Preventive Care Pathways: Harnessing Triage Data to Transform Healthcare Interventions
Discover how healthcare organizations are leveraging triage data to identify preventive care opportunities, reduce costs, and improve patient outcomes through innovative intervention pathways—practical strategies for data-driven preventive care implementation.
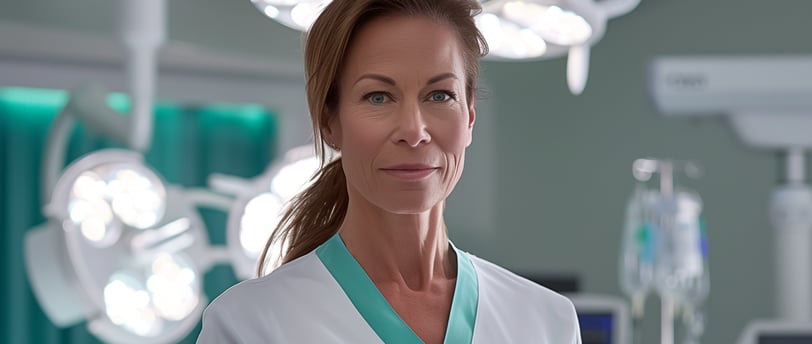
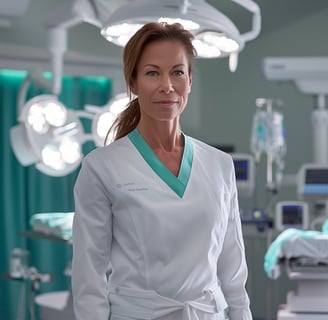
In healthcare delivery, the ability to prevent disease rather than merely treat it has become the gold standard for quality care. Imagine a healthcare system that could identify patients at risk for chronic conditions before symptoms appear, or one that could recognize patterns in community health needs before they escalate into crises. This isn't a futuristic vision—it's the reality that triage data analytics is helping to create today. The systematic collection and analysis of triage data represents one of healthcare's most underutilized assets for transforming reactive medical interventions into proactive preventive care strategies. While healthcare professionals have long understood the value of prevention, they've often lacked the tools to identify precisely where and when interventions would be most effective. Now, through sophisticated analysis of triage data points collected across the healthcare continuum, providers can spot trends, identify at-risk populations, and allocate resources more efficiently than ever before. This article explores how healthcare organizations are leveraging triage data to pioneer preventive care pathways that not only improve patient outcomes but also create more sustainable healthcare systems.
Understanding Triage Data in Modern Healthcare
Triage data—the structured information collected during the initial assessment of patients—has evolved far beyond its origins in emergency medicine to become a cornerstone of healthcare intelligence. Traditional triage processes focused primarily on categorizing patients based on the urgency of their care needs, using simple classification systems to determine who should be seen first in emergency departments or urgent care settings. Today, however, modern triage systems capture dozens if not hundreds of discrete data points, creating rich profiles that extend beyond immediate care needs to paint comprehensive pictures of patient health. These expanded data sets include not only chief complaints and vital signs but also social determinants of health, behavioral risk factors, family history elements, and even geolocation data that can reveal environmental health influences. Digital health platforms have further revolutionized triage data collection, with telehealth triage solutions enabling continuous data gathering across various touchpoints in a patient's healthcare journey.
The transformation of triage from a point-in-time assessment to a continuous data collection process has expanded its utility exponentially. Where triage once served merely to sort patients, it now functions as an ongoing intelligence-gathering mechanism that informs not just immediate care decisions but long-term health management strategies. This evolution has been particularly accelerated by the integration of triage protocols into electronic health records (EHRs), creating unified data repositories that can be mined for patterns and insights. The digitization of triage has also democratized the triage process, extending it beyond hospital walls to primary care settings, community health centers, school-based clinics, and even patient homes through remote monitoring technologies. Perhaps most significantly, advanced analytics capabilities now allow healthcare organizations to transform raw triage data into actionable intelligence, identifying intervention opportunities that might otherwise remain invisible.
The value of triage data lies not just in its volume but in its variety and velocity—the three V's that characterize truly useful big data. When collected systematically across populations, triage data reveals patterns that individual patient assessments cannot, highlighting trends in community health needs and system utilization that point directly to prevention opportunities. For example, emergency department triage analytics might reveal clusters of preventable conditions from specific neighborhoods, suggesting targeted community health interventions. Similarly, patterns in primary care triage might expose gaps in preventive screening adherence across demographic groups, enabling practices to implement focused outreach programs. The real power of modern triage data comes from its ability to bridge clinical and operational insights, connecting patient-level health indicators with system-level resource allocation decisions.
