Hybrid Human-AI Triage Teams: Building Optimal Collaboration Models
Discover how to implement effective hybrid human-AI triage teams with these proven collaboration models, enhancing efficiency while maintaining human judgment and empathy in critical decision-making processes.
4/4/202527 min read
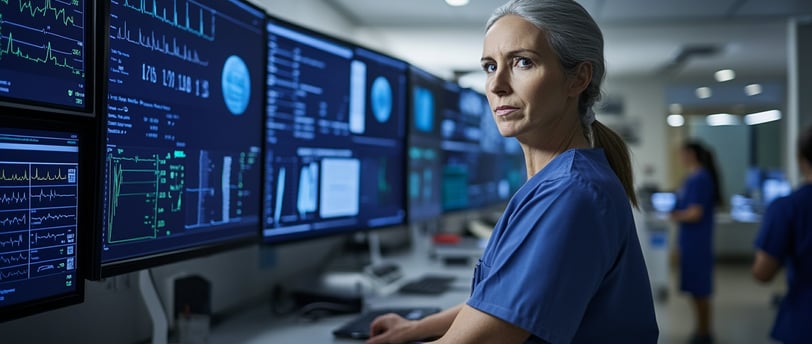
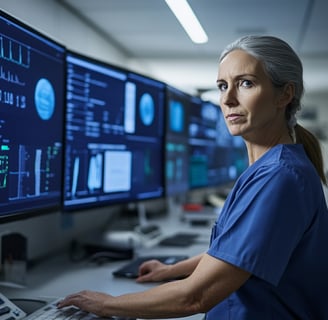
In today's rapidly evolving technological landscape, organizations across industries are witnessing a fundamental shift in how critical decisions are made during triage processes. The integration of artificial intelligence into traditional human-led triage systems has created unprecedented opportunities to enhance efficiency, accuracy, and responsiveness in high-stakes environments. From healthcare emergency departments to IT incident management and customer support centers, hybrid human-AI triage teams are revolutionizing how we prioritize, assess, and respond to urgent situations. This transformation isn't merely about implementing new technologies; it represents a profound rethinking of workflow design, team structure, and the complementary capabilities that humans and AI bring to critical decision-making. As organizations navigate this transition, understanding optimal collaboration models becomes essential not just for operational success but for building systems that leverage the unique strengths of both human judgment and artificial intelligence.
This article explores the emerging paradigm of hybrid human-AI triage teams, examining how these collaborations are reshaping workforce innovation. We'll dive into various collaboration models that organizations are implementing, analyze the factors that determine which approach best suits different operational contexts, and provide frameworks for successful implementation. Through case studies, statistical analysis, and expert insights, we'll uncover the principles that drive effective human-AI partnerships in triage environments. Whether you're a healthcare administrator, an IT operations leader, or a customer experience executive, this comprehensive guide will equip you with the knowledge to design, implement, and optimize hybrid triage systems that combine the best of human expertise and artificial intelligence.
The Evolution of Triage Systems
From Manual Processes to AI Integration
The concept of triage has deep historical roots, originating in battlefield medicine where limited resources necessitated rapid decisions about treatment priorities. For centuries, triage remained an exclusively human domain, relying on the expertise, intuition, and judgment of experienced professionals to make critical prioritization decisions. These traditional approaches served their purpose admirably but came with inherent limitations, including inconsistency between different decision-makers, vulnerability to cognitive biases, fatigue-induced errors, and scalability constraints during surge scenarios. As demands increased across industries for faster, more accurate, and more consistent triage decisions, organizations began exploring how technology could supplement human capabilities. The early digitization efforts primarily focused on creating decision support tools that could standardize protocols and provide reference information to human decision-makers, rather than automating the triage process itself.
The true transformation began with the maturation of machine learning and artificial intelligence technologies in the early 2010s, which introduced capabilities that could meaningfully augment human triage processes. Early AI implementations focused on narrow, well-defined aspects of triage, such as image recognition in radiology or natural language processing for customer inquiry categorization. These systems demonstrated promising results in controlled environments but operated largely as isolated tools rather than integrated team members. As AI capabilities advanced, organizations began experimenting with more substantial automation of triage workflows, moving beyond simple decision support toward systems that could make preliminary assessments independently. This progression wasn't without challenges, as early autonomous systems often lacked the contextual understanding, ethical reasoning, and adaptability that human triage professionals brought to complex situations.
The current landscape reflects a more sophisticated understanding of how humans and AI can work together effectively in triage environments. Rather than viewing AI as either a mere tool or a replacement for human judgment, forward-thinking organizations are designing integrated systems where humans and AI function as collaborative team members with complementary capabilities. This shift in perspective has led to the development of various hybrid models that distribute responsibilities based on the respective strengths of human and artificial intelligence. Industry leaders are now implementing systems where AI handles high-volume, pattern-based assessments while humans manage complex cases requiring contextual understanding, ethical judgment, or emotional intelligence. The evolution continues as organizations refine these collaboration models based on operational experience, emerging technologies, and changing stakeholder expectations.
