Multimodal AI Triage Combining Voice, Text, and Vision
Explore how multimodal AI triage is transforming healthcare by integrating voice, text, and visual data for enhanced patient assessment and better diagnostic outcomes. Dive into the latest advancements, case studies, and practical applications of this cutting-edge technology.
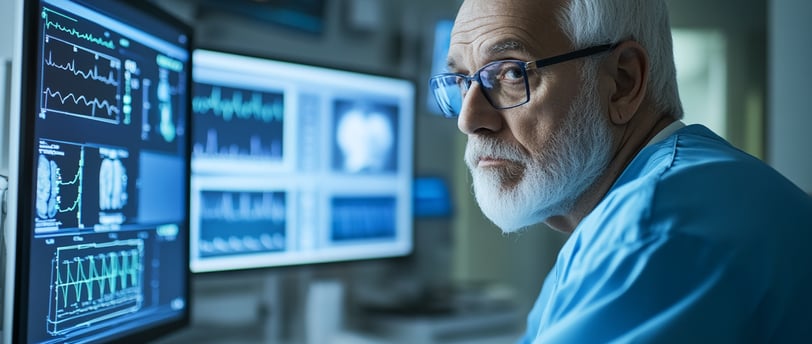
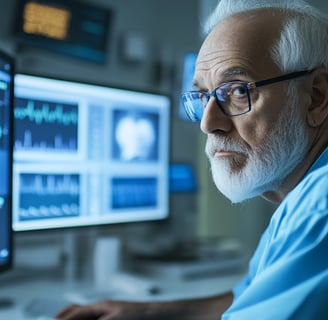
Imagine walking into a hospital emergency room, but instead of waiting hours to see a doctor, you're greeted by an AI-driven triage system that can assess your condition in minutes. This system combines voice inputs, textual data from your medical records, and visual inputs from medical images to provide a comprehensive evaluation. Welcome to the future of healthcare, where multimodal AI triage is revolutionising patient assessment and diagnostics. In this article, we'll explore the fascinating world of multimodal AI, its applications in healthcare, and how it's transforming how we approach patient care.
The Rise of Multimodal AI in Healthcare
Multimodal AI integrates multiple data modalities—text, images, and audio—to create more accurate and comprehensive AI models. This means combining patient records, medical images, and voice inputs in healthcare to enhance diagnostic accuracy and improve patient outcomes.
Enhancing Diagnostic Accuracy
One of the primary advantages of multimodal AI in healthcare is its ability to enhance diagnostic accuracy. By integrating data from various sources, AI models can provide a more holistic view of a patient's condition. For instance, a multimodal AI system can analyse X-ray images, patient symptoms described in text, and even the sound of a patient's cough to diagnose respiratory conditions more accurately. This approach has been efficient in radiology, where visual and textual data are crucial for diagnosis1.
Improving Patient Triage
Patient triage is the process of determining the priority of patients' treatments based on the severity of their condition. Traditional triage methods often rely on manual assessments, which can be time-consuming and subject to human error. Multimodal AI triage systems, however, can quickly analyse a patient's vital signs, medical history, and even real-time visual data to prioritise care more effectively. For example, AI chatbots can provide initial assessments and direct patients to the appropriate level of care, reducing wait times and optimising hospital resources2.
Real-World Applications
The applications of multimodal AI in healthcare are vast and varied. From automated remote assessment of neurological and mental health conditions to enhancing user experience in telemedicine, multimodal AI is a game-changer. For instance, AI-driven systems can analyse linguistic and acoustic cues to monitor patients with neurological disorders, providing real-time insights and improving treatment outcomes3.
Key Components of Multimodal AI Triage
Voice Inputs
Voice recognition technology allows patients to describe their symptoms naturally, making the triage process more intuitive and accessible. AI models can analyse the tone, pitch, and emotional state of the patient's voice to provide more accurate assessments. For example, voice inputs can be used to detect signs of stress or anxiety in a patient's voice, which can be crucial for mental health assessments.
Textual Data
Textual data, such as patient records and clinical notes, provide valuable context for AI models. By analysing this data, AI can identify patterns and correlations that human clinicians might miss. For instance, textual data can predict the risk of diseases like asthma by analysing patient history and clinical notes.