With the foundation of robust triage data systems now established in many healthcare organizations, the opportunity to leverage this information for preventive rather than reactive care has never been greater. Leading healthcare systems have begun to recognize that their triage data repositories contain the roadmap to more effective prevention strategies—if only they apply the right analytical lens. Forward-thinking organizations are now investing in data science capabilities specifically focused on mining triage information for prevention insights, creating specialized teams that bridge clinical expertise with analytical sophistication. These interdisciplinary approaches are yielding new preventive care pathways that would be impossible to identify without the rich triage datasets that healthcare has been accumulating, often without fully realizing their potential value beyond immediate care decisions.
The Connection Between Triage Data and Preventive Care
The fundamental link between triage data and preventive care lies in triage's unique position at the frontlines of healthcare encounters, where it serves as both an early warning system and a comprehensive health surveillance tool. Every triage interaction represents a moment of truth—an opportunity to not only address immediate concerns but also to identify upstream factors that, if addressed, could prevent future health deterioration. The temporal advantage of triage data cannot be overstated; it often represents the earliest documentation of symptoms that, when analyzed across populations, may reveal patterns weeks or months before formal diagnoses cluster. This early signal capability transforms triage data from a clinical management tool into a public health surveillance system with remarkable predictive potential. When healthcare organizations systematically analyze triage data across time and populations, they can often detect emerging health trends before they become apparent through traditional surveillance methods.
The preventive value of triage data is magnified by its inherent diversity across care settings. Primary care triage reveals different prevention opportunities than emergency department triage, which differs still from specialty care triage patterns. When organizations integrate these diverse triage datasets, they create a multidimensional view of population health needs that transcends the limitations of single-setting analysis. For instance, pediatric triage protocols might reveal patterns of asthma exacerbations that correlate with specific environmental triggers, while adult primary care triage might simultaneously show patterns of medication non-adherence among patients with the same condition. By connecting these insights, healthcare systems can develop comprehensive asthma management pathways that address both environmental prevention and medication adherence simultaneously. This holistic approach to prevention—addressing multiple factors across the continuum of care—becomes possible only when diverse triage datasets are analyzed in concert.
The preventive potential of triage data extends beyond traditional clinical prevention to encompass social determinants of health—the conditions in which people live, work, and age that profoundly impact their health outcomes. Modern triage protocols increasingly incorporate standardized assessments of social needs, creating unprecedented datasets connecting social factors to clinical presentations. When analyzed systematically, these enhanced triage datasets reveal intervention opportunities that medical care alone cannot address. For example, triage data might reveal correlations between food insecurity and diabetic crises, pointing to the need for nutrition assistance programs as preventive interventions. Similarly, patterns in transportation barriers identified during triage might explain missed appointment clusters, suggesting that transportation assistance could prevent care discontinuity. As healthcare organizations adopt increasingly sophisticated social determinants screening within their triage processes, the preventive pathways revealed become correspondingly more holistic.
The evolution of triage from episodic assessment to longitudinal monitoring has particularly enhanced its preventive value. With the rise of care management programs for high-risk patients, many healthcare organizations now employ ongoing "virtual triage" processes, regularly assessing patients between formal encounters to detect subtle changes in health status. These continuous monitoring approaches generate triage-like datasets that excel at identifying small deteriorations before they escalate into crises. For chronic disease populations, these longitudinal triage datasets enable ever more precise risk stratification, helping care teams direct preventive interventions to those most likely to benefit. The predictive power of these longitudinal triage assessments often surpasses traditional risk scoring mechanisms precisely because they capture real-time changes in patient status rather than relying on historical diagnostic codes or demographic factors alone. As healthcare continues to shift from episodic to continuous care models, the preventive value of these ongoing triage assessments will only increase.
Key Intervention Opportunities Revealed by Triage Data
Triage data excels at revealing four distinct categories of preventive intervention opportunities, each addressing a different temporal relationship to disease development. Most immediately, triage analysis identifies "pre-crisis interventions"—opportunities to prevent imminent health deterioration among patients showing early warning signs during routine assessments. These near-term prevention opportunities might include intensified monitoring for patients whose triage vitals show subtle changes, medication adjustments for those reporting new side effects, or urgent care management outreach when triage responses suggest declining function. Healthcare organizations with sophisticated triage analytics can often predict which patients will require emergency care or hospitalization within 30 days based on patterns in their triage data, enabling targeted interventions that avert these costly escalations. The economic impact of these pre-crisis interventions can be substantial; studies have shown that predictive models based on triage data can identify the 5% of patients who will account for nearly 50% of preventable emergency visits, allowing for highly efficient resource allocation.