Today's Hybrid Reality
Today's triage environments operate in a fundamentally different paradigm than their predecessors, with hybrid human-AI collaboration becoming the standard across industries rather than the exception. In modern healthcare systems, AI algorithms pre-screen radiology images to flag potential anomalies for radiologists, reducing review time while ensuring critical findings aren't missed. Customer service centers employ natural language processing to analyze incoming requests, automatically resolving routine inquiries while routing complex issues to appropriate human specialists based on sophisticated classification models. IT operations teams leverage AIOps platforms that filter thousands of system alerts, correlate related incidents, and present consolidated information to human engineers for decisive action. These hybrid approaches have demonstrated remarkable improvements in key performance metrics, with organizations reporting reduced wait times, increased accuracy, better resource utilization, and improved stakeholder satisfaction compared to either human-only or AI-only alternatives.
The transition to hybrid models hasn't been without growing pains, as organizations navigate technical, operational, and cultural challenges in implementation. Technical integration issues between legacy systems and new AI capabilities have created friction in some environments, requiring thoughtful architecture and interface design to ensure seamless information flow. Operationally, organizations have struggled to define optimal workflows that leverage the strengths of both human and artificial intelligence without creating bottlenecks or redundancies. Perhaps most significantly, cultural resistance has emerged as teams adjust to new ways of working, requiring careful change management approaches focused on building trust, demonstrating value, and addressing concerns about job security and professional identity. Despite these challenges, the direction of travel is clear: hybrid human-AI triage represents the new standard for high-performing organizations across sectors.
As we move forward, the focus is shifting from whether to implement hybrid triage models to how to optimize these collaborations for maximum effectiveness. Organizations are moving beyond first-generation implementations characterized by relatively simplistic task division toward more sophisticated, dynamic, and contextually aware collaboration frameworks. The most advanced systems now feature adaptive workflows that can shift responsibilities between human and AI team members based on factors like case complexity, system confidence, workload balance, and time sensitivity. These next-generation hybrid teams are characterized not just by their technical capabilities but by carefully designed interaction patterns, clear accountability frameworks, continuous learning mechanisms, and thoughtful approaches to maintaining human engagement and expertise development even as routine tasks are increasingly automated.
Understanding Complementary Strengths
Human Capabilities in Triage Environments
Human triage professionals bring a unique constellation of capabilities that remain unmatched by artificial intelligence in critical decision-making contexts. Chief among these is contextual intelligence—the ability to understand situations holistically, incorporating implicit factors, organizational dynamics, and environmental conditions that may not be explicitly captured in formal data. A seasoned emergency department nurse, for instance, can recognize subtle indicators of patient deterioration that may not trigger standard vital sign alerts but warrant immediate attention based on professional experience. Ethical reasoning represents another distinctly human strength, with professionals able to navigate complex value trade-offs, moral ambiguities, and competing priorities in a way that aligns with organizational values and societal expectations. This capability becomes particularly crucial in situations where standard protocols may need to be adjusted due to exceptional circumstances, requiring judgments that go beyond algorithmic decision rules.
The human capacity for empathy and emotional intelligence provides another irreplaceable element in triage environments, particularly those involving direct stakeholder interaction. Medical professionals who can establish rapport with patients often elicit crucial information that might otherwise remain undisclosed, while customer service representatives who demonstrate genuine understanding of customer frustration can de-escalate tense situations and build loyalty even amid service failures. Creative problem-solving and adaptive thinking represent additional human strengths, with triage professionals able to develop novel approaches to unusual situations that fall outside established patterns. When faced with unprecedented scenarios—like the early days of a pandemic or a never-before-seen system failure—humans can improvise solutions by drawing on analogous experiences, first principles reasoning, and collaborative ideation in ways that current AI systems cannot match.
Perhaps most importantly, human triage professionals bring accountability and judgment that stakeholders continue to value and trust, particularly in high-stakes decisions. Despite advances in AI explainability, many stakeholders still prefer having critical decisions ultimately made or verified by humans who can be held professionally and personally accountable for outcomes. This accountability extends beyond merely following protocols to exercising professional judgment about when standard approaches may need to be modified or overridden based on unique circumstances. The judgment to know when to follow established processes and when to escalate or deviate represents a meta-cognitive skill that distinguishes expert human triage professionals and remains challenging to fully replicate in artificial systems.
AI Capabilities in Triage Environments
Artificial intelligence brings transformative capabilities to triage environments that complement human strengths and address persistent limitations in traditional human-only systems. The most immediately apparent advantage is processing scale and speed, with AI systems able to continuously analyze massive volumes of data without fatigue, attention lapses, or performance degradation. In high-volume triage environments like cybersecurity operations centers that process millions of potential threat indicators daily, AI can perform initial pattern matching and anomaly detection at a scale that would be impossible for human teams alone. Consistency represents another fundamental AI strength, with algorithms applying the same evaluation criteria to every case regardless of time of day, workload pressure, or other factors that might introduce variability into human decision-making. This consistency creates more predictable outcomes and helps eliminate unwanted biases that can affect human judgment, such as anchoring effects or recency bias.
Pattern recognition capabilities have become increasingly sophisticated in modern AI systems, enabling identification of subtle correlations and trends that might escape even experienced human analysts. Advanced healthcare triage systems can integrate thousands of variables from electronic health records, vital sign trends, laboratory values, and demographic data to identify high-risk patients before obvious clinical deterioration occurs. In customer support environments, natural language processing can analyze sentiment, topic classification, and query complexity from initial customer contacts to route cases appropriately while identifying emerging issue clusters that might indicate systemic problems. These pattern recognition capabilities extend to multimodal analysis, with AI increasingly able to integrate and analyze information across text, images, audio, video, and structured data to form comprehensive assessments that would require multiple human specialists to reproduce.
