How Voice-First AI is Humanising Digital Triage
Discover how voice-first AI technology is transforming healthcare triage, creating more empathetic, accessible patient experiences while reducing clinical burden and improving outcomes.
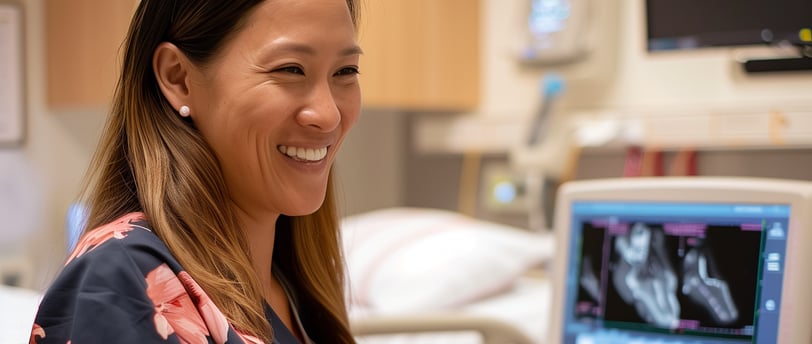
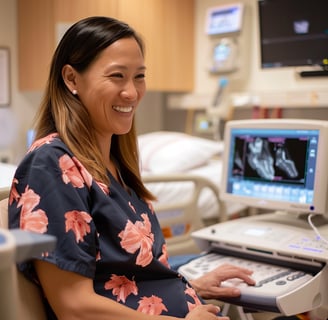
Imagine calling your healthcare provider about worsening symptoms and instead of navigating a frustrating maze of button-pressing or waiting endlessly on hold, you're greeted by a conversational AI that understands your concerns, asks relevant follow-up questions, and helps determine your next steps—all through natural speech. This isn't a distant future scenario; it's the emerging reality of voice-first AI in healthcare triage. The traditional patient journey, often fraught with communication barriers, lengthy wait times, and impersonal interactions, is undergoing a profound transformation. Voice-first AI technology is at the forefront of this revolution, fundamentally changing how patients access care and how providers deliver it. By leveraging advanced natural language processing and machine learning, these intelligent systems are creating more human-centered experiences while simultaneously addressing critical healthcare challenges such as clinician burnout, access disparities, and operational inefficiencies. Throughout this article, we'll explore how voice-first AI is humanizing digital triage, enhancing patient satisfaction, improving clinical outcomes, and reshaping the healthcare landscape for the better.
The Evolution of Digital Triage in Healthcare
Healthcare triage has come a long way from purely manual processes conducted via telephone or in-person assessments. The first generation of digital triage solutions emerged in the early 2000s, primarily consisting of simple symptom checkers and basic online questionnaires that offered limited guidance based on fixed decision trees. These early systems, while innovative for their time, often frustrated patients with their rigidity and inability to understand nuance or context. As healthcare organizations sought to improve efficiency and reduce unnecessary visits, second-generation solutions incorporated more sophisticated algorithms and began integrating with electronic health records, yet still relied heavily on text-based interfaces that many patients—particularly older adults, those with disabilities, or individuals with limited digital literacy—found challenging to navigate.
The COVID-19 pandemic served as a catalyst for accelerating digital transformation across healthcare, with virtual care adoption skyrocketing out of necessity. During this period, the limitations of existing digital triage became glaringly apparent, as healthcare systems struggled to efficiently screen and direct the surge of concerned patients. Many digital solutions failed to provide the reassurance and human connection that anxious patients needed during a time of crisis. This gap between technological capability and human needs highlighted the importance of creating more intuitive, compassionate digital interfaces.
Enter voice-first AI triage—the third and most sophisticated generation of digital triage solutions. Unlike its predecessors, voice-first technology prioritizes natural conversation as the primary mode of interaction, allowing patients to describe their symptoms and concerns just as they would to a human clinician. These systems leverage advanced natural language processing, contextual understanding, and emotional intelligence to create experiences that feel remarkably human. By removing the barriers of complex user interfaces and technical language, voice-first AI makes healthcare triage more accessible to diverse patient populations while simultaneously collecting more comprehensive clinical information.