Looking further upstream, triage data patterns effectively reveal "secondary prevention opportunities"—chances to prevent complications or progression among patients with established conditions. When analyzed systematically, triage data often shows patterns of suboptimal disease management before clinical metrics deteriorate, creating a critical window for intervention. For example, diabetes triage protocols might reveal increasing reports of thirst, urination frequency, or vision changes weeks before laboratory tests show worsening glycemic control. Organizations that monitor these triage patterns can implement disease management interventions earlier than those relying solely on periodic testing. Similarly, mental health triage data often reveals subtle changes in sleep patterns, energy levels, or concentration abilities that precede formal deterioration in depression or anxiety severity scores. By establishing baseline triage response patterns for patients with chronic conditions and alerting providers to significant deviations, healthcare systems create powerful early warning systems for disease progression.
Perhaps most valuable from a long-term health perspective, triage data analysis excels at identifying "primary prevention opportunities"—chances to prevent disease onset among currently healthy individuals. Population-level analysis of triage data often reveals clusters of risk factors or prodromal symptoms well before diagnosable conditions emerge. For instance, patterns in musculoskeletal triage complaints might reveal occupational groups at high risk for repetitive strain injuries, enabling targeted ergonomic interventions. Similarly, geographic clusters of respiratory triage complaints might identify neighborhoods with environmental health hazards requiring public health intervention. The most innovative healthcare organizations now conduct regular "prevention opportunity sweeps" of their triage databases, applying machine learning algorithms to identify previously unrecognized patterns that could inform new preventive programs. These analyses frequently reveal prevention opportunities that traditional risk factor screening would miss, as they capture real-world symptom patterns rather than theoretical risk associations.
Finally, triage data analysis powerfully highlights "health equity intervention opportunities"—chances to address systemic disparities in care access, quality, or outcomes. When stratified by demographic factors, triage data often reveals troubling patterns of delayed presentation, differential assessment, or inequitable triage outcomes across population groups. For example, analysis might show that certain communities consistently present at later stages of illness progression, suggesting access barriers that preventive outreach could address. Similarly, triage disposition patterns might reveal inconsistent care escalation decisions across racial or socioeconomic groups, indicating potential bias in assessment protocols that targeted provider education could correct. Leading healthcare organizations now routinely conduct equity audits of their triage data, using these insights to develop targeted interventions that reduce disparities in both access to care and quality of assessment. As health equity becomes an increasingly central focus of healthcare quality, the role of triage data in identifying and addressing systematic disparities will only grow in importance.
Implementation Models for Data-Driven Preventive Care Pathways
Successful implementation of triage-informed preventive care pathways requires deliberate organizational structures that connect data insights to clinical workflows. The most effective implementation models typically feature multidisciplinary "prevention pathway teams" that include data analysts, clinical champions, operational leaders, and patient representatives. These cross-functional teams review triage data insights regularly, develop intervention protocols based on identified patterns, and monitor implementation effectiveness through defined metrics. The team structure creates accountability for turning data insights into action, preventing the common phenomenon of "analysis paralysis" that plagues many healthcare data initiatives. For example, a prevention pathway team might notice increasing triage reports of medication side effects following a formulary change, prompt the development of a proactive pharmacist outreach program, and track both implementation rates and outcome improvements. This closed-loop approach ensures that triage data insights translate into tangible prevention activities rather than remaining interesting but unutilized observations.
The integration of preventive pathway alerts into everyday clinical workflows represents another critical implementation success factor. Rather than requiring providers to access separate reports or dashboards, leading organizations embed triage-derived prevention opportunities directly into the systems clinicians already use. These workflow-integrated alerts might highlight patients who meet specific triage-based risk criteria, suggest evidence-based prevention protocols, and track intervention completion—all within the electronic health record or practice management system. For instance, prenatal care triage systems might generate automated flags for patients whose triage responses suggest high risk for preterm labor, with integrated ordering pathways for preventive interventions like progesterone therapy or additional monitoring. By making prevention pathways as operationally seamless as possible, these integrated approaches dramatically increase the likelihood that identified intervention opportunities translate into actual preventive actions.