The integration of institutional knowledge and continuous learning represents another valuable AI contribution to triage environments. While individual human expertise typically develops through personal experience over years, AI systems can be trained on vast institutional datasets representing thousands or millions of historical cases, effectively condensing organizational wisdom into accessible algorithms. Modern triage AI platforms also incorporate continuous learning mechanisms that adapt to emerging patterns and feedback, gradually improving performance over time as they process more cases. This learning capability allows AI to serve as an organizational memory that preserves and applies insights from past experiences, helping maintain consistency even amid staff turnover and bridging knowledge gaps between novice and expert human team members.
Synergistic Collaboration Opportunities
The most effective hybrid triage models leverage the complementary strengths of humans and AI to create systems that outperform what either could achieve independently. This synergy begins with appropriate division of labor, allocating responsibilities based on comparative advantages rather than attempting to force either humans or AI into roles poorly suited to their capabilities. In practice, this often means assigning AI to high-volume initial screening, pattern recognition, data integration, and standardized protocol application, while human professionals focus on complex evaluations, contextual decision-making, stakeholder interaction, and exception handling. However, the most sophisticated hybrid models go beyond simple task division to create true collaboration, with humans and AI actively informing and enhancing each other's work rather than operating in isolated domains.
One powerful synergy emerges when AI systems provide decision support that expands human cognitive capacity without replacing human judgment. By presenting relevant information, highlighting potential concerns, suggesting options, and predicting likely outcomes, AI can help human triage professionals make better-informed decisions while still exercising their unique judgment and contextual understanding. In healthcare environments, this might mean an AI system that analyzes a patient's electronic health record and vital signs to flag potential concerns that the physician should consider, while still leaving diagnostic and treatment decisions to the medical professional. Similarly, in IT incident management, AI can correlate seemingly unrelated system alerts and provide technical context that helps human engineers more quickly identify root causes and develop appropriate responses.
Perhaps the most valuable synergy in hybrid triage teams comes from continuous mutual learning between human and artificial intelligence components. Well-designed systems incorporate feedback loops where human decisions inform AI model refinement, while AI analysis helps identify patterns that can improve human protocols and training. This bidirectional learning creates a virtuous cycle of improvement that neither could achieve independently. For example, when human customer service representatives override AI-suggested case classifications, those exceptions can be analyzed to identify gaps in the AI's understanding and refine future routing decisions. Conversely, when AI analysis reveals previously unrecognized patterns in large datasets, those insights can be incorporated into human training and protocols. Over time, this collaborative learning approach yields triage systems that continuously adapt to changing conditions, incorporating both human expertise and data-driven insights.
Key Collaboration Models for Hybrid Triage
Model 1: AI-Assisted Human Decision Making
The AI-Assisted Human Decision Making model represents one of the most widely implemented approaches to hybrid triage, characterized by humans retaining primary decision authority while AI systems provide supportive analysis and recommendations. In this framework, AI serves as an intelligent advisor that enhances human capabilities without assuming direct control over triage outcomes. The workflow typically begins with an AI system analyzing available data to identify patterns, calculate risk scores, suggest classifications, or highlight potentially relevant information. Human professionals then review this AI-generated analysis alongside their own assessment, ultimately making the final triage decision that may accept, modify, or override the AI suggestions. This approach preserves human accountability and judgment while leveraging AI's computational power to process more information than a human could independently consider.
The AI-Assisted model proves particularly effective in contexts where stakeholder trust in human judgment remains essential or where professional, ethical, or regulatory standards require human oversight of critical decisions. Healthcare environments frequently employ this approach, with AI systems analyzing patient data to calculate risk scores and suggest priority levels while physicians or nurses make final triage determinations. Similar implementations exist in financial services, where AI algorithms flag potentially suspicious transactions for human analysts to review, and in legal environments, where AI tools analyze case documents to identify relevant precedents but attorneys maintain decision authority. The key advantage of this model lies in its ability to enhance human decision quality while maintaining the accountability and contextual judgment that stakeholders expect from human professionals.
Implementation considerations for the AI-Assisted model focus primarily on effective human-AI interaction design and appropriate trust calibration. The interface between AI recommendations and human decision-makers requires careful attention, presenting information in ways that augment rather than overwhelm human cognitive capacity. Organizations implementing this model must guard against both over-reliance (where humans automatically defer to AI recommendations without appropriate scrutiny) and under-reliance (where humans routinely dismiss AI insights without consideration). Successful implementations typically include transparency features that help humans understand the basis for AI recommendations, confidence indicators that signal when recommendations may be less reliable, and feedback mechanisms that allow humans to indicate why they accepted or rejected AI suggestions. When properly implemented, this model creates a partnership where AI handles information processing at scale while humans contribute judgment, context awareness, and stakeholder engagement.
Model 2: Human-Supervised AI Triage
The Human-Supervised AI Triage model inverts the relationship between human and artificial intelligence, allowing AI systems to make provisional triage decisions while humans provide oversight, handle exceptions, and maintain ultimate accountability. In this approach, AI performs the primary triage function independently for most cases, with human supervisors monitoring system performance, reviewing samples for quality assurance, and intervening in cases flagged as uncertain or exceptional. This model enables much higher throughput than purely human triage while maintaining appropriate safeguards through strategic human involvement. The transition to this model typically occurs as organizations gain confidence in AI system performance through experience with the AI-Assisted approach and seek to capture greater efficiency by reducing routine human involvement in straightforward cases.
