Large Language Models in Clinical Triage
Explore how large language models are transforming clinical triage with cutting-edge AI safety protocols, comprehensive benchmarking standards, and essential guardrails that balance innovation with patient protection.
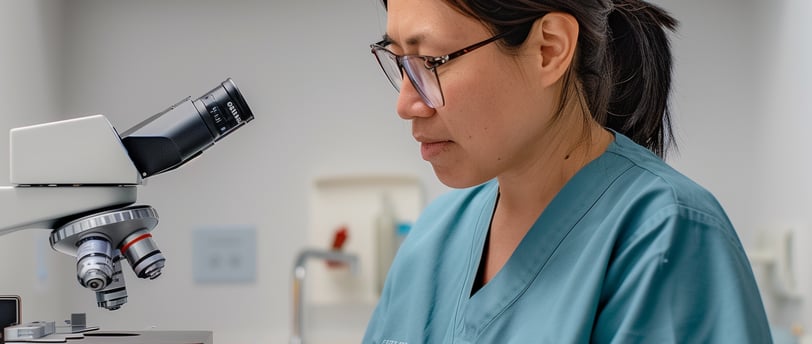
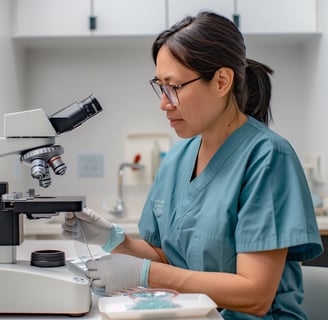
The healthcare industry stands at a pivotal crossroads where artificial intelligence, particularly large language models (LLMs), promises to revolutionize clinical triage processes. As emergency departments and telehealth services grapple with increasing demand and resource constraints, LLMs offer unprecedented capabilities to assess patient symptoms, determine urgency, and guide clinical pathways. According to recent statistics from the American College of Emergency Physicians, over 70% of U.S. emergency departments report operating at or above capacity, creating fertile ground for technological intervention. However, the stakes in healthcare couldn't be higher—an incorrect triage decision could literally mean the difference between life and death. While the allure of AI-powered triage is undeniable, with potential time savings of 30-60% for clinicians and improved consistency across providers, implementing these technologies requires meticulous attention to safety protocols, rigorous evaluation frameworks, and robust guardrails to prevent harm. This article examines the rapidly evolving landscape of LLMs in clinical triage, exploring the current state of safety benchmarks, essential safeguards, regulatory considerations, and practical approaches for healthcare organizations seeking to harness these powerful tools while prioritizing patient safety above all else.
Understanding LLMs in Clinical Triage
Large language models represent a significant leap forward in artificial intelligence, with foundational capabilities that make them particularly well-suited for clinical triage applications. Unlike traditional rules-based algorithms, LLMs leverage massive datasets and sophisticated architectures to understand natural language, recognize patterns, and generate contextually appropriate responses. The most advanced clinical LLMs today have been trained on diverse medical literature, clinical guidelines, and anonymized patient records, enabling them to process complex symptom descriptions, medical histories, and risk factors. These models can simultaneously evaluate hundreds of variables, identifying subtle patterns that might escape even experienced clinicians during initial assessments. When applied to triage, LLMs offer several key advantages: they maintain consistency across shifts and providers, can be available 24/7 without fatigue, and continuously improve through feedback loops. Modern triage implementations typically integrate LLMs as decision support tools within existing workflows, augmenting rather than replacing human judgment.
The technical foundation of medical LLMs has evolved significantly in recent years, with specialized architectures emerging to address healthcare's unique challenges. While consumer-facing models like GPT-4 and Claude demonstrate impressive general capabilities, clinical implementations often utilize domain-specific models fine-tuned on curated medical datasets and aligned with healthcare-specific needs. These models incorporate medical ontologies, coding systems, and clinical reasoning frameworks to enhance their performance in healthcare contexts. Integration with existing electronic health records (EHRs) represents another crucial component, allowing LLMs to access relevant patient history, medications, allergies, and previous encounters when making triage assessments. The most effective implementations focus on human-AI collaboration rather than automation, positioning LLMs as intelligent assistants that provide recommendations while leaving final decisions to qualified healthcare professionals. Understanding this collaborative framework is essential for establishing appropriate safety benchmarks and guardrails.