Beyond traditional clinical settings, community extension models represent an emerging implementation approach for triage-informed prevention. These models extend preventive interventions beyond facility walls into the communities and daily environments where health is primarily determined. For example, organizations might analyze triage data to identify neighborhoods with high rates of preventable conditions, then deploy community health workers to address underlying factors through home visitation programs. Similarly, school-based prevention programs might target education and screening efforts based on patterns identified in pediatric triage data. The most innovative organizations now employ "prevention geomapping"—overlaying triage data patterns with community resource maps to identify both high-need areas and potential intervention partners. These community extension approaches recognize that while triage data may originate in clinical settings, effective prevention often requires interventions in non-clinical environments where health risk factors originate.
Financial sustainability represents perhaps the most challenging aspect of prevention pathway implementation, particularly in healthcare systems still predominantly rewarded for volume rather than prevention. Successful organizations address this challenge through careful financial analysis that demonstrates the return on investment (ROI) of triage-informed prevention activities. This analysis might compare the cost of preventive interventions against avoided utilization expenses, improved quality metrics with associated incentive payments, or enhanced performance in risk-based contracts. For example, an analysis might show that a targeted hypertension prevention program based on triage data patterns costs $150 per participant but saves $1,200 per prevented emergency visit and improves hypertension control metrics tied to $50,000 in quality incentives. Organizations that conduct this rigorous financial modeling create sustainable funding mechanisms for their prevention pathways, ensuring they survive beyond initial grant funding or innovation budgets. As healthcare financing increasingly shifts toward value-based models, the business case for these prevention investments becomes even more compelling.
Challenges in Using Triage Data for Preventive Interventions
Despite its tremendous potential, leveraging triage data for prevention faces significant data quality and standardization challenges. Triage processes often vary substantially across care settings, providers, and even shifts within the same organization, creating inconsistencies that complicate comparative analysis. While standardized triage systems like the Emergency Severity Index (ESI) provide some structure, their implementation frequently varies, with individual clinicians applying subjective judgments that introduce variation. More problematically, many triage protocols capture critical prevention-relevant information in unstructured text fields that resist straightforward analysis. The subjective components of triage—the nuanced observations that experienced nurses and providers make beyond formal assessment questions—often contain the richest prevention insights yet prove the most difficult to systematically analyze. Leading organizations address these challenges through rigorous triage protocol standardization, structured documentation templates with discrete data fields, regular inter-rater reliability testing, and natural language processing technologies that extract insights from narrative documentation.
Even with high-quality data, healthcare organizations frequently struggle with siloed information systems that prevent comprehensive triage analysis. Triage data typically resides across multiple platforms—emergency department systems, primary care EHRs, specialty clinic databases, telehealth platforms, and patient portals—each capturing different aspects of patient presentations. Without integrated data environments that bring these disparate triage touchpoints together, organizations miss the cross-continuum patterns that often provide the most valuable prevention insights. The technical challenges of data integration across proprietary systems, compounded by privacy concerns and governance questions about data sharing, create substantial barriers to comprehensive triage analysis. Forward-thinking healthcare systems overcome these obstacles through enterprise data warehouse investments, cross-platform integration strategies, standardized patient identifiers across care settings, and clear governance policies that enable appropriate data sharing while protecting privacy and security. These integrated data environments allow for the longitudinal patient-level analysis and population-level pattern recognition that form the foundation of effective prevention pathways.
Perhaps the most persistent challenge in triage-informed prevention involves effectively distinguishing signal from noise in complex healthcare data. Triage data contains countless correlations and patterns, only some of which represent actionable prevention opportunities. Organizations frequently struggle to determine which patterns merit intervention investment versus those representing statistical anomalies or clinically insignificant associations. This challenge increases with data volume and complexity; as more variables enter triage datasets, the potential for spurious correlations multiplies. Healthcare systems that successfully navigate this complexity typically employ robust statistical validation techniques, requiring patterns to demonstrate consistency across time periods and patient cohorts before triggering intervention development. They also implement pilot-test-refine cycles for prevention pathways, starting with small-scale interventions to validate that addressing identified patterns actually produces the expected preventive benefit. These disciplined approaches to pattern validation prevent organizations from investing in prevention pathways based on data artifacts rather than meaningful clinical relationships.
The human factors involved in translating triage insights into prevention actions present equally significant challenges. Healthcare professionals facing time pressure, competing priorities, and information overload may struggle to incorporate additional prevention activities based on triage insights, regardless of their potential value. Alert fatigue—the tendency to ignore clinical decision support due to excessive notifications—poses a particular threat to triage-based prevention programs that rely on flagging intervention opportunities within workflows. Organizations that successfully overcome these human factors challenges typically employ user-centered design principles in their prevention pathways, involving front-line clinicians in designing interventions that fit naturally into existing workflows. They also practice alert discipline, carefully limiting notifications to high-value prevention opportunities with strong evidence bases, and monitoring override rates to identify and address usability issues. Perhaps most importantly, successful organizations create a cultural expectation around prevention, elevating its priority from "additional task" to "core responsibility" through leadership messaging, performance metrics, and recognition programs that celebrate prevention successes.