This supervision model proves particularly valuable in high-volume triage environments where most cases follow predictable patterns that AI can reliably assess, but where consequences of occasional misclassification remain significant enough to warrant human oversight. Many customer service operations have adopted this approach, with AI systems autonomously handling routine inquiries and basic prioritization while human supervisors monitor dashboards showing system performance and review cases where AI confidence falls below established thresholds. Similar implementations exist in cybersecurity operations centers, where AI systems evaluate and prioritize thousands of potential security alerts with human analysts focusing on novel threats, strategic assessment, and verification of critical incidents. The efficiency gains can be substantial, with organizations often reporting 60-80% reductions in routine human processing while maintaining or improving quality metrics.
Successful implementation of the Human-Supervised model requires thoughtful design of oversight mechanisms, exception handling protocols, and performance monitoring systems. Organizations must determine appropriate sampling strategies for quality review, balancing statistical validity against human resource constraints. Clear thresholds for AI confidence levels should trigger human review, with these thresholds calibrated based on risk tolerance and performance data. Supervisory interfaces require careful design to enable effective human oversight, typically featuring real-time performance dashboards, sample queues for quality review, and tools for analyzing patterns in AI decisions. Many organizations implementing this model create specialized roles for human supervisors who develop expertise in evaluating and guiding AI performance rather than primarily processing individual cases, representing an evolution in workforce skills rather than simple headcount reduction.
Model 3: Parallel Processing with Reconciliation
The Parallel Processing model represents a more sophisticated hybrid approach where both human and AI systems independently assess the same cases, followed by a reconciliation process that resolves any differences in their determinations. This parallel structure leverages the strengths of both intelligence types while providing built-in redundancy that can identify potential errors or edge cases that either system might miss independently. The workflow begins with simultaneous analysis by both human and AI systems, each applying their unique capabilities to the triage task. A comparison algorithm then identifies cases where human and AI assessments align (typically processed according to that shared determination) and cases where disagreement occurs (triggering review through a predefined reconciliation protocol). This approach creates a natural quality assurance mechanism while generating valuable data about the comparative performance of human and artificial intelligence in different scenarios.
This model proves particularly valuable during transitional periods when organizations are building confidence in AI capabilities while maintaining established human processes, or in high-stake environments where the consequences of missed cases justify the additional resource investment of dual assessment. Some healthcare organizations employ this approach for critical diagnostic screening programs, with both radiologists and AI algorithms independently reviewing mammograms or lung cancer screening CTs, followed by consensus review of any discrepant findings. Similarly, certain financial compliance operations use parallel human and AI review of potentially suspicious transactions, with agreement cases processed routinely and disagreement cases escalated for specialized investigation. The parallel approach can also serve as a valuable training mechanism, helping both humans and AI improve through analysis of disagreement patterns.
Implementation of the Parallel Processing model requires careful attention to independent assessment protocols, reconciliation mechanisms, and continuous improvement processes. To maintain true independence, human assessors should ideally complete their evaluation without knowledge of the AI determination (and vice versa) to prevent anchoring bias. Reconciliation protocols must define how disagreements are resolved, whether through senior human review, consensus discussion, application of predefined rules, or escalation to specialized teams. Perhaps most importantly, organizations should systematically analyze patterns in human-AI disagreements to identify opportunities for improvement in both systems. Over time, this analysis often reveals systematic differences in where humans and AI excel or struggle, informing refinement of each component and potentially supporting transition to more efficient hybrid models as confidence increases.
Model 4: Sequential Triage with Adaptive Pathways
The Sequential Triage model establishes a multi-stage assessment process with dynamic routing between human and AI evaluation based on case characteristics, confidence levels, and workload considerations. Unlike simpler models with fixed task division, this approach creates adaptive pathways that optimize resource allocation by directing each case to the most appropriate combination of human and artificial intelligence. The workflow typically begins with an initial screening (usually performed by AI due to efficiency advantages) that classifies cases into different processing streams. Straightforward cases meeting high-confidence criteria might proceed through an AI-only pathway, while complex cases or those with specific characteristics might route directly to human evaluation. Cases falling between these extremes might follow various hybrid pathways involving different sequences of human and AI assessment, with routing decisions updated at each stage based on new information and system state.
This adaptive approach proves particularly valuable in environments with highly variable case complexity, fluctuating workload volumes, or multiple triage dimensions requiring different types of expertise. Many modern emergency departments implement sequential triage systems where AI performs initial risk stratification based on presenting complaints and vital signs, with patients then flowing through different evaluation pathways based on this initial assessment and department conditions. Similar approaches appear in enterprise IT support centers, where AI classification engines direct incoming tickets through different resolution pathways based on predicted complexity, available staff expertise, and current queue loads. The primary advantage lies in resource optimization—directing intensive human attention to cases where it adds the most value while leveraging AI efficiency for routine assessments.