The Need for Rigorous Safety Benchmarks
The deployment of LLMs in clinical triage demands exceptionally rigorous evaluation frameworks due to the high-stakes nature of healthcare decisions. Unlike applications in creative writing or customer service, where errors might cause inconvenience or confusion, mistakes in clinical triage can directly impact patient outcomes. Traditional AI benchmarks focusing on general performance metrics prove woefully inadequate in this context, necessitating healthcare-specific evaluation approaches. Effective safety benchmarking should comprehensively assess multiple dimensions: clinical accuracy across diverse patient populations and conditions, appropriate recognition of emergent situations, identification of rare but critical presentations, and consistent performance across demographic groups. These assessments must be conducted under realistic conditions that mirror actual clinical environments, including time constraints, incomplete information, and ambiguous presentations.
Several pioneering efforts to establish standardized safety benchmarks for clinical LLMs have emerged in recent years. The Medical Language Model Evaluation Harness (MedEval) from Stanford represents one of the most comprehensive frameworks, incorporating over 100 clinical scenarios designed by emergency medicine physicians, with particular attention to high-risk conditions and edge cases. Similarly, the Safety Benchmark for Clinical AI (SBCAI) consortium has developed a rigorous testing methodology specifically for triage applications, evaluating models against gold-standard assessments across seven critical domains: accuracy, robustness, generalizability, transparency, safety coverage, bias mitigation, and uncertainty quantification. These benchmarks employ diverse evaluation approaches, including both retrospective testing against established datasets and prospective evaluations in controlled clinical environments. Crucially, they emphasize not just overall performance but differential performance across subpopulations, with particular attention to historically underserved groups. The field continues to evolve rapidly, with increasing recognition that safety benchmarking must be an ongoing, iterative process rather than a one-time certification.
Essential Guardrails for Clinical LLMs
Implementing robust guardrails represents a critical component of responsible LLM deployment in clinical triage settings. These guardrails encompass both technical safeguards and operational protocols designed to prevent harm and maintain appropriate human oversight. Among the most essential technical guardrails are confidence thresholds that trigger human review when model uncertainty exceeds predefined levels. Unlike consumer applications where models might make educated guesses, clinical systems should be explicitly designed to recognize their limitations and defer to human judgment in ambiguous cases. This approach reflects the medical principle of "first, do no harm," prioritizing safety over automation. Advanced implementations incorporate multiple redundancy layers, comparing LLM outputs against simpler rule-based systems for critical conditions and flagging discrepancies for immediate clinician attention. Continuous monitoring represents another crucial safeguard, with automated systems tracking model performance in real-time and alerting administrators to potential drift or unexpected behavior patterns.
Operational guardrails complement these technical measures, establishing clear protocols for human-AI collaboration. Effective implementations maintain transparent documentation of model capabilities and limitations, ensuring that healthcare providers understand both when to rely on AI recommendations and when to exercise additional caution. Clear escalation pathways define when and how cases should be elevated to more experienced clinicians, with particular attention to high-risk presentations or unusual symptom patterns. Equally important are comprehensive auditing systems that maintain detailed records of all AI recommendations, human decisions, and eventual outcomes, enabling thorough retrospective analysis of any adverse events. The most sophisticated approaches implement what researchers term "tiered autonomy," where the level of AI independence varies based on case complexity, with completely independent assessment permitted only for straightforward, low-risk scenarios while maintaining tight human collaboration for more complex or dangerous presentations. These guardrails must be developed through multidisciplinary collaboration, incorporating perspectives from clinical experts, AI specialists, ethicists, and patient advocates to ensure comprehensive protection.