The evolution toward voice-first approaches represents a fundamental shift in healthcare technology philosophy—moving from systems designed around technical limitations to solutions built around human needs and natural communication patterns. This transition aligns with the broader industry movement toward patient-centered care models that prioritize individual preferences, accessibility, and emotional well-being alongside clinical outcomes. As we continue this evolutionary journey, voice-first AI stands as a bridge between high-tech healthcare delivery and the deeply human experience of seeking care when vulnerable.
Understanding Voice-First AI Technology
Voice-first AI represents a sophisticated convergence of several cutting-edge technologies working in harmony to create intuitive healthcare interactions. At its core, these systems rely on advanced natural language processing (NLP) capabilities that have evolved dramatically in recent years. Modern NLP allows AI systems to understand not just the literal meaning of words but also context, intent, and even emotional nuances in a patient's speech. This understanding goes far beyond keyword recognition, enabling the system to interpret complex symptom descriptions, vague complaints, and the kind of narrative storytelling that patients naturally use when describing health concerns.
Complementing the language understanding components are sophisticated clinical decision support algorithms that evaluate patient inputs against evidence-based protocols and medical knowledge bases. These algorithms help determine the appropriate level of care urgency, potential diagnoses to consider, and recommended next steps. Unlike earlier rules-based systems, today's voice-first platforms employ machine learning techniques that continuously improve their accuracy and relevance based on outcomes data. Many leading solutions now incorporate feedback loops where clinician assessments help refine the AI's understanding, creating a system that becomes increasingly precise over time.
The voice interface itself leverages advances in speech recognition technology that can accurately transcribe a wide range of accents, dialects, and speech patterns with remarkably low error rates. Beyond mere transcription, these systems analyze vocal biomarkers such as tone, cadence, breathing patterns, and other subtle characteristics that can provide additional clinical insights. For instance, research has demonstrated that voice analysis can detect cognitive impairment, respiratory conditions, depression, and other health states that might not be explicitly stated by the patient but are reflected in their speech patterns.
Behind the scenes, voice-first AI triage platforms maintain sophisticated integration capabilities with existing healthcare IT infrastructure. This includes secure connections to electronic health records (EHRs), appointment scheduling systems, telehealth platforms, and provider communication tools. These integrations ensure that the information collected during the AI interaction seamlessly flows into the appropriate clinical workflows, avoiding redundant data entry and providing clinicians with a comprehensive picture of the patient's situation before direct human interaction occurs.
Privacy and security considerations are paramount in voice-first healthcare applications, with systems employing end-to-end encryption, secure cloud architectures, and rigorous compliance with healthcare data protection regulations such as HIPAA. Many platforms now offer multi-factor authentication options and maintain detailed audit trails of all interactions to ensure appropriate data governance. As these technologies mature, the technical sophistication remains largely invisible to the patient, who experiences only a natural, conversational interface that listens, understands, and guides them through their healthcare journey.
The Human Element: How Voice AI Creates Empathetic Interactions
The concept of empathy in healthcare technology might initially seem paradoxical—how can artificial intelligence demonstrate genuine understanding of human suffering? Yet voice-first AI is remarkably effective at creating interactions that patients perceive as empathetic and supportive. This perception stems from several key design elements that prioritize the emotional aspects of healthcare encounters. First, advanced voice AI systems employ natural conversation patterns, including appropriate pauses, acknowledgments, and conversational markers that signal attentiveness to the patient's narrative. The simple act of allowing patients to express their concerns completely, without interruption, creates a foundation for perceived empathy that many time-pressed human interactions unfortunately lack.
Research from the Patient Experience Institute demonstrates that patients rate voice interactions significantly higher on empathy scales compared to text-based digital triage, even when the underlying assessment protocol remains identical. This emotional connection occurs largely because voice is our most natural form of communication, carrying rich paralinguistic information through tone, pace, and emphasis that text simply cannot convey. Voice AI systems are now sophisticated enough to adapt their own communication style based on detected emotional cues, responding with appropriate levels of reassurance, concern, or clinical directness depending on the situation.
The conversational structure of voice-first AI also supports narrative medicine principles by encouraging patients to tell their health stories holistically rather than reducing their experience to a series of checkboxes or dropdown selections. This approach allows for the capture of important contextual information that might be missed in more structured formats. For instance, a patient might mention in passing that their symptoms worsen after visiting certain environments—a detail that could significantly impact clinical decision-making but might not be explicitly asked about in traditional digital triage formats.
