How AI Triage Connects to Hospital Capacity Management
Discover how AI-powered triage systems are revolutionizing hospital capacity management by enabling real-time resource allocation, reducing wait times, and improving patient outcomes while maximizing operational efficiency.
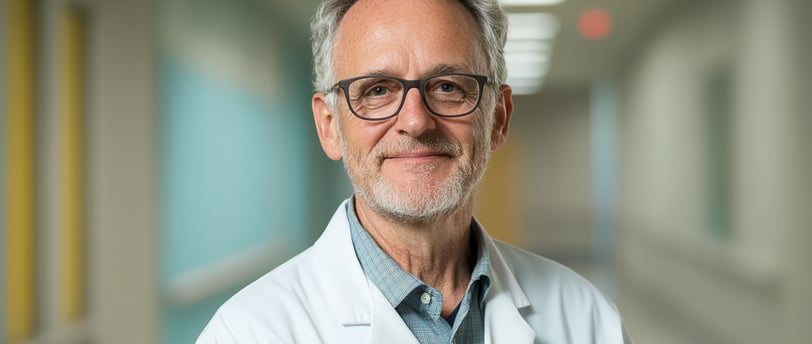
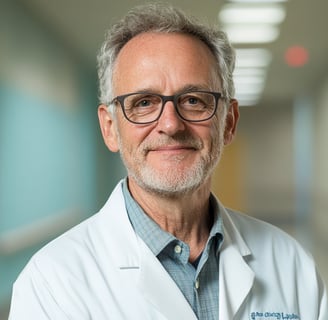
The global healthcare landscape is currently grappling with unprecedented challenges, including an aging population, a rising prevalence of chronic diseases, and a diminishing workforce. These pressures necessitate a fundamental shift in operational paradigms, with a critical focus on optimizing hospital capacity. Artificial intelligence (AI) triage systems are emerging as a transformative solution, offering a synergistic approach to enhance patient flow, rationalize resource allocation, and streamline administrative processes. By leveraging advanced machine learning and natural language processing, AI triage accurately assesses patient acuity, directs individuals to the most appropriate care settings, and provides predictive analytics for demand forecasting. This comprehensive report explores the multifaceted ways AI triage directly connects to and profoundly impacts hospital capacity management, presenting a compelling case for its strategic adoption to foster system resilience, improve patient outcomes, and ensure economic viability. While significant challenges related to data quality, interoperability, and ethical considerations persist, the demonstrated benefits across various real-world applications underscore AI's pivotal role in shaping the future of efficient and patient-centric healthcare delivery.
Introduction: The Imperative for AI in Healthcare Operations
Healthcare systems worldwide are facing an escalating crisis driven by a confluence of demographic shifts and workforce constraints. An aging global population, coupled with a rising prevalence of chronic diseases, places immense and growing demands on existing healthcare infrastructure and resources. This demographic pressure is further exacerbated by a declining healthcare workforce, leading to profound concerns regarding the quality of care and the potential compromise of patient safety. Hospitals, in particular, operate under increasing economic pressure and a critical shortage of skilled professionals, making the optimal utilization of every available capacity not merely an advantage, but an absolute necessity.
The current environment underscores a critical need for enhanced efficiency and optimized resource utilization across all healthcare operations. Unpredictable patient volumes, coupled with inherent limitations in hospital resources and clinical staffing, directly impede patient safety, compromise the quality of care, and negatively affect patient outcomes. Without a strategic and comprehensive approach to capacity management, healthcare facilities are prone to severe operational challenges, including widespread staff burnout, significant treatment delays, critical equipment shortages, and even violations of regulatory compliance protocols. Furthermore, inefficient service provision frequently results in fewer patients being treated, more cost-intensive treatments, and a pervasive increase in dissatisfaction among both patients and staff.