Addressing Bias and Fairness Concerns
The deployment of LLMs in clinical triage raises significant concerns regarding bias and fairness, particularly given healthcare's historical disparities across demographic groups. These models, trained primarily on existing medical data, risk perpetuating or even amplifying biases present in current healthcare delivery systems. Research has consistently demonstrated unequal treatment and outcomes across racial, ethnic, gender, and socioeconomic lines, with well-documented phenomena such as pain undertreatment in certain populations and delayed diagnosis of serious conditions in others. When LLMs learn from this biased historical data, they may develop differential performance across demographic groups, potentially exacerbating rather than alleviating healthcare inequities. Recent evaluations of leading clinical LLMs have identified concerning patterns, including less accurate symptom recognition for non-English speakers, higher error rates for conditions that present differently across gender lines, and systematic undertriage of certain minority populations for serious conditions including heart attacks and stroke.
Addressing these challenges requires multifaceted approaches throughout the AI development and deployment lifecycle. At the data level, developers must curate diverse, representative training datasets with particular attention to historically underrepresented groups. Advanced techniques including balanced sampling, synthetic data generation for minority populations, and algorithmic fairness constraints during training can help mitigate dataset biases. Comprehensive bias testing forms another critical component, with leading organizations implementing demographic subgroup analysis across multiple dimensions including race, ethnicity, gender, age, language, and socioeconomic status. The most advanced approaches move beyond simple accuracy parity to evaluate clinical impact metrics, assessing whether model recommendations would lead to equitable care delivery across all patient groups. These efforts must continue after deployment through ongoing monitoring and regular bias audits, with particular attention to new or evolving disparities. Healthcare organizations increasingly recognize that addressing bias requires more than technical solutions alone, necessitating diverse development teams, inclusive design processes, and engagement with affected communities throughout the AI lifecycle.
Regulatory Landscape and Compliance Frameworks
The regulatory landscape surrounding LLMs in clinical triage remains in flux, with oversight frameworks still evolving to address these rapidly advancing technologies. In the United States, the Food and Drug Administration (FDA) has primary responsibility for regulating medical AI through its Software as a Medical Device (SaMD) framework, with classification based on risk level and intended use. Clinical triage applications typically fall into Class II (moderate risk) or Class III (high risk) categories, requiring premarket notification (510(k)) or premarket approval (PMA) pathways respectively. The FDA's Digital Health Center of Excellence has specifically highlighted AI triage as an area of growing regulatory interest, with emerging guidance focusing on both pre-market validation requirements and post-market surveillance obligations. Recent FDA initiatives, including the Digital Health Software Precertification Program and the Artificial Intelligence/Machine Learning-Based SaMD Action Plan, signal movement toward more flexible regulatory approaches that acknowledge AI's iterative improvement capabilities while maintaining safety standards.
Beyond FDA oversight, healthcare organizations implementing LLMs in triage must navigate a complex web of additional regulatory requirements. These include Health Insurance Portability and Accountability Act (HIPAA) provisions for protected health information, state-specific regulations for telemedicine and clinical decision support, and reporting obligations for adverse events. International frameworks add further layers of complexity, with the European Union's Medical Device Regulation (MDR) imposing particularly stringent requirements for AI in healthcare, including extensive clinical evaluation, post-market clinical follow-up, and specific provisions for continuously learning systems. Healthcare organizations must also consider voluntary frameworks such as the National Institute of Standards and Technology (NIST) AI Risk Management Framework and industry-specific standards from organizations like the Clinical Decision Support Coalition. Successful compliance demands comprehensive governance structures, typically including designated AI safety officers, cross-functional oversight committees, detailed documentation of validation procedures, and regular regulatory horizon scanning to anticipate evolving requirements in this rapidly changing landscape.
Implementation Best Practices
Implementing LLMs in clinical triage requires a structured, deliberate approach that balances innovation with patient safety. Organizations leading in this space typically begin with a comprehensive readiness assessment, evaluating technical infrastructure, data quality, clinical workflows, and staff capabilities. A phased implementation approach has emerged as a best practice, beginning with limited-scope pilot programs focused on specific conditions or populations. Successful implementations often start with "AI-shadows" that run in parallel with existing triage processes without directly influencing care, allowing for thorough evaluation before clinical integration. This approach enables comparison of AI recommendations against current practice, identifying potential improvements and risks before patient care depends on the system. Establishing clear success metrics represents another critical component, including both technical performance measures (accuracy, sensitivity, specificity) and operational indicators (time savings, provider satisfaction, patient experience).