Perhaps most importantly, well-designed voice AI systems acknowledge their own limitations transparently and incorporate seamless escalation pathways to human clinicians when needed. This honesty about capabilities builds trust with patients while ensuring they receive appropriate levels of support. The best implementations function as collaborative partners in the care team rather than replacements for human connection. By handling routine triage functions compassionately and efficiently, these systems actually create more space for meaningful human-to-human interactions when they're most valuable—allowing clinicians to focus their empathy and expertise on complex cases while AI handles more straightforward situations with surprising humanity.
Many healthcare organizations implementing voice-first triage report an interesting secondary benefit: the example set by well-designed AI conversations actually improves the quality of human clinical interactions by modeling thorough, patient-centered communication approaches. As one physician noted in a recent implementation case study, "Observing how the AI patiently explores symptoms without rushing to conclusions has made me more conscious of my own interview techniques."
Accessibility and Inclusion Benefits
Voice-first AI represents a significant leap forward in making healthcare triage more accessible to diverse patient populations who have historically been underserved by digital health solutions. For the approximately 36 million American adults with limited English proficiency, voice technologies now offer real-time translation capabilities that allow patients to speak in their native language while the system both understands their input and responds appropriately. This multilingual capability eliminates critical language barriers that often lead to healthcare disparities and misunderstandings, ensuring that important clinical information isn't lost in translation during the crucial triage phase of care.
The nearly 26% of American adults living with some form of disability find particular benefit in voice-based interfaces. Patients with visual impairments no longer need to navigate complex visual interfaces or rely on inconsistent screen reader compatibility. Those with motor limitations who struggle with typing or precise mouse movements can simply speak naturally to access triage services. For individuals with cognitive disabilities, the conversational approach eliminates the burden of understanding complex medical terminology or navigating multi-step digital processes, instead allowing them to describe their concerns in their own words at their own pace.
The digital divide—the gap between those who have ready access to computers and the internet and those who do not—represents another significant barrier that voice technology helps overcome. Approximately 24 million Americans lack home internet access, with disproportionate impact on rural communities, low-income populations, and older adults. Voice-first triage systems that can operate via standard telephone technology provide a critical bridge across this divide, enabling digital triage benefits without requiring smartphones, computers, or broadband connections. This telephone accessibility means that sophisticated clinical algorithms can serve populations who might otherwise be excluded from digital health innovations.
Age-related barriers to technology adoption present particular challenges in healthcare, where older adults typically have the highest utilization rates. Voice interfaces offer a solution that aligns with natural communication preferences and doesn't require learning new technical skills. Research from the Healthcare Accessibility Project shows that patients over 65 demonstrate adoption rates for voice-based health tools at nearly three times the rate of traditional digital interfaces, with significantly higher satisfaction scores. This preference likely stems from both the reduced cognitive load of voice interaction and the familiarity of telephone-like communication patterns.
Beyond technical accessibility, voice-first AI also addresses cultural barriers to healthcare engagement. Many communities have strong oral traditions and preferences for verbal communication over written forms. Voice interfaces honor these cultural communication preferences while still delivering the benefits of algorithmic triage. Additionally, well-designed voice systems can be programmed with cultural competence considerations, adapting their communication style, health question framing, and response patterns to align with diverse cultural expectations around healthcare conversations. This cultural sensitivity helps build trust with communities that have historically experienced discrimination or misunderstanding in healthcare settings.
Efficiency and Accuracy Improvements
The implementation of voice-first AI in healthcare triage delivers remarkable efficiency gains while simultaneously improving clinical accuracy—a rare combination in healthcare innovation where quality and cost improvements often work in opposition. From an operational perspective, voice AI systems have demonstrated the capacity to handle up to 70% of initial patient triage interactions without requiring human intervention, according to a comprehensive analysis by the Healthcare Efficiency Consortium. This dramatic reduction in routine triage burden allows clinical staff to focus their expertise on complex cases and high-value interventions rather than repetitive questioning and documentation.
Time savings represent another significant efficiency metric, with voice-first triage reducing average patient wait times by 37-62% across various implementation studies. This improvement stems primarily from the AI's ability to conduct multiple patient assessments simultaneously, eliminating the queue formation inherent in human-staffed triage processes. For healthcare organizations, this translates to higher patient satisfaction, better utilization of clinical resources, and the ability to manage volume fluctuations without staffing changes. From the patient perspective, faster triage means quicker access to appropriate care pathways and reduced time spent in uncertainty about their health concerns.