These persistent challenges reveal a systemic vulnerability to external pressures that traditional healthcare models struggle to address. The escalating demands stemming from an aging populace and the increasing burden of chronic diseases, compounded by a shrinking workforce, highlight a macro-level trend impacting the very sustainability of healthcare delivery. This situation suggests that AI is not just a tool for incremental improvement but a foundational requirement for building system resilience. Without integrating AI, healthcare systems risk a continuous decline in service quality and economic viability, potentially leading to a broader crisis in public trust and access to essential care. AI emerges here as a strategic enabler for systemic resilience. The interconnected challenges of staff shortages, burnout, treatment delays, and economic pressure are symptomatic of a deeper strain on capacity. By addressing these core operational inefficiencies and resource imbalances through AI-driven solutions, hospitals can significantly enhance their ability to withstand unpredictable demand and overcome workforce limitations. This capability extends beyond individual hospital efficiency, contributing to the overall stability and responsiveness of national or regional healthcare ecosystems.
Understanding AI Triage: Definition, Functions, and Goals
AI triage in healthcare represents a pivotal advancement, leveraging artificial intelligence to guide patients in managing their health and navigating to the most appropriate care settings when unwell. This technology fundamentally transforms the initial patient assessment process by automating key steps, enhancing decision-making capabilities, and significantly improving overall operational efficiency within healthcare facilities. At its core, AI triage utilizes sophisticated machine learning (ML) and natural language processing (NLP) algorithms to meticulously analyze diverse patient data, including symptoms, medical history, and real-time clinical indicators.
Core Functionalities
The operational capabilities of AI triage systems are multifaceted, designed to address various critical points in the patient journey:
Automated Patient Assessment: AI-powered systems automate the initial evaluation of patients, a crucial step for effective care prioritization and optimizing hospital workflows. This automation extends to comprehensive medical history-taking and symptom checking, often facilitated through interactive chatbots or digital intake forms.
Decision Support and Augmentation: AI functions as a powerful tool to support or, in some cases, automate clinical decision-making. It achieves this by analyzing vast datasets to identify subtle clinical patterns, predict disease severity, and suggest potential diagnoses. Importantly, AI is designed to augment, rather than replace, the expertise and skill of human clinicians, ensuring human oversight remains integral to the process.
Patient Routing and Navigation: A key function of AI triage is to intelligently direct patients to the most appropriate care pathways and settings. This includes guiding individuals to urgent care centers, telehealth consultations, emergency departments (EDs), or primary care facilities, thereby minimizing unnecessary and inefficient care utilization. For simpler health pathways, AI can even empower citizens to self-diagnose, self-refer, self-treat, and self-discharge, promoting greater patient autonomy.
Real-time Data Analysis: AI systems are capable of processing real-time data, such as vital signs, to provide immediate recommendations and detect the early onset of critical conditions, enabling swift interventions.
Primary Objectives
The deployment of AI triage systems is driven by several strategic objectives aimed at improving healthcare delivery:
Reduction of Unnecessary and Inefficient Care Pathways: By ensuring patients are directed to the correct care setting from the outset, AI significantly reduces the incidence of unnecessary emergency room visits and avoidable hospital admissions.
Improvement in Efficiency and Quality of Care: AI-based triage systems are designed to alleviate the workload on healthcare professionals while simultaneously enhancing the overall efficiency and quality of care provided to patients.
Enhancement of Patient Outcomes: Through improved precision in triage decisions, AI ensures that high-risk patients receive immediate attention, which leads to better recovery rates and a reduction in potential complications.
Reduction of Waiting Times and Overcrowding: By streamlining patient flow and expediting the decision-making process, AI directly mitigates overcrowding in high-demand areas such as emergency departments.
Support for Clinicians: AI empowers clinicians to dedicate more time to direct patient interaction and focus on higher-value clinical tasks by automating routine administrative burdens and initial patient assessments.
The capabilities of AI triage represent a profound shift in how patients engage with healthcare. The vision of an "AI Navigation Assistant for every citizen" and the ability for patients to "self-diagnose, self-refer, self-treat and self-discharge" for appropriate pathways extends beyond mere operational efficiency. This signifies a fundamental transformation in the patient-healthcare provider relationship, fostering greater patient autonomy and direct access to care pathways. This approach has the potential to democratize health information and reduce reliance on traditional gatekeepers. The increased patient engagement facilitated by AI-driven self-service tools directly reduces the administrative burden on healthcare staff and optimizes the initial point of contact, thereby improving overall system efficiency.