Visual Inputs
Medical images, such as X-rays, MRIs, and CT scans, are essential for diagnosing many conditions. Multimodal AI systems can analyse these images with other data modalities to provide more accurate diagnoses. For example, AI can detect abnormalities in medical images and cross-reference them with patient records to provide a comprehensive assessment6.
Case Studies and Success Stories
Case Study 1: Improving Radiology Workflows
In radiology, multimodal AI has optimised workflows and improved diagnostic accuracy. For instance, AI-generated findings can be used for triage and early flagging of critical findings, allowing radiologists to prioritise their workload more effectively. This approach has been shown to reduce the time required for image analysis and improve the overall efficiency of radiology departments1.
Case Study 2: Enhancing Telemedicine
Telemedicine has become increasingly popular, especially after the COVID-19 pandemic. Multimodal AI systems can enhance telemedicine by providing more interactive and intuitive user experiences. For example, patients can describe their symptoms using voice inputs, while clinicians can access real-time visual data and patient records to provide more accurate diagnoses7.
Case Study 3: Monitoring Neurological Conditions
Multimodal AI has also been used to monitor neurological conditions remotely. By analysing linguistic and acoustic cues, AI systems can provide real-time insights into a patient's condition, allowing for early intervention and improved treatment outcomes. This approach has been efficient in monitoring conditions like Parkinson's disease3.
Challenges and Considerations
While multimodal AI holds immense promise for healthcare, several challenges and considerations must be considered.
Data Privacy and Security
One of the primary concerns with multimodal AI is data privacy and security. Healthcare data is sensitive and must be protected from unauthorised access. AI systems must be designed with robust security measures to ensure patient data remains confidential and secure.
Ethical Considerations
The ethical implications of using AI in healthcare are significant. AI systems must be designed to be fair, unbiased, and transparent. It's crucial to ensure that AI algorithms do not perpetuate existing biases or discriminate against certain patient groups.
Integration with Existing Systems
Integrating multimodal AI systems with existing healthcare infrastructure can be challenging. Healthcare organisations must ensure that AI systems are compatible with their current technologies and workflows. Additionally, healthcare professionals must be trained to use these new systems effectively.
Conclusion
Multimodal AI triage is revolutionising healthcare by integrating voice, text, and visual data to provide more accurate and comprehensive patient assessments. From enhancing diagnostic accuracy to improving patient triage and remote monitoring, the applications of multimodal AI in healthcare are vast and varied. As we continue to explore the possibilities of this cutting-edge technology, it's essential to address the challenges and considerations related to data privacy, ethical implications, and integration with existing systems. By embracing multimodal AI, we can pave the way for a future where healthcare is more personalised, efficient, and effective for everyone.
FAQ Section
What is multimodal AI?
Multimodal AI integrates multiple data modalities, such as text, images, and audio, to create more accurate and comprehensive AI models.
How does multimodal AI improve diagnostic accuracy?
By integrating data from various sources, multimodal AI provides a more holistic view of a patient's condition, enhancing diagnostic accuracy.
What are the key components of multimodal AI triage?
The key components include voice inputs, textual data, and visual inputs, which are analysed to provide comprehensive patient assessments.
How does multimodal AI enhance patient triage?
Multimodal AI triage systems can quickly analyse a patient's vital signs, medical history, and real-time visual data to prioritise care more effectively.
What are some real-world applications of multimodal AI in healthcare?
Applications include improving radiology workflows, enhancing telemedicine, and monitoring neurological conditions remotely.
What are the challenges of implementing multimodal AI in healthcare?
Challenges include data privacy and security, ethical considerations, and integration with existing healthcare systems.
How does multimodal AI improve patient satisfaction?
By providing more personalised and efficient care, multimodal AI has been linked to increased patient satisfaction.
Can multimodal AI reduce unnecessary emergency room visits?
Yes, AI chatbots and triage systems can reduce unnecessary ER visits by directing patients to the appropriate level of care.
How does multimodal AI enhance remote monitoring?
Multimodal AI systems improve the effectiveness of remote monitoring for neurological and mental health conditions by providing real-time insights.
What is the future of multimodal AI in healthcare?
The future of multimodal AI in healthcare is promising, with potential applications in various fields and continuous technological advancements.
Additional Resources