Documentation quality improvements offer benefits that extend far beyond the initial triage interaction. Voice AI systems generate comprehensive, structured clinical documentation that integrates directly with electronic health records, eliminating transcription errors and inconsistent documentation practices. These detailed records include not just the final triage determination but the complete conversation context, creating rich clinical narratives that provide valuable insights for subsequent care. Many clinicians report that AI-generated triage documentation provides more thorough symptom exploration and detailed patient history than typical human-conducted triage, likely because the AI never feels rushed or fatigued.
The accuracy advantages of voice-first AI stem from several factors working in concert. Unlike human clinicians who may vary in their adherence to evidence-based protocols, AI systems apply consistent, up-to-date clinical guidelines to every patient interaction. These systems also benefit from collective learning across thousands of cases, recognizing subtle patterns and correlations that might escape even experienced clinicians. A multi-center study published in the Journal of Medical Systems demonstrated that voice AI triage achieved accuracy rates comparable to experienced nurse triage for common conditions while significantly outperforming human triage for rare presentations where pattern recognition from vast data sets provided an advantage.
Perhaps most importantly, voice AI excels at thorough, methodical information gathering without the cognitive biases that can affect human judgment. The AI never makes assumptions based on a patient's appearance, background, or communication style, and it explores all relevant symptom patterns with equal diligence regardless of its initial hypothesis. This thoroughness helps identify critical clinical indicators that might otherwise be overlooked, particularly for conditions where symptoms may be subtle or atypical. The Clinical Decision Support Initiative has documented numerous cases where voice AI triage identified serious conditions that might have been missed in traditional triage processes, creating a safety net that benefits both patients and providers.
Implementation Challenges and Solutions
Despite the compelling benefits of voice-first AI for healthcare triage, organizations implementing these technologies face several significant challenges that must be thoughtfully addressed for successful adoption. Integration with existing healthcare IT infrastructure often presents the first hurdle, as many healthcare systems operate with a complex patchwork of legacy technologies that weren't designed for modern AI interaction. Leading organizations overcome this challenge by implementing middleware solutions that create standardized API connections between voice AI platforms and existing EHR systems, appointment schedulers, and clinical communication tools. This approach allows for phased implementation without requiring wholesale replacement of foundational IT systems.
Provider resistance represents another common implementation barrier, with clinicians sometimes expressing concerns about AI replacing human judgment or changing established workflows. Successful implementations address these concerns through collaborative design processes that involve clinical staff from the earliest planning stages. Organizations that position voice AI as an assistive technology that handles routine cases while elevating the importance of human expertise for complex situations typically see higher adoption rates among clinical teams. Transparent performance metrics that demonstrate both the AI's capabilities and its limitations help build trust with the clinical teams who will ultimately work alongside these systems.
Privacy and security considerations require particular attention given the sensitive nature of healthcare communications. Organizations must implement robust data governance frameworks that address not just regulatory compliance but also ethical usage of patient information. Technical solutions include end-to-end encryption, secure cloud architectures with appropriate access controls, and clear data retention policies. Many successful implementations also incorporate explicit consent processes that clearly explain to patients how their voice data will be used, stored, and protected, building trust in this new interaction modality.
The diversity of patient populations presents both linguistic and cultural implementation challenges that must be addressed for equitable deployment. Organizations serving multilingual communities need voice AI solutions with robust translation capabilities across all relevant languages, including regional dialects and culturally specific health terminology. Beyond mere translation, cultural adaptation of conversation flows, question framing, and response patterns is essential for building trust across diverse populations. Leading implementers work with cultural consultants to ensure their voice AI interactions respect cultural norms around health discussions and decision-making processes.
Measuring success represents a final implementation challenge, as organizations need meaningful metrics that capture both operational efficiencies and patient experience impacts. Comprehensive evaluation frameworks that include clinical accuracy metrics, patient satisfaction measures, staff impact assessments, and financial outcomes provide the most complete picture of implementation success. Organizations that establish clear baseline measurements before implementation and track changes across multiple dimensions are best positioned to demonstrate return on investment and guide ongoing optimization of their voice AI triage systems.
