Impact of Federated Learning on Healthcare Triage Privacy
Explore how federated learning revolutionises healthcare triage privacy by enabling collaborative AI model training across institutions while preserving patient data confidentiality.
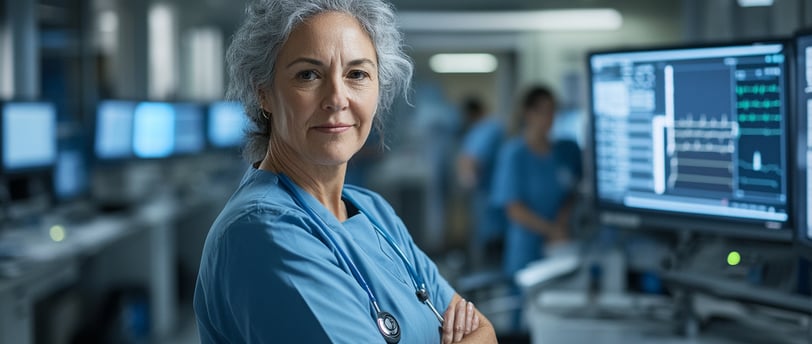
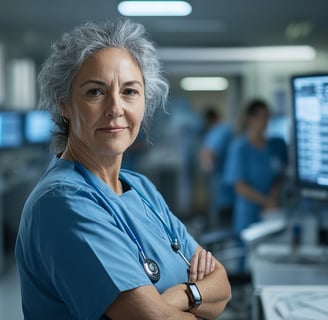
Imagine a world where healthcare institutions can collaborate to improve patient outcomes without compromising privacy. Federated learning makes this vision a reality. By enabling AI models to be trained across multiple institutions without sharing sensitive data, federated learning addresses critical privacy concerns in healthcare triage. This article delves into the transformative potential of federated learning in healthcare, its applications, challenges, and the future of privacy-preserving AI in medical settings.
Understanding Federated Learning in Healthcare
What is Federated Learning?
Federated learning is a machine learning paradigm where a model is trained across multiple decentralised devices or servers holding local data samples without exchanging them. This approach is particularly valuable in healthcare, where data privacy is paramount. Instead of centralising data, federated learning allows institutions to train models while keeping patient data local collaboratively, ensuring compliance with regulations like HIPAA and GDPR. The general solutions to the statistical challenges, system challenges, and privacy issues in federated learning point out the implications and potentials in healthcare12.
Applications in Healthcare Triage
Predictive Analytics: Federated learning can enhance predictive models for patient triage by training on diverse datasets from multiple hospitals. For example, models can more accurately predict hospital readmission risks by learning from varied patient populations without sharing sensitive data1.
Real-Time Monitoring: Integration with IoT devices and wearables enables real-time monitoring and predictive analytics. Federated learning allows these devices to contribute to model training without compromising patient privacy3.
Personalized Medicine: Federated learning can help tailor treatments to individual patients, improving outcomes and reducing adverse effects by analysing data from various sources. This personalised approach is crucial in oncology and cardiology, where treatment responses vary widely2.
Challenges and Solutions
Data Heterogeneity
One of the main challenges in federated learning is data heterogeneity. Healthcare data is often fragmented and varies significantly across institutions. Ensuring that models trained on such diverse data are generalisable is complex. Solutions include using transfer learning and domain adaptation techniques to bridge the gap between different data distributions4.
Communication Efficiency
Training models across multiple institutions require efficient communication protocols. Federated learning must optimise the exchange of model updates to minimise bandwidth usage and latency. Techniques like model compression and selective parameter updating can help address these issues.
Privacy and Security
While federated learning inherently protects data privacy by keeping data local, it is not immune to security threats. Malicious actors could still infer sensitive information from model updates. Differential privacy and secure multi-party computation can enhance security and prevent data leakage6.
Case Studies
1. Multi-Institutional Collaboration for Disease Prediction
A consortium of hospitals used federated learning to develop a model for predicting heart disease. The consortium achieved higher accuracy by training the model on data from multiple institutions than models trained on single-institution data. This collaborative approach improved patient outcomes without compromising data privacy1.
2. Smart Healthcare Systems with IoT Integration
A healthcare provider integrated federated learning with IoT devices to monitor patients in real time. The system used data from wearables to predict and prevent adverse events, such as falls or cardiac episodes, without sharing sensitive data with external servers. This implementation demonstrated the potential of federated learning in enhancing patient safety and care quality3.
Conclusion
Federated learning revolutionises healthcare triage by enabling collaborative AI model training while preserving patient privacy. As healthcare institutions increasingly adopt this technology, the potential for improved patient outcomes and enhanced data security is immense. Embracing federated learning can lead to a future where healthcare is more efficient, personalised, and secure.
FAQ Section
Q: What is federated learning? A: Federated learning is a machine learning paradigm where a model is trained across multiple decentralised devices or servers holding local data samples without exchanging them. This approach is particularly valuable in healthcare, where data privacy is paramount. Instead of centralising data, federated learning allows institutions to train models while keeping patient data local collaboratively, ensuring compliance with regulations like HIPAA and GDPR.
Q: How does federated learning improve healthcare triage? A: Federated learning improves healthcare triage by enabling collaborative model training across institutions without sharing sensitive data. This leads to more accurate predictive models, real-time monitoring, and personalised medicine, ultimately enhancing patient outcomes and privacy.
Q: What are the challenges in implementing federated learning in healthcare? A: Challenges include data heterogeneity, communication efficiency, and privacy concerns. Solutions involve transferring learning, optimising communication protocols, and implementing differential privacy and secure multi-party computation.
Q: Can federated learning be integrated with IoT devices? A: Federated learning can be integrated with IoT devices to enable real-time monitoring and predictive analytics. This integration enhances patient safety and care quality without compromising data privacy.
Q: How does federated learning enhance data privacy in healthcare? A: Federated learning enhances data privacy by keeping sensitive patient data local to the institutions. This approach ensures that data is not shared or transferred, complying with regulations like HIPAA and GDPR and reducing the risk of data breaches.
Q: How does federated learning impact predictive accuracy in healthcare? A: Federated learning can significantly improve predictive accuracy by training models on diverse datasets from multiple institutions. This collaborative approach leads to more generalisable and accurate models, enhancing patient outcomes.
Q: How does federated learning address data heterogeneity in healthcare? A: Federated learning addresses data heterogeneity through transfer learning and domain adaptation. These methods help bridge the gap between data distributions, ensuring that models trained on diverse data are generalisable.
Q: What are the security concerns in federated learning? A: Security concerns include the risk of malicious actors inferring sensitive information from model updates. Differential privacy and secure multi-party computation can enhance security and prevent data leakage.
Q: How does federated learning benefit real-time monitoring in healthcare? A: Federated learning benefits real-time monitoring by enabling IoT devices to contribute to model training without sharing sensitive data. This integration allows continuous monitoring and predictive analytics, improving patient safety and care quality.
Q: What is the role of federated learning in personalised medicine? A: Federated learning plays a crucial role in personalised medicine by analysing data from various sources to tailor treatments to individual patients. This personalised approach improves outcomes and reduces adverse effects, particularly in oncology and cardiology.
Additional Resources
Journal of Healthcare Informatics Research - Federated Learning for Healthcare Informatics
PMC - Privacy preservation for federated learning in healthcare
ACM Transactions on Computing for Healthcare - Federated Learning for Healthcare Domain - Pipeline, Applications and Challenges
ScienceDirect - Privacy preservation for federated learning in healthcare
Frontiers in Artificial Intelligence - In the Pursuit of Privacy: The Promises and Predicaments of Federated Learning in Healthcare