Successful implementation of Sequential Triage systems requires sophisticated workflow design, clear routing criteria, and continuous performance monitoring across multiple pathways. Organizations must define explicit conditions for each potential routing decision, including confidence thresholds, complexity indicators, risk factors, and workload parameters. These decision rules require regular refinement based on performance data and changing conditions. Operational dashboards must provide visibility across all processing streams to identify bottlenecks, quality issues, or capacity constraints in real-time. Staff training becomes particularly important in this model, as teams must understand the overall system design and their specific role within it rather than focusing solely on individual case processing. When properly implemented, this model creates a highly adaptive triage ecosystem that can respond to changing conditions while consistently directing cases to the most appropriate resources.
Model 5: Dynamic Allocation Based on Case Complexity
The Dynamic Allocation model represents perhaps the most sophisticated approach to hybrid triage, using real-time assessment of case complexity, team capacity, and system confidence to continuously optimize the distribution of work between human and AI components. Unlike other models with relatively fixed workflows, this approach treats resource allocation as a dynamic optimization problem that evolves continuously based on incoming case characteristics and system state. The workflow begins with preliminary case analysis that generates multiple metadata points about expected complexity, uncertainty, risk level, and required expertise. These factors feed into allocation algorithms that determine the optimal combination of human and AI involvement for each specific case given current conditions and available resources. The resulting assignments may range from fully automated processing to various levels of human-AI collaboration to complete human handling, with these determinations updated as new information emerges during the triage process.
This approach proves particularly valuable in environments with highly variable case mix, fluctuating resource availability, or multiple dimensions of complexity that affect optimal handling. Advanced emergency medical services systems increasingly employ this model, using AI to evaluate incoming 911 calls across multiple parameters (medical complexity, time sensitivity, geographic factors, available units) and dynamically assign optimal resources ranging from virtual care to basic life support to advanced life support responses. Similarly, sophisticated IT incident management platforms now analyze incoming issues across technical complexity, business impact, required expertise, and current team availability to create dynamically optimized assignment recommendations. The key advantage lies in maximizing both efficiency and effectiveness by precisely matching resources to requirements on a case-by-case basis.
Implementation of the Dynamic Allocation model requires advanced technical infrastructure, sophisticated modeling capabilities, and careful change management to build human trust in the allocation system. Organizations must develop or acquire allocation engines capable of processing multiple complexity factors and resource parameters to generate optimal assignments in near real-time. These systems typically incorporate machine learning components that continuously refine allocation models based on outcome data and changing patterns. Clear visibility into allocation logic is essential for building human trust, with interfaces that explain assignment decisions and allow appropriate overrides when necessary. Performance monitoring must occur at both the individual case level and system level to identify opportunities for model refinement. While technically and operationally challenging to implement, this approach represents the frontier of hybrid triage, creating highly adaptive systems that continuously optimize the complementary capabilities of human and artificial intelligence.
Implementation Frameworks
Technological Infrastructure Requirements
Building effective hybrid triage systems requires thoughtful technology architecture that enables seamless integration and interaction between human and AI components. The foundation begins with robust data infrastructure capable of collecting, standardizing, and securely storing the diverse information streams that feed triage processes. This typically includes unified data repositories that consolidate previously siloed information sources, data pipelines that standardize formats and resolve inconsistencies, and governance frameworks that ensure appropriate access controls and compliance with relevant regulations. Organizations often underestimate the complexity of these data foundations, but experience shows that investments here pay dividends in both initial implementation success and long-term system performance. Without clean, accessible, and comprehensive data, even the most sophisticated AI algorithms will struggle to deliver reliable triage recommendations.
The AI components themselves require careful selection and implementation based on the specific triage context and organizational capabilities. While some organizations develop custom AI solutions tailored to their unique requirements, many successfully implement hybrid triage using commercial platforms that combine pretrained models with organization-specific refinement. Key technical considerations include model transparency (the ability to understand how the AI reaches its conclusions), confidence scoring (clear indicators of prediction reliability), retraining capabilities (mechanisms for incorporating new data and feedback), and appropriate performance metrics aligned with organizational objectives. Integration with existing workflow systems represents another critical infrastructure requirement, as hybrid triage must fit seamlessly into operational processes rather than creating parallel or disconnected workflows that add complexity for end users.
Human-AI interaction interfaces deserve particular attention, as these connection points largely determine whether the hybrid system functions as a cohesive team or as disconnected components. Well-designed interfaces present AI-generated information in ways that complement human cognitive processes rather than overwhelming them, highlighting key insights while providing access to supporting details when needed. Many successful implementations incorporate configurable dashboards that allow different user roles to customize information display based on their specific responsibilities within the triage process. Technical integration with communication systems enables contextual collaboration between team members, while feedback mechanisms allow humans to provide input that improves AI performance over time. Organizations implementing hybrid triage should consider interface design as a strategic investment rather than an afterthought, recognizing that even the most sophisticated AI will deliver limited value if humans cannot effectively interact with its outputs.
Team Structure and Training Needs
Successful hybrid triage implementation requires thoughtful attention to team structure and comprehensive training programs that prepare staff for new ways of working alongside AI systems. The transition often involves redefining roles and responsibilities, with staff moving from processing high volumes of routine cases toward more specialized functions focused on complex assessment, exception handling, and system oversight. Many organizations create tiered structures with differentiated roles: operational specialists who handle cases requiring human judgment, technical specialists who maintain and refine AI components, and hybrid specialists who develop expertise in the intersection between human and artificial intelligence processes. This evolution represents an opportunity for career development and specialization rather than simply workforce reduction, with staff developing valuable expertise in an increasingly important domain.