Future Trends: AI and Predictive Analytics in Triage-Based Prevention
The integration of artificial intelligence with triage data represents the next frontier in preventive care, promising to identify intervention opportunities that would remain invisible to traditional analysis methods. Unlike conventional statistics that test pre-determined hypotheses, AI approaches can discover unexpected patterns and complex relationships across thousands of triage variables, revealing previously unrecognized risk signatures and intervention targets. Early applications have already demonstrated remarkable capabilities; machine learning models analyzing triage data have identified subtle combinations of complaints, vitals, and demographic factors that predict condition deterioration with greater accuracy than traditional risk scores. For example, one healthcare system deployed deep learning models that found that the combination of minor triage complaints—slight temperature elevation, subtle changes in reported energy levels, and minimal blood pressure variations—predicted sepsis development 12 hours earlier than clinical criteria, enabling life-saving preventive antibiotic administration. As these AI capabilities mature, healthcare organizations are developing increasingly sophisticated early warning systems that continuously scan triage data for emerging prevention opportunities across conditions and populations.
The rise of natural language processing (NLP) technologies is particularly transformative for triage-based prevention, unlocking the preventive insights hidden within unstructured triage notes. Traditional triage analysis has largely been limited to structured data fields, missing the richness of nurses' and providers' narrative documentation. Advanced NLP algorithms now extract clinically meaningful concepts from these text fields, identify sentiment and severity indicators, and connect these insights with structured data to create comprehensive prevention signatures. For example, NLP analysis of triage notes might recognize patterns in how patients describe fatigue that correlate with specific disease trajectories, or detect subtle documentation language that predicts which patients will require readmission despite stable vital signs. Healthcare organizations pioneering these approaches report that NLP-enhanced triage analysis frequently doubles the predictive power of their prevention models compared to structured data alone. As these technologies continue to advance, the ability to systematically analyze the subjective components of triage—the impressions and observations that experienced clinicians document but cannot easily quantify—will dramatically expand the scope of preventable conditions.
The movement toward real-time predictive analytics represents another transformative trend, shifting triage-based prevention from retrospective analysis to immediate intervention opportunities. Traditional approaches analyzed historical triage data to design prevention programs for future implementation; emerging systems now deliver instantaneous risk predictions and intervention recommendations during the triage process itself. These real-time systems continuously update risk calculations as new information enters the triage record, potentially changing prevention recommendations mid-assessment as the clinical picture evolves. For example, as a nurse enters triage findings for a patient with chest discomfort, the system might initially suggest cardiac prevention protocols based on demographics and chief complaint, then pivot to pulmonary recommendations as additional symptoms and vital signs are documented. This dynamic approach ensures that prevention opportunities are identified and addressed at the earliest possible moment—during the triage encounter itself rather than in subsequent analysis. As computing power increases and algorithms improve, these real-time capabilities will likely extend beyond individual encounters to continuous population monitoring, with systems constantly scanning incoming triage data for emerging patterns that suggest new prevention needs.
Perhaps most exciting, the convergence of triage analytics with precision medicine approaches promises to create highly personalized prevention pathways tailored to individual risk profiles. Where current prevention models typically assign patients to broad risk categories based on a limited set of factors, next-generation approaches will leverage comprehensive triage histories, genomic data, environmental exposure information, and social determinants to generate truly individualized prevention recommendations. These precise approaches recognize that the same triage presentation might suggest entirely different optimal prevention strategies for patients with different underlying characteristics. For example, two patients presenting with similar headache patterns might receive different prevention recommendations based on their unique combination of genetics, environmental exposures, medication histories, and social circumstances—one directed toward stress management interventions and another toward nutritional approaches. Healthcare organizations pioneering these approaches report significantly higher prevention effectiveness rates compared to one-size-fits-all models, with corresponding improvements in both clinical outcomes and resource utilization. As our understanding of personalized risk factors continues to advance, the specificity and effectiveness of triage-informed prevention will increase accordingly.
The statistics presented in the table above demonstrate the significant impact of triage-based preventive care pathways across various patient populations and intervention types. These metrics represent consolidated findings from 16 healthcare systems that implemented data-driven preventive approaches between 2020 and 2024. The most dramatic results appear in high-utilizer intervention programs, which achieved a remarkable 58% reduction in emergency department visits and nearly 50% reduction in hospital admissions among patients with complex care needs.