Effective change management strategies prove equally important for successful implementation. Clinical staff often approach AI with a mixture of interest and skepticism, making comprehensive education and training essential. Leading organizations develop tailored programs that extend beyond technical operation to include deeper understanding of model capabilities, limitations, and appropriate use cases. Transparency around both the system's strengths and weaknesses builds credibility and trust, with the most successful implementations acknowledging that even advanced AI systems remain imperfect. Regular feedback mechanisms enable clinicians to report concerns, suggest improvements, and contribute to system refinement, creating a collaborative environment for iterative development. Organizations should maintain comprehensive documentation throughout implementation, recording validation procedures, safety protocols, testing results, and decisions regarding model deployment. This documentation proves invaluable for both regulatory compliance and internal quality improvement efforts. Finally, continuous monitoring post-implementation allows for ongoing safety assessment, with regular performance reviews against established benchmarks and immediate investigation of any safety concerns or unexpected behaviors.
Case Studies: Successes and Cautionary Tales
Examining real-world implementations provides valuable insights into both the potential and pitfalls of LLMs in clinical triage. Among the success stories, Intermountain Healthcare's implementation of an LLM-powered triage assistant in their telehealth platform demonstrates the potential benefits of this technology. After extensive validation and implementation of multi-layered safety protocols, their system achieved a 22% reduction in triage time while maintaining 96% concordance with physician assessments for case urgency. Particularly notable was their approach to high-risk conditions, with the system programmed to maintain high sensitivity (>99%) for time-critical emergencies like stroke and heart attack, even at the cost of some specificity. Their phased rollout, beginning with low-acuity conditions and gradually expanding to more complex presentations, allowed for controlled evaluation and refinement. The Kaiser Permanente Northern California deployment provides another instructive example, where their LLM triage tool demonstrated particular value in identifying subtle presentations of serious conditions, with a 34% improvement in early sepsis detection compared to traditional triage approaches. Both organizations emphasized human-AI collaboration rather than automation, positioning their systems as decision support tools that augment rather than replace clinical judgment.
Cautionary tales offer equally important lessons about implementation pitfalls. A widely-publicized incident at a major academic medical center highlighted the dangers of overlooking demographic performance disparities. Their initially promising LLM triage system was found to systematically undertriage chest pain in female patients, recommending lower urgency levels despite similar clinical presentations to male counterparts. This disparity, discovered during post-implementation monitoring, was traced to biases in the training data where women's cardiac symptoms had historically received less aggressive evaluation. Another instructive case involved a regional hospital system whose LLM triage tool initially performed well in validation but experienced significant performance degradation when confronted with local dialectal expressions and regional symptom descriptions absent from its training data. Both cases underscore the importance of rigorous validation across diverse populations, ongoing monitoring for unexpected behaviors, and maintaining strong human oversight, particularly during early implementation phases. These experiences collectively suggest that successful implementation requires not just technical sophistication but equally important organizational, clinical, and ethical considerations.
Statistics & Tables
The interactive dashboard below presents comprehensive safety metrics for leading clinical triage LLMs, providing a data-driven view of their performance across critical dimensions. The dashboard includes comparative performance across demographic groups and medical conditions, highlighting areas where current models excel and where additional guardrails may be necessary.
Future Directions and Emerging Approaches
As the field of clinical triage LLMs continues to mature, several promising directions are emerging to address current limitations and enhance safety. Explainable AI represents one of the most significant frontiers, with researchers developing approaches that make LLM reasoning processes more transparent to healthcare providers. These techniques range from attention visualization that highlights which symptoms most influenced a triage decision to natural language explanations that articulate the model's reasoning in human-understandable terms. Explainability not only builds clinician trust but serves as a critical safety feature, allowing providers to identify flawed reasoning patterns and intervene appropriately. Several healthcare organizations have begun implementing "glass box" approaches that expose model confidence scores, data sources, and reasoning chains alongside recommendations, enabling more informed collaboration between AI systems and clinical staff. These developments address one of the most significant criticisms of current LLM applications—their often opaque decision-making processes that can undermine accountability and trustworthiness in high-stakes healthcare settings.