Perhaps the most effective implementation approach comes from organizations that adopt a continuous improvement mindset, viewing their voice AI triage system not as a finished product but as an evolving capability that will grow more sophisticated over time. By establishing clear feedback loops where both patient and provider experiences inform ongoing development, these organizations create virtuous cycles of improvement that progressively enhance both the technology's capabilities and its integration into clinical workflows. The Digital Transformation Resource Center offers comprehensive implementation playbooks based on successfully deployed voice AI triage systems across diverse healthcare settings.
Real-World Success Stories
The theoretical benefits of voice-first AI are compelling, but real-world implementations provide the most convincing evidence of this technology's transformative impact on healthcare triage. Midwest Health Network, a 12-hospital system serving urban and rural communities, implemented voice-first triage in 2022 amid severe staffing shortages in their centralized nurse triage center. Within six months, their voice AI system was successfully handling 68% of incoming triage calls without human intervention, while maintaining patient satisfaction scores within two percentage points of nurse-conducted triage. More impressively, the AI system identified 23 cases of potential stroke that initial human triage might have categorized as less urgent, demonstrating the life-saving potential of algorithmic consistency in emergency situations.
On the opposite coast, Pacific Community Health Plan deployed voice-first triage specifically to address care disparities among their diverse membership base, which includes substantial immigrant populations speaking 17 different primary languages. Their implementation focused on multilingual capabilities and cultural adaptation, resulting in a 47% increase in digital triage utilization among limited English proficiency members. Clinical outcomes data showed a 31% reduction in avoidable emergency department visits among these previously underserved populations, suggesting that improved triage accessibility directly translated to more appropriate care utilization patterns. The health plan's Chief Health Equity Officer noted, "Voice AI has accomplished what years of traditional outreach couldn't—creating a culturally comfortable digital front door that our diverse members actually want to use."
Rural applications demonstrate another compelling use case, as exemplified by Mountain States Healthcare Collaborative. This network of critical access hospitals implemented voice-first triage to extend their limited clinical resources across a vast geographic area where many patients lack broadband internet access but do have telephone connectivity. Their voice AI triage system, accessible via standard telephone lines, reduced unnecessary 60+ mile patient trips to emergency departments by providing appropriate home care guidance for minor conditions while ensuring those with truly urgent needs sought immediate care. The system's ability to function effectively over low-bandwidth connections proved crucial in mountainous regions where even cellular service is inconsistent.
Pediatric implementations present unique challenges due to the developmental variations in children's symptom presentation. Children's Health Alliance tackled these challenges by developing age-specific voice interaction models that adjust questioning techniques and language complexity based on whether a parent or the child patient is speaking. Their specialized implementation demonstrated a 42% reduction in unnecessary urgent care visits while identifying high-risk situations requiring immediate intervention with 94% accuracy compared to pediatric nurse triage. Parents particularly appreciated the system's ability to recognize when a child's condition warranted in-person evaluation even when the specific symptoms might not trigger concern in adult triage protocols.
Perhaps the most illuminating success stories come from specialized implementations addressing specific clinical challenges. The Veterans Behavioral Health Initiative implemented voice-first triage specifically for mental health concerns, creating an environment where veterans could discuss sensitive psychological symptoms without the stigma sometimes experienced in person. This implementation not only achieved high utilization rates but also identified numerous high-risk situations requiring immediate intervention that veterans admitted they would not have disclosed in traditional screening formats. The voice AI's nonjudgmental, consistent approach created psychological safety that facilitated honest disclosure of suicidal ideation, substance use, and other sensitive concerns, creating life-saving intervention opportunities that might otherwise have been missed.
These diverse success stories share common elements: thoughtful implementation strategies, clear metrics for success, and a commitment to continuous improvement based on real-world outcomes. Organizations interested in implementing similar solutions can find detailed case studies through the Implementation Success Network, which maintains a repository of implementation approaches, challenges overcome, and lessons learned across diverse healthcare contexts.