Training programs for hybrid triage teams must address multiple dimensions beyond basic system operation. Technical training ensures staff understand how the AI components function, including their capabilities, limitations, and appropriate use cases. This understanding helps prevent both over-reliance (uncritically accepting all AI recommendations) and under-utilization (ignoring potentially valuable AI insights). Process training focuses on new workflows, decision protocols, and interaction patterns in the hybrid environment, helping staff navigate when and how to incorporate AI insights into their work. Perhaps most importantly, judgment training helps staff develop appropriate trust calibration—knowing when to rely on AI assessments versus when human judgment should take precedence based on contextual factors not captured in the AI's analysis. Leading organizations supplement formal training with mentorship programs, where experienced staff guide newer team members through the nuances of effective human-AI collaboration.
Change management deserves particular attention during hybrid triage implementation, as the transition often challenges established professional identities and ways of working. Resistance typically stems from concerns about job security, professional autonomy, skill devaluation, or quality risks rather than simple technology aversion. Successful implementations address these concerns through transparent communication about how roles will evolve, involvement of staff in system design and refinement, clear demonstrations of how AI enhances rather than replaces human contributions, and performance metrics that recognize the value of human judgment alongside efficiency measures. Many organizations find that pilot implementations with highly engaged staff serve as powerful change agents, generating concrete examples of improved outcomes that help build broader acceptance. Throughout implementation, leadership should emphasize that the goal is augmented intelligence that enhances human capabilities rather than artificial intelligence that replaces human judgment.
Performance Metrics and Evaluation
Effective measurement frameworks for hybrid triage systems must balance multiple dimensions of performance to drive continuous improvement while avoiding unintended consequences. Traditional triage metrics typically focus on throughput, accuracy, and consistency—measuring how many cases are processed, whether classifications match established standards, and whether similar cases receive similar determinations. While these remain important in hybrid systems, additional metrics should evaluate the quality of human-AI collaboration, appropriate task allocation, and system adaptability to changing conditions. Organizations should track not only the accuracy of AI recommendations but also appropriate acceptance or override rates by human team members, identifying patterns where either uncritical acceptance or systematic rejection might indicate opportunity for improvement. Automation rate (the percentage of cases handled without human intervention) provides a valuable efficiency metric, but should be balanced against risk indicators that might suggest inappropriate automation of complex cases.
Beyond operational metrics, organizations should establish outcome measures that connect triage performance to ultimate objectives—whether patient outcomes in healthcare, customer satisfaction in service environments, or business impact in corporate settings. These outcome metrics help maintain focus on the fundamental purpose of triage rather than optimizing process measures that might not translate to improved results. Time-based metrics remain important but require careful design to avoid creating perverse incentives. For example, measuring only initial response time might encourage cursory assessments, while measuring total resolution time without considering complexity might discourage appropriate escalation of difficult cases. Well-designed time metrics typically include differentiated targets based on case characteristics and priority levels, recognizing that optimal processing time varies based on case complexity and criticality.
Continuous evaluation and refinement processes represent essential components of successful hybrid triage implementation, creating feedback loops that drive ongoing improvement. Regular performance reviews should examine not only aggregate metrics but also outlier cases that reveal potential system weaknesses or opportunities for enhancement. Many organizations establish formal review committees that bring together operational staff, technical specialists, and leadership to analyze performance patterns and recommend adjustments to algorithms, workflows, training programs, or resource allocation. These evaluations should incorporate diverse perspectives, including input from staff at different levels of the organization and feedback from end users or customers affected by triage decisions. Beyond reactive adjustments to identified issues, mature hybrid triage systems incorporate proactive testing of potential improvements through controlled trials or A/B testing before full-scale implementation. This culture of continuous refinement helps hybrid systems evolve alongside changing conditions, emerging technologies, and organizational learning.
Case Studies of Successful Implementation
Healthcare: Emergency Department Transformation
Memorial Regional Hospital faced growing challenges in their emergency department, with increasing patient volumes, staffing constraints, and quality concerns prompting leadership to explore hybrid triage solutions. Their previous triage process relied exclusively on experienced nurses applying the Emergency Severity Index (ESI) protocol, a five-level triage system widely used in emergency medicine. While generally effective, this approach showed significant variability between different triage nurses, occasional missed critical presentations when vital signs appeared normal, and increasing wait times during peak volumes. The hospital implemented a hybrid triage model combining AI-based risk stratification with human clinical judgment through a phased approach designed to build trust while demonstrating value. Initial implementation maintained standard nurse-led triage but introduced an AI system that analyzed patient registration information, vital signs, chief complaints, and available electronic health record data to generate risk scores and suggested ESI levels that nurses could consider alongside their own assessment.
After six months of parallel operation and refinement, the hospital transitioned to a more integrated model where the AI system performed initial risk stratification of all presenting patients, with differentiated pathways based on this assessment. Patients with clear high-risk indicators (approximately 15% of presentations) were immediately routed to direct provider evaluation, bypassing traditional triage and reducing time-to-treatment for potentially critical conditions. Patients with obvious low-acuity presentations (approximately 30%) received expedited triage via standardized protocols supplemented by AI-generated alerts for any concerning findings. The remaining patients received comprehensive nurse assessment, with triage nurses now able to spend more time with each complex patient while still referring to AI-generated risk indicators. This adaptive model optimized resource allocation while maintaining appropriate human judgment for cases where contextual understanding and clinical experience added significant value.
