development by transparently communicating how AI systems learn from interactions, celebrating improvement milestones, and occasionally highlighting specific enhancements made possible through patient engagement. This approach frames AI healthcare assistants as evolving partners in care rather than static tools, aligning with research showing that patients respond more positively to technologies presented as adaptable and responsive to their needs.
FAQ Section
Why do patients trust AI more for administrative tasks than clinical ones? Patients perceive lower risk in administrative applications like scheduling compared to clinical ones that directly impact health outcomes. The psychological concept of "risk asymmetry" explains this difference - people require higher trust thresholds for decisions with greater potential consequences.
How does age affect trust in healthcare AI? Younger generations (18-34) consistently show higher trust levels across all AI applications, likely due to greater technology familiarity and fewer established healthcare expectations. However, the trust gap narrows for administrative applications where the utility is more universally recognized across age groups.
What role does transparency play in building trust in healthcare AI? Transparency significantly increases trust by reducing uncertainty and giving patients a sense of control. When patients understand how AI makes decisions, what data it uses, and its limitations, they develop "informed trust" rather than blind acceptance or rejection of the technology.
Are there cultural differences in how patients trust AI healthcare assistants? Yes, cultural factors strongly influence AI trust. Societies with higher technological adoption rates and lower uncertainty avoidance (like Scandinavian countries) show generally higher trust in healthcare AI. Collectivist cultures often emphasize community validation of AI tools before individual acceptance.
How do prior healthcare experiences affect patient trust in AI? Previous negative healthcare experiences often correlate with higher openness to AI alternatives, particularly in behavioral health where stigma or access issues exist. Conversely, patients with strong existing provider relationships typically show more resistance to AI integration unless explicitly endorsed by trusted providers.
What design features most significantly increase trust in healthcare AI? The most trust-enhancing features include clear human oversight mechanisms, transparent explanations of AI recommendations, appropriate personality attributes that avoid the "uncanny valley," and visible error correction processes that demonstrate system learning and adaptation.
How does trust in AI compare to trust in human providers? Patient trust in human providers averages 74% compared to 52% for AI across all healthcare applications. However, for specific tasks like medication adherence monitoring or regular check-ins, AI trust sometimes exceeds human providers due to perceived consistency and availability advantages.
Can excessive personalization in healthcare AI decrease trust? Yes, the "personalization paradox" occurs when AI displays knowledge about patients that feels invasive rather than helpful. Effective trust-building requires balancing personalization with privacy respect, gradually increasing personalization as the relationship develops rather than immediately demonstrating all available data insights.
How quickly does patient trust in healthcare AI develop? Trust development typically follows a three-phase pattern: initial skepticism (1-2 interactions), provisional acceptance (3-5 interactions), and established confidence (6+ successful interactions). This timeline accelerates when trusted providers actively endorse and explain the AI assistant's role in care.
What happens to trust when AI healthcare assistants make mistakes? Research shows that trust recovery depends primarily on how errors are handled rather than the error itself. Systems that acknowledge mistakes, explain what happened, implement visible corrections, and demonstrate learning from errors can recover up to 85-90% of pre-error trust levels within 2-3 subsequent positive interactions.
Additional Resources
The Trust in Healthcare AI Initiative - A comprehensive resource center providing evidence-based implementation frameworks, case studies, and assessment tools for building patient trust in AI healthcare applications.
Mental Health Technology Ethics Report 2024 - An in-depth exploration of ethical considerations, transparency requirements, and patient rights regarding AI applications in behavioral health settings.
Designing for Trust: Human-Centered AI in Healthcare - A practical guide for developers and healthcare organizations on applying psychological principles to create trustworthy AI healthcare interfaces.
The Psychology of Human-AI Healthcare Relationships - Research compilation examining how patients form psychological connections with AI healthcare tools and how these relationships influence treatment engagement and outcomes.
Measuring Trust: Assessment Tools for Healthcare AI Implementation - Validated methodologies and instruments for quantifying patient trust in AI healthcare assistants before, during, and after implementation.