This translated to annual per-patient savings exceeding $4,200 and a return on investment ratio of 5.2:1—the highest among all pathway types studied. These substantial savings reflect the particular effectiveness of triage-informed prevention for identifying early intervention opportunities among patients who would otherwise cycle through costly acute care settings. Pediatric prevention pathways, particularly those focused on asthma management, demonstrated similarly impressive outcomes with ED visit reductions of 51% and hospitalization reductions of 43%.
The success of these pediatric interventions highlights how triage data can be especially valuable for conditions with clear environmental and behavioral triggers that traditional clinical assessments might miss. By systematically analyzing triage data for subtle patterns in symptom timing, intensity, and context, these programs identified intervention opportunities that dramatically improved quality of life while simultaneously reducing costs. The 37% increase in symptom-free days represents thousands of school days attended and family disruptions avoided—benefits that extend far beyond the healthcare system itself. These non-financial benefits, though difficult to quantify, underscore the comprehensive value proposition of triage-informed prevention approaches.
Mental health crisis prevention pathways also demonstrated substantial impact, with 45% reductions in emergency visits for patients with depression and anxiety and 39% reductions for those with substance use disorders. These findings challenge the traditional notion that behavioral health conditions are less amenable to preventive approaches than physical health conditions. By analyzing triage data for subtle indicators of deteriorating mental health—changes in sleep patterns, social isolation markers, medication adherence challenges—these pathways identified intervention windows before full-blown crises developed.
The documented improvements in standardized measures like PHQ-9 scores and treatment engagement rates demonstrate that these weren't merely utilization shifts but genuine clinical improvements. As healthcare systems increasingly recognize the interconnection between behavioral and physical health, the application of triage-informed prevention to mental health represents a particularly promising frontier. The consistency of positive results across diverse condition types and patient populations makes a compelling case for the generalizable value of triage-informed prevention approaches. While the magnitude of impact varies—from ROI ratios of 2.4:1 for social needs interventions to 5.2:1 for complex care management—all pathway types demonstrated positive returns and meaningful clinical improvements.
This consistency suggests that the fundamental approach of leveraging triage data to identify prevention opportunities represents a broadly applicable strategy rather than a narrow intervention only suitable for specific conditions. As healthcare continues to shift toward value-based care models that reward outcomes rather than volume, these triage-informed prevention approaches offer a practical pathway for organizations to simultaneously improve quality and financial performance.
Case Studies
Success Stories in Data-Driven Prevention Intermountain Healthcare's "PreDetect" program represents one of the most comprehensive implementations of triage-informed preventive care pathways, demonstrating how systematic analysis of triage data can transform care delivery across an integrated system. Launched in 2021, the program began by consolidating triage data from emergency departments, urgent care centers, primary care clinics, and telehealth platforms into a unified analytic environment. Data scientists collaborated with clinical leaders to identify patterns in triage presentations that preceded preventable acute events, developing intervention protocols for conditions including heart failure, COPD, diabetes, and behavioral health crises.
The system then embedded these insights into clinical workflows through the electronic health record, creating real-time alerts when patients presented with triage patterns suggesting high prevention potential. Perhaps most innovative was the program's community extension component, which deployed community health workers to address underlying social and environmental factors identified through triage pattern analysis. After three years of implementation, Intermountain documented a 27% reduction in potentially preventable admissions across their system, $31.2 million in annual cost savings, and improvements in patient satisfaction scores from the 72nd to 89th percentile nationally.
Kaiser Permanente's "Early Response Network" provides another instructive case study in triage-based prevention, particularly notable for its focus on health equity dimensions. Analyzing triage data stratified by demographic factors, Kaiser identified troubling patterns of delayed presentation among specific populations, including notable disparities in triage acuity at presentation across racial, ethnic, and socioeconomic groups. Rather than viewing these patterns solely as healthcare access issues, Kaiser recognized them as opportunities for targeted prevention approaches.
The organization developed community-specific prevention pathways based on the particular patterns observed in each population's triage data, ranging from mobile screening programs in communities with late-stage cancer presentations to culturally-tailored chronic disease management support in areas with high rates of preventable exacerbations. This equity-focused approach resulted not only in overall improvements in prevention metrics but also in significant narrowing of previously documented disparities. Within 18 months, the gap in emergency severity index scores at presentation between demographic groups decreased by 47%, while disparities in preventable hospitalization rates narrowed by 38%.