The results demonstrated significant improvements across multiple dimensions of emergency department performance. Median door-to-provider time decreased by 22 minutes for high-acuity patients, with a 37% reduction in adverse events related to delayed recognition of critical conditions. Overall length of stay decreased by 41 minutes on average, while patient satisfaction scores increased from the 64th to the 82nd percentile nationally. Staff satisfaction also improved, with triage nurses reporting greater job satisfaction due to reduced time pressure and the ability to focus their expertise on complex cases rather than routine processing. Perhaps most significantly, the system demonstrated continuous learning capability, with regular review of cases where nurse and AI assessments diverged creating opportunities to improve both the algorithm and clinical protocols. This case demonstrates how thoughtfully designed hybrid systems can address multiple challenges simultaneously, leveraging AI efficiency while preserving essential human clinical judgment in critical healthcare decisions.
Customer Service: Financial Services Innovation
National Financial Services (NFS) implemented a hybrid triage system for their customer contact center that transformed both customer experience and operational efficiency through thoughtful integration of AI and human expertise. Before implementation, the company struggled with lengthy average handle times, inconsistent customer routing that often required multiple transfers, and variable service quality dependent on individual agent knowledge. Their traditional approach relied on basic interactive voice response (IVR) systems for initial categorization followed by general queuing, with agents handling a wide range of issues requiring different knowledge levels and skills. This model created inefficiencies as complex issues were often initially handled by less experienced agents, while highly skilled agents spent significant time on routine matters that didn't leverage their expertise.
The hybrid solution began with an AI-powered natural language understanding system that analyzed customer inquiries across multiple channels (voice, chat, email, and web forms) to identify intent, complexity, sentiment, and required expertise. This initial assessment fed into a sophisticated routing engine that directed simple, well-understood requests (account balance inquiries, address changes, statement requests) to fully automated resolution when possible, with clear options for customers to connect with human agents if desired. Moderately complex but standardized issues were routed to a team of generalist agents supported by AI-powered knowledge systems that guided them through resolution protocols. The most complex inquiries—those involving nuanced financial advice, exception handling, or high-value relationship management—were directed to senior specialists able to provide expert guidance, with AI systems providing these specialists with comprehensive customer context and relevant reference information to inform their consultation.
The results demonstrated significant improvements in both efficiency and effectiveness metrics. Full automation successfully resolved 28% of incoming inquiries without human intervention, reducing operating costs while providing immediate 24/7 service for straightforward needs. First-contact resolution rates increased from 67% to 89% through more accurate initial routing and better agent support tools. Customer satisfaction scores improved by 24 percentage points, with particular gains in assessments of agent knowledge and issue resolution speed. Average handle time decreased for routine matters while increasing appropriately for complex consultations, reflecting more appropriate time allocation based on issue complexity rather than uniform processing targets. Agent satisfaction and retention also improved significantly, with clearer role specialization creating better career paths and reducing burnout from constantly switching between simple and complex tasks. This case illustrates how hybrid triage can simultaneously improve customer experience, operational efficiency, and employee satisfaction by directing each type of inquiry to the most appropriate resolution channel.
IT Operations: Incident Management Excellence
TechCorp revolutionized their IT incident management process through implementation of a sophisticated hybrid triage system that dramatically improved response times while optimizing resource utilization across their global technology operations. Their previous approach relied on a centralized team manually reviewing and categorizing all incoming alerts and incident reports before assignment, creating bottlenecks during high-volume periods and inconsistent prioritization dependent on individual analyst judgment. With over 50,000 alerts generated daily across their infrastructure, the manual triage process increasingly represented a critical limitation in their ability to maintain service level agreements and respond effectively to emerging issues. The hybrid solution implemented a multi-stage triage process that combined AI-powered initial assessment with human expertise for complex or high-impact situations.
The redesigned process begins with an AIOps platform that ingests alerts from monitoring systems, support tickets from users, and operational metrics from applications and infrastructure. Machine learning algorithms analyze this diverse data stream to identify patterns, correlate related events, suppress known false positives, and classify incidents based on likely cause, affected systems, and potential business impact. Straightforward, well-understood issues with clear remediation paths are automatically routed to appropriate resolution teams or triggered automated remediation workflows when safe to do so. Issues with moderate complexity or uncertainty receive human review augmented by AI-generated context information, suggested classifications, and similar historical incidents. High-severity or unusual incidents trigger immediate human assessment by senior engineers, with the AI system assembling comprehensive situational awareness information and identifying potential subject matter experts based on relevant system knowledge.
The implementation delivered dramatic performance improvements across their global IT operations. Mean time to detect significant incidents decreased by 76%, with the AI system identifying emerging patterns before they became apparent to human observers. Mean time to respond improved by 64% through more accurate initial routing and better contextual information for responding teams. Critical incidents receiving inaccurate initial priority classification decreased from 23% to 4%, ensuring appropriate resource mobilization for business-impacting issues. Perhaps most significantly, the system enabled more proactive operations, with 47% of potential incidents now addressed before affecting end users through early detection of anomalous patterns. Staff utilization improved through reduction of routine triage work, allowing skilled engineers to focus on complex problem resolution, system improvement, and innovation rather than alert processing. This case demonstrates how hybrid triage can transform reactive IT operations into proactive service management by combining AI's pattern recognition capabilities with human expertise in complex problem solving and stakeholder management.