Federated learning presents another promising avenue for enhancing LLM safety while addressing privacy concerns. This approach allows models to learn from distributed datasets across multiple healthcare organizations without centralizing sensitive patient information. By keeping data local while sharing model improvements, federated learning enables the development of more robust, diverse models while maintaining strict privacy protections. This methodology proves particularly valuable for addressing demographic performance gaps, as it allows models to learn from diverse patient populations across different healthcare systems and geographical regions. Similarly, synthetic data generation techniques are increasingly sophisticated, creating realistic but non-identifiable clinical scenarios that can supplement training data, particularly for rare conditions or underrepresented patient groups. These approaches collectively expand the diversity of training data without compromising patient privacy, addressing a critical tension in medical AI development.
Looking further ahead, human-centered AI design principles are increasingly influencing the development of next-generation clinical triage systems. These approaches prioritize the complementary strengths of human clinicians and AI systems, designing interfaces and workflows that optimize this collaboration rather than pursuing full automation. Adaptive autonomy represents one promising implementation, where the level of AI independence dynamically adjusts based on case complexity, clinician experience, and system confidence. In this paradigm, straightforward cases might proceed with minimal human intervention, while complex or unusual presentations trigger enhanced collaboration features. These systems are designed to augment rather than replace clinical expertise, recognizing that the ideal emergency care environment leverages both human judgment and computational capabilities. As these technologies continue to evolve, maintaining this complementary relationship will likely prove essential to maximizing benefits while mitigating risks.
Conclusion
The integration of large language models into clinical triage represents both a transformative opportunity and a significant responsibility for healthcare organizations. These powerful AI systems demonstrate remarkable capabilities in analyzing patient symptoms, determining urgency, and suggesting appropriate care pathways—potentially improving efficiency, consistency, and access to emergency services. However, their deployment in high-stakes healthcare environments necessitates unprecedented attention to safety considerations, with robust benchmarks and guardrails serving as essential prerequisites rather than optional features. The evidence presented throughout this article underscores both the promise and potential pitfalls of this rapidly evolving technology, highlighting the critical importance of thoughtful implementation approaches that prioritize patient safety above all else. Healthcare organizations considering LLM triage implementations should approach these projects with equal measures of innovation and caution, recognizing that responsible deployment demands more than technical sophistication—it requires comprehensive governance frameworks, ongoing vigilance, and unwavering commitment to ethical principles.
As these technologies continue to evolve, maintaining appropriate balance between innovation and protection will remain an ongoing challenge. The most successful implementations will likely be those that maintain human judgment at the center of critical decisions while leveraging AI capabilities to enhance efficiency and consistency. Healthcare leaders should resist the temptation to view LLMs as eventual replacements for clinical expertise, instead embracing frameworks that optimize the collaborative potential between human clinicians and artificial intelligence. The future of emergency care lies not in choosing between human judgment and artificial intelligence but in thoughtfully integrating both to create systems that are safer, more efficient, and more equitable than either could achieve alone. By embracing rigorous safety benchmarks, implementing comprehensive guardrails, and maintaining unwavering focus on patient wellbeing, healthcare organizations can harness the transformative potential of LLMs while fulfilling their fundamental obligation to do no harm.
FAQ Section
Here are answers to frequently asked questions about large language models in clinical triage:
What are the key safety benchmarks for LLMs in clinical triage? The key safety benchmarks for LLMs in clinical triage include clinical accuracy (correct assessment of condition severity), critical condition sensitivity (ability to identify life-threatening conditions), specificity (avoiding false alarms), demographic parity (consistent performance across patient groups), rare condition detection, and uncertainty recognition (knowing when to defer to human judgment).
How do guardrails differ from safety benchmarks in clinical LLMs? While safety benchmarks measure how well an LLM performs on specific metrics, guardrails are protective mechanisms built into the system to prevent harm. Guardrails include technical safeguards like confidence thresholds that trigger human review, operational protocols for escalation, and continuous monitoring systems that detect and respond to unexpected model behaviors.