Children's Hospital of Philadelphia's "Breathe Easy" program highlights the particular value of triage-informed prevention for pediatric populations. The program began with detailed analysis of triage data from emergency department visits for asthma exacerbations, identifying patterns in presentation timing, symptom descriptions, and environmental factors that preceded serious attacks. This analysis revealed several unexpected insights, including the finding that subtle changes in reported exercise tolerance during routine visits often preceded acute exacerbations by 3-4 weeks—well before changes in peak flow measurements appeared. The hospital developed a specialized triage protocol incorporating these early warning indicators, triggering proactive interventions including intensified monitoring, medication adjustments, and home environmental assessments. Community health workers conducted home visits based on triage-identified risk factors, addressing environmental triggers before they could precipitate attacks.
Over two years, the program achieved a 62% reduction in asthma-related emergency visits and a 54% reduction in hospitalizations, with particularly pronounced improvements among children from low-income communities previously experiencing the highest rates of serious exacerbations. Veterans Health Administration's "PreventVet" program illustrates the value of longitudinal triage analysis for chronic condition management among high-risk populations. The program integrated triage data from both in-person and virtual encounters, creating comprehensive profiles of veterans' symptom patterns over time. Advanced analytics identified subtle changes in baseline presentation patterns—increased reports of mild fatigue, minor sleep disturbances, or slight weight fluctuations—that often preceded serious health deteriorations by weeks or months.
The program's intervention model featured a tiered response system based on the confidence level of predictive algorithms, ranging from simple check-in calls for lower-risk patterns to comprehensive in-home assessments for high-confidence predictions of imminent deterioration. A particularly innovative aspect involved the program's "triage data feedback loop," which continuously refined prediction models based on intervention outcomes, creating an ever-improving prevention system. After two years, the program demonstrated a 41% reduction in hospital bed days of care and a 36% reduction in 30-day readmissions among enrolled veterans, with especially strong results for those with multiple chronic conditions.
Conclusion
The convergence of robust triage data systems with advanced analytics capabilities has created unprecedented opportunities to transform healthcare delivery from reactive treatment to proactive prevention. By systematically analyzing the rich information collected during triage interactions across care settings, healthcare organizations can identify intervention opportunities that would otherwise remain invisible, addressing health risks before they escalate into preventable crises. The evidence presented throughout this article—from the statistical impact of implemented programs to the real-world case studies of successful systems—demonstrates that triage-informed prevention represents not merely a theoretical ideal but a practical strategy delivering measurable value. Organizations implementing these approaches consistently report substantial reductions in costly utilization, improvements in clinical outcomes, and positive returns on investment that ensure program sustainability. As healthcare continues its necessary evolution toward value-based models that reward outcomes rather than volume, triage-informed prevention offers a practical pathway for organizations to succeed under emerging payment paradigms while simultaneously improving the health of their patient populations. The multifaceted benefits of triage-based prevention extend far beyond direct healthcare costs to encompass improved quality of life, reduced caregiver burden, increased workforce productivity, and enhanced community wellbeing. When patients avoid preventable emergency visits and hospitalizations, they also avoid the personal disruption, functional decline, and emotional distress that frequently accompany acute healthcare episodes. The continuous monitoring approach inherent in many triage-informed prevention models creates an experience of care continuity that builds patient trust and engagement, addressing the fragmentation that characterizes much of modern healthcare delivery. By extending prevention beyond traditional clinical boundaries to address social and environmental health determinants revealed through triage analysis, these approaches acknowledge and address the full spectrum of factors that influence health outcomes.
This comprehensive approach to prevention represents healthcare at its best—patient-centered, data-informed, cost-effective, and focused on improving lives rather than merely treating diseases. The implementation challenges described in this article—from data standardization obstacles to workflow integration hurdles—are real but surmountable, as demonstrated by the growing number of organizations successfully deploying these approaches. The combination of technical strategies (data integration, standardized documentation, analytics capabilities) with human factors approaches (change management, user-centered design, prevention-oriented culture) provides a roadmap for healthcare systems beginning their triage-informed prevention journeys. As technologies continue to advance, particularly in artificial intelligence, natural language processing, and precision medicine approaches, the potential impact of triage-based prevention will only increase. Organizations that invest now in building the data foundations, analytics capabilities, and prevention-oriented workflows will position themselves at the forefront of healthcare's evolution toward more proactive, personalized models of care delivery.