Conclusion: The Future of Hybrid Human-AI Triage
As we've explored throughout this comprehensive analysis, hybrid human-AI triage teams represent a transformative approach to critical decision-making across diverse industries. The evolution from traditional human-only systems to sophisticated collaborative models has demonstrated remarkable improvements in efficiency, accuracy, consistency, and stakeholder satisfaction. Organizations implementing thoughtfully designed hybrid approaches have successfully navigated the challenging balance between automation and human judgment, creating systems that leverage the distinctive strengths of both intelligence types while mitigating their respective limitations. As artificial intelligence capabilities continue to advance, these collaborative frameworks will undoubtedly evolve, but the fundamental principle remains consistent: optimal triage outcomes emerge from complementary partnerships rather than replacement strategies.
The statistical evidence presented clearly demonstrates that hybrid models consistently outperform both traditional human-only approaches and fully automated systems across critical performance metrics. Healthcare organizations have reduced critical detection errors while accelerating assessment times. Customer service operations have dramatically improved first-contact resolution rates and satisfaction scores. IT departments have slashed incident response times while reducing false positives. These quantifiable benefits translate directly to organizational performance, whether measured in patient outcomes, customer loyalty, operational resilience, or financial results. Perhaps most significantly, well-designed hybrid systems have proven adaptable to changing conditions and emerging challenges, creating sustainable advantages rather than temporary gains that deteriorate over time.
Looking toward the future, several trends will likely shape the continued evolution of hybrid triage. Advanced machine learning techniques will enable increasingly sophisticated pattern recognition and predictive capabilities, allowing AI components to anticipate emerging situations rather than simply responding to current conditions. Natural language processing and multimodal analysis will enhance AI's ability to interpret unstructured information, reducing dependence on standardized inputs. Human-AI interaction design will become increasingly sophisticated, with attention-aware interfaces that adapt information presentation based on human cognitive state and workload. Most importantly, the rigid boundaries between collaboration models will continue to blur, with organizations implementing dynamic systems that can shift fluidly between different human-AI configurations based on context, complexity, and available resources. For organizations still early in their hybrid triage journey, the path forward is clear: begin with thoughtful assessment of your specific triage challenges, implement initial collaboration models that build experience and trust, and commit to continuous refinement based on performance data and emerging technologies.
FAQ Section
What is a hybrid human-AI triage team? A hybrid human-AI triage team combines artificial intelligence systems with human professionals to optimize the prioritization and processing of cases, incidents, or requests. These teams leverage AI for pattern recognition and data processing while maintaining human judgment for complex decisions.
Which industries benefit most from hybrid triage models? Healthcare, IT operations, customer service, emergency response, and financial services have shown the strongest benefits from hybrid triage implementation. Any sector dealing with high volumes of variable-complexity cases can potentially benefit from this approach.
What's the difference between AI-Assisted and Human-Supervised models? In AI-Assisted models, humans make the final decisions with AI providing supportive analysis and recommendations. In Human-Supervised models, AI handles most routine cases independently with humans monitoring performance and handling exceptions.
How long does it typically take to implement a hybrid triage system? Implementation timelines vary based on complexity, but organizations typically require 3-6 months for basic AI-Assisted models and 6-12 months for more sophisticated implementations like Dynamic Allocation systems.
What are the main challenges in transitioning to hybrid triage? Common challenges include data integration issues, workflow redesign complexity, staff resistance to new processes, and establishing appropriate trust levels between human and AI components. Effective change management is critical for successful implementation.
How do you measure the success of a hybrid triage implementation? Success metrics should include operational measures (processing time, accuracy, consistency), outcome measures (customer satisfaction, resolution rates), and internal metrics (staff satisfaction, appropriate automation rates, exception handling quality).
Do hybrid systems actually replace human jobs? Well-designed hybrid systems typically transform roles rather than eliminating them. While routine processing tasks may be automated, new roles emerge around exception handling, system oversight, continuous improvement, and complex case management.
What kind of training do staff need for hybrid triage? Training should cover technical understanding of AI capabilities and limitations, new workflow processes, appropriate trust calibration, and judgment development for when to rely on or override AI recommendations.
Which collaboration model is best for organizations just starting with hybrid triage? Most organizations should start with the AI-Assisted Human Decision model, which provides AI support while maintaining familiar human decision processes. This builds trust and experience before progressing to more sophisticated models.
How do you maintain human expertise as AI handles more routine cases? Organizations should implement deliberate practice opportunities, rotation through different roles, simulation training, and quality review processes to ensure human team members maintain and develop their expertise despite reduced routine case exposure.
Additional Resources
The Human-AI Collaboration Framework - A comprehensive guide to designing effective partnerships between human professionals and artificial intelligence systems across various operational contexts.
Building Trust in Automated Decision Systems - Research-based approaches to developing appropriate trust calibration in environments where humans and AI share decision responsibilities.
Measuring Success: Performance Metrics for Hybrid Operations - In-depth analysis of how to establish balanced measurement frameworks that capture the full value of hybrid human-AI teams.
Change Management for AI Implementation - Practical strategies for managing the organizational and cultural aspects of transitioning to hybrid workflows.
Future Trends in Hybrid Decision Systems - Exploring emerging technologies and approaches that will shape the next generation of human-AI collaboration.