Future Trends and Developments
The evolution of voice-first AI in healthcare triage continues at a remarkable pace, with several emerging trends poised to further transform the patient experience in coming years. Multimodal integration represents perhaps the most significant near-term development, with voice interfaces increasingly combining with visual elements, secure messaging, and video capabilities to create comprehensive triage experiences. These integrated systems allow patients to begin with natural voice interaction but seamlessly transition to showing a rash via camera, receiving illustrated home care instructions, or escalating to video consultation when clinically indicated. This flexibility maintains the accessibility benefits of voice while incorporating visual information when beneficial for clinical assessment.
Emotion recognition capabilities are advancing rapidly, with next-generation systems able to detect subtle vocal biomarkers indicating anxiety, pain levels, and psychological distress. These capabilities enable more responsive triage that adapts not just to clinical information but to the patient's emotional state, providing appropriate reassurance or escalation based on detected distress levels. Early research indicates that voice-based emotion recognition may actually outperform human assessment in some contexts, particularly in detecting undisclosed depression and anxiety that influence health-seeking behavior. As these capabilities mature, they promise to create more empathetic digital experiences that respond appropriately to both spoken and unspoken patient needs.
Ambient intelligence represents another frontier, with voice AI systems evolving from reactive query-response models to proactive health monitoring. Advanced systems in development can detect subtle changes in regular voice patterns that might indicate emerging health concerns such as cognitive changes, respiratory conditions, or psychological distress. For chronically ill patients, these ambient monitoring capabilities could enable earlier intervention before conditions deteriorate to the point of requiring emergency care. The integration of voice biomarkers with other passive monitoring approaches creates comprehensive health surveillance with minimal patient burden, potentially transforming chronic disease management.
The rise of federated learning approaches promises to address one of the most significant limitations in current voice AI implementations: the need for massive centralized data sets to train effective algorithms. Federated learning allows multiple healthcare organizations to collaboratively improve AI capabilities without sharing sensitive patient data, instead updating algorithms based on local learning that is then aggregated without exposing underlying information. This approach enables rapid improvement of voice AI performance while maintaining strict privacy protections, accelerating the development of capabilities for rare conditions and diverse patient populations that might be underrepresented in any single organization's data.
Perhaps most exciting is the potential for voice-first AI to fundamentally redesign care pathways rather than simply digitizing existing triage processes. Forward-thinking healthcare organizations are using voice AI as the cornerstone of "triage to treatment" models where the initial conversation flows directly into appropriate care delivery—whether that's automated prescription renewal, scheduled diagnostic testing, virtual consultation, or in-person evaluation. This seamless continuum eliminates traditional hand-offs between triage and care delivery, creating more coherent patient journeys and reducing the information loss that typically occurs across care transitions.
As these trends converge, we can envision a future where voice-first AI serves as an intelligent health companion that knows each patient's history, preferences, and unique needs—providing personalized guidance that considers their specific clinical situation and communication style. This evolution from standardized triage to individualized health navigation represents the ultimate fulfillment of voice AI's promise to humanize digital healthcare while leveraging technological capabilities to deliver care that is simultaneously more personal and more evidence-based than traditional approaches could achieve.
Conclusion
The integration of voice-first AI into healthcare triage represents far more than a technological upgrade—it reflects a fundamental shift toward more human-centered digital healthcare experiences. By reclaiming the natural power of conversation in clinical interactions, these technologies are successfully bridging the often jarring divide between high-tech healthcare delivery and the deeply personal experience of seeking care. The evidence presented throughout this article demonstrates that voice-first AI is uniquely capable of addressing seemingly contradictory healthcare challenges: simultaneously improving clinical efficiency while enhancing patient connections; expanding access while reducing costs; and leveraging sophisticated algorithms while creating more natural human experiences.
While implementation challenges exist, the organizations successfully deploying these technologies show that thoughtful integration strategies, clinician involvement, and commitment to continuous improvement can overcome initial barriers. The resulting benefits—dramatically reduced wait times, improved clinical documentation, enhanced accessibility, and more appropriate care utilization—create compelling value propositions for patients, providers, and healthcare systems alike. The most successful implementations recognize that voice-first AI isn't merely a replacement for existing processes but an opportunity to fundamentally rethink how patients access and experience care from the earliest touchpoints.