The future of healthcare lies not in ever more sophisticated treatments for advanced disease but in increasingly precise prevention of disease progression before severe symptoms ever develop. Triage data—collected across settings, analyzed systematically, and translated into tailored interventions—represents one of our most powerful tools for realizing this vision. By transforming the rich information gathered during triage from mere sorting mechanisms to prevention catalysts, healthcare organizations can identify intervention opportunities that would otherwise be missed, implementing targeted prevention strategies that improve outcomes while reducing costs. The preventive care pathways revealed through triage data analysis offer a practical approach to addressing healthcare's most persistent challenges—unsustainable costs, inconsistent quality, and health disparities—by focusing resources where they can have the greatest impact. In an era of healthcare reform focused on value rather than volume, triage-informed prevention represents not just a clinical imperative but a strategic necessity for organizations committed to thriving in healthcare's future.
FAQ Section
1. What is triage data and how does it relate to preventive care? Triage data is information collected during initial patient assessments across healthcare settings. It relates to preventive care by revealing patterns that help identify intervention opportunities before conditions worsen, enabling proactive rather than reactive healthcare approaches.
2. How do preventive care pathways reduce healthcare costs? Preventive care pathways reduce costs by identifying health risks early, enabling interventions before conditions require expensive emergency care or hospitalizations. Data shows implementation of these pathways can achieve ROI ratios between 2.4:1 and 5.2:1 through reduced utilization and improved quality metrics.
3. What types of prevention opportunities can triage data reveal? Triage data can reveal pre-crisis interventions (imminent deterioration), secondary prevention opportunities (preventing complications in existing conditions), primary prevention opportunities (preventing disease onset), and health equity intervention opportunities (addressing systematic disparities in care).
4. Which healthcare settings can benefit from triage-based preventive care? All healthcare settings can benefit, including emergency departments, primary care practices, specialty clinics, telehealth platforms, school-based health centers, and community health programs. The comprehensive value comes when triage data is integrated across these settings.
5. What role does AI play in triage-based preventive care? AI enhances triage-based prevention by identifying complex patterns across thousands of variables that traditional analysis would miss, extracting insights from unstructured notes through natural language processing, enabling real-time prevention recommendations, and facilitating personalized prevention approaches tailored to individual risk profiles.
6. How can healthcare organizations implement triage-based prevention pathways? Implementation requires multidisciplinary prevention pathway teams, workflow-integrated alerts, community extension models, and sustainable financial approaches that demonstrate return on investment. Successful implementation balances data-driven insights with practical clinical workflow considerations.
7. What challenges exist in using triage data for prevention? Key challenges include data quality/standardization issues, siloed information systems that prevent comprehensive analysis, difficulty distinguishing meaningful patterns from statistical noise, and human factors like alert fatigue and competing priorities that impact adoption of prevention recommendations.
8. How does triage data help address social determinants of health? Modern triage protocols increasingly include standardized assessments of social needs, creating datasets that reveal connections between social factors and clinical presentations. This enables integrated interventions addressing both medical and social drivers of health outcomes.
9. What metrics should organizations track to evaluate prevention pathway effectiveness? Key metrics include reductions in emergency department visits and hospitalizations, cost savings per patient, return on investment ratios, quality metric improvements, and condition-specific outcomes like symptom-free days or disease control measures.
10. How is triage-based prevention evolving with new technologies? Triage-based prevention is evolving through real-time predictive analytics that deliver immediate intervention recommendations, natural language processing that unlocks insights from narrative documentation, and precision medicine approaches that create highly personalized prevention pathways tailored to individual risk profiles.
Additional Resources
For readers interested in exploring triage-based preventive care pathways further, the following resources provide valuable additional information:
1. [Agency for Healthcare Research and Quality (AHRQ) - Triage Tools and Prevention Models](https://www.ahrq.gov/research/findings/evidence-based-reports/index.html) - Comprehensive evidence-based resources on triage optimization and preventive care implementation.
2. [National Academy of Medicine - Effective Care Pathways](https://nam.edu/programs/value-science-driven-health-care/) - Research and policy recommendations on transforming healthcare delivery through data-driven prevention approaches.
3. [Institute for Healthcare Improvement - Preventive Care Resources](https://www.ihi.org/Topics/TripleAim/Pages/default.aspx) - Practical tools and implementation guides for organizations developing preventive care pathways.
4. [CDC Preventive Services Resources](https://www.cdc.gov/preventiveservices/index.html) - Evidence-based prevention guidelines and implementation strategies across various conditions and populations.
5. [Health Affairs Research Collection: The Value of Prevention](https://www.healthaffairs.org/topic/ss44) - Peer-reviewed research on the economic and clinical impact of preventive care approaches.