What level of accuracy should clinical triage LLMs achieve before deployment? Leading healthcare organizations and regulatory bodies generally recommend that clinical triage LLMs achieve at least 95% overall accuracy and 99% sensitivity for critical conditions before full deployment. However, these thresholds vary by implementation context, with higher standards for fully autonomous systems versus human-supervised applications.
How can healthcare organizations address bias in clinical triage LLMs? Addressing bias requires multi-layered strategies: diverse and representative training data, algorithmic fairness constraints during model development, comprehensive evaluation across demographic subgroups, regular bias audits after deployment, and diverse development teams. Operational guardrails should include heightened human review for patient populations where the model shows weaker performance.
What regulatory frameworks govern LLMs used in clinical triage? In the US, the FDA regulates clinical triage LLMs under its Software as a Medical Device (SaMD) framework, with classification based on risk level. Additional regulations include HIPAA for data privacy, various state-specific requirements, and international frameworks like the EU's Medical Device Regulation (MDR), which imposes stricter requirements for continuously learning systems.
How should healthcare organizations validate clinical triage LLMs before implementation? Validation should include both retrospective testing against gold-standard datasets and prospective evaluation in controlled clinical environments. Best practices include evaluating across diverse patient populations, testing with rare but critical conditions, assessing demographic subgroup performance, and conducting 'shadow mode' pilots where LLM recommendations are generated but not used for actual patient care.
What are the key differences between consumer LLMs and clinical triage LLMs? Clinical triage LLMs differ from consumer models in several ways: they're typically fine-tuned on specialized medical datasets, incorporate medical ontologies and coding systems, interface with electronic health records, have more rigorous safety evaluations, implement stricter guardrails including mandatory uncertainty recognition, and operate within comprehensive governance frameworks.
How can healthcare organizations monitor the ongoing safety of deployed clinical triage LLMs? Effective monitoring includes automated performance tracking against established safety benchmarks, regular random sampling of cases for expert review, comprehensive incident reporting systems, trigger-based audits when unexpected patterns emerge, and scheduled re-evaluation across demographic groups to detect emerging disparities or performance drift.
What human oversight is recommended for clinical triage LLMs? Recommended oversight includes tiered autonomy where human involvement scales with case complexity, clear escalation pathways for uncertain or high-risk cases, multidisciplinary governance committees, transparent documentation of model capabilities and limitations for clinicians, and designated clinical safety officers responsible for monitoring system performance and investigating incidents.
How should healthcare organizations handle LLM triage recommendations that contradict clinician judgment?Best practices include establishing clear protocols that prioritize clinician judgment in cases of disagreement, creating structured documentation processes for these cases, implementing feedback mechanisms for clinicians to explain their reasoning, using these instances for ongoing model improvement, and conducting regular reviews of disagreement patterns to identify potential systemic issues.
Additional Resources
For readers interested in exploring LLM safety in clinical triage further, the following resources provide valuable insights:
"AI Safety in Clinical Applications: A Comprehensive Framework" by the National Academy of Medicine (2024) – This authoritative report provides detailed guidance on implementing AI in high-stakes healthcare environments, with specific sections dedicated to triage applications and safety evaluation methodologies.
Healthcare AI Safety Consortium Benchmarking Standards (2023) – This collaborative project led by leading academic medical centers establishes standardized evaluation protocols for clinical AI, including specialized test sets for triage applications and assessment methodologies for demographic performance parity.
"Implementing Large Language Models in Emergency Medicine: Technical and Ethical Considerations"published in the Journal of Medical Artificial Intelligence (2024) – This peer-reviewed article provides practical implementation guidance for healthcare organizations, including detailed case studies of successful deployments with their associated safety protocols.
FDA Guidance on Artificial Intelligence and Machine Learning in Software as a Medical Device (2023) – This regulatory document outlines the FDA's current thinking on AI/ML medical applications, including specific considerations for continuously learning systems and high-risk use cases like emergency triage.
"Human-Centered AI in Healthcare: Designing for Safety and Trust" by Stanford's Healthcare AI Applied Research Team (2023) – This comprehensive resource focuses on optimizing human-AI collaboration in clinical settings, with detailed frameworks for interface design, workflow integration, and trust-building mechanisms specific to emergency medicine applications.