Looking ahead, the continued evolution of voice technology promises even more transformative possibilities as systems become increasingly capable of understanding context, emotion, and subtle health indicators while seamlessly connecting patients to appropriate care pathways. As healthcare continues its digital transformation journey, voice-first AI stands as a powerful example of technology that enhances rather than diminishes the human dimensions of care—proving that advanced digital capabilities and empathetic connections can coexist and even reinforce one another. For healthcare organizations seeking to improve both operational performance and patient experience, voice-first AI triage represents not just a technological investment but a strategic commitment to creating more accessible, responsive, and human-centered healthcare for all.
FAQ Section
What is voice-first AI triage in healthcare? Voice-first AI triage uses natural language processing and clinical algorithms to assess patient symptoms through conversational voice interfaces. It allows patients to describe health concerns in their own words and provides appropriate guidance based on clinical protocols and evidence-based medicine.
How does voice-first AI improve accessibility in healthcare? Voice-first AI improves healthcare accessibility by removing barriers for patients with limited digital literacy, visual impairments, motor limitations, or language differences. It works over standard telephone technology, making it accessible to those without smartphones or internet access, while offering real-time translation across multiple languages.
Is voice-first AI as accurate as human triage nurses? Studies show that voice-first AI achieves comparable accuracy rates to experienced triage nurses for common conditions, with accuracy rates around 93%. For rare conditions, AI may outperform humans due to its ability to recognize patterns across thousands of cases and apply consistent protocols without fatigue or cognitive bias.
How do patients feel about interacting with AI for triage? Patient satisfaction ratings for voice-first AI triage average 4.7/5, approximately 15% higher than text-based digital triage. Patients particularly appreciate the reduced wait times, 24/7 availability, and the system's ability to listen to their complete symptom narrative without rushing or interrupting.
What cost savings can healthcare organizations expect? Healthcare organizations typically see 70-80% cost reductions per triage encounter compared to traditional nurse triage, with ROI timelines of 8-12 months. Additional savings come from reduced unnecessary ED and urgent care visits through more accurate triage and appropriate care navigation.
How does voice-first AI handle multiple languages? Leading voice-first AI triage platforms support 20+ languages with dialect recognition, covering approximately 96% of the US population. They offer real-time translation capabilities that allow patients to speak in their native language while maintaining clinical accuracy and cultural appropriateness.
What are the main implementation challenges? The main implementation challenges include integration with existing healthcare IT infrastructure, clinician resistance, privacy and security considerations, and adapting to diverse patient populations. Organizations with successful implementations address these through collaborative design processes, middleware solutions, and clear governance frameworks.
Can voice-first AI detect emergency situations? Yes, voice-first AI systems are designed with robust safety protocols to identify emergency situations. They can detect urgent warning signs through both explicit symptoms and subtle speech patterns, immediately escalating to human providers or emergency services when necessary.
How does voice-first AI protect patient privacy? Voice-first AI protects privacy through end-to-end encryption, secure cloud architectures, and compliance with healthcare data regulations like HIPAA. Systems typically include explicit consent processes, clear data retention policies, and comprehensive audit trails of all interactions.
What future developments are expected in voice-first AI triage? Future developments include multimodal integration with visual elements, advanced emotion recognition capabilities, ambient intelligence for proactive monitoring, federated learning approaches for privacy-preserving improvement, and seamless integration with treatment pathways for end-to-end patient journeys.
Additional Resources
Healthcare Voice Technology Alliance - Comprehensive Implementation Guide
This detailed resource provides step-by-step implementation guidance for healthcare organizations, including technical specifications, workflow integration approaches, and change management strategies.Journal of Medical Internet Research - Special Issue: "Voice Interfaces in Clinical Care"
This peer-reviewed collection features 12 research papers examining voice technology effectiveness across various healthcare applications, with particular focus on triage, remote monitoring, and patient engagement.Digital Health Equity Foundation - Voice Technology Accessibility Standards
These standards provide comprehensive guidelines for ensuring voice-first healthcare technologies meet the needs of diverse populations, including multilingual requirements, cognitive accessibility considerations, and cultural adaptation frameworks.Health Tech Future Report: Voice AI Forecast 2025-2030
This industry analysis examines emerging trends, projected adoption rates, and anticipated technological developments in voice-first healthcare applications over the next five years.Center for Patient Experience Innovation - Voice Technology Case Studies Database
This searchable repository contains detailed case studies from over 40 healthcare organizations that have implemented voice-first AI triage, including implementation approaches, challenges overcome, and measured outcomes.