Crucially, the role of AI in triage is augmentative, not a replacement for human expertise. While AI can "automate medical history-taking" and "suggest diagnoses" , it is explicitly stated that it "cannot replace the expertise and skill of clinicians" and emphasizes the critical importance of "human control". This highlights a core design philosophy: AI serves as a tool to enhance human capabilities and decision-making, rather than fully automating complex clinical judgment, especially given the inherent "uncertainties" and "considerable risks associated with erroneous decision-making" in healthcare. Successful integration of AI requires a collaborative human-AI model, where AI efficiently handles routine, data-intensive tasks, thereby freeing clinicians to focus on complex problem-solving, empathetic patient care, and essential oversight. This collaborative model also underscores the paramount importance of trust and interpretability in AI systems for their widespread acceptance and effective deployment.
Hospital Capacity Management: Principles, Challenges, and Objectives
Hospital capacity management is a foundational operational discipline in healthcare, defined as the strategic process of aligning available hospital resources—including staff, beds, critical medical equipment, and physical space—with the real-time needs and demands of patients. A modern and effective capacity management strategy prioritizes patient throughput, operational readiness, and seamless workflow coordination to ensure that care delivery is consistently timely, safe, efficient, and highly responsive to dynamic conditions within healthcare facilities.
Key Components of Capacity Planning
Effective capacity planning within a hospital system encompasses several interconnected components:
Workforce Planning: This involves meticulously matching staffing levels to patient acuity and specific care needs. The objective is to proactively prevent staff shortages and mitigate burnout, thereby sustaining high staff satisfaction and operational efficiency.
Bed Management: This component focuses on real-time tracking of bed status across all units to reduce emergency department boarding, minimize patient wait times, and expedite bed turnover. The goal is to ensure smooth patient progression through the hospital system. This also critically includes effective management of patient length of stay (LOS) and robust discharge planning.
Equipment Capacity: This ensures the optimal utilization and ready availability of essential critical medical equipment, which is vital for enhancing resource use and reducing treatment delays.
Acuity Adaptable Rooms: An emerging concept, these rooms are designed with the flexibility to accommodate patients with varying levels of care needs without requiring transfers to different units, thereby improving workflow efficiency and resource utilization.
Strategic Objectives
The overarching objectives of hospital capacity management are designed to optimize operational performance and patient care:
Maximize Resource Utilization: This objective aims to ensure the optimal deployment of all available resources, including staff, rooms, and equipment, while also addressing supply chain issues to maintain the availability of essential hospital resources.
Match Capacity to Patient Demand: This involves accurately predicting seasonal surges, daily fluctuations, and unexpected changes in patient volume using real-time and historical data to effectively align hospital beds and staff resources.
Reduce Bottlenecks: A critical objective is to identify and proactively resolve operational impediments, such as delayed patient discharges, diagnostic testing backlogs, and inefficient workflows, before they negatively impact patient flow and satisfaction.
Improved Coordination of Patient Flow: This encompasses the targeted control of under- and overutilization of functional areas and large equipment, ensuring a harmonious patient journey from admission through to discharge.
Time Relief for Staff: A key aim is to reduce the administrative burdens on nursing staff and doctors, thereby freeing up their time for direct patient care and ensuring permanently sustainable and high-quality service.
Optimized Interdisciplinary Cooperation: This objective focuses on fostering effective synergies between different disciplines and departments, preventing unnecessary waste of capacities and improving overall operational fluidity.
Common Challenges in Healthcare Capacity Planning
Hospitals frequently encounter significant challenges in effectively managing their capacity:
Unpredictable Demand: Healthcare inherently deals with highly unpredictable patient arrivals and fluctuating demands, making accurate forecasting a complex undertaking.
Siloed Systems and Limited Visibility: Many hospitals operate with disparate IT systems, such as Real-Time Location Systems (RTLS), Electronic Health Records (EHRs), and scheduling platforms, which often function in isolation. This lack of integration severely hinders real-time situational awareness and comprehensive, integrated decision-making.
Staffing Gaps and Burnout: Addressing chronic staff shortages, preventing excessive overtime, and mitigating widespread staff burnout remain persistent and critical challenges for healthcare organizations.
Operational Bottlenecks: A multitude of operational inefficiencies create significant delays, including uncoordinated admission planning for elective patients, patient rejections, insufficient patient outflow, unnecessary internal transfers between wards, inefficient bed utilization, inadequate length of stay management, and missing discharge planning.
Communication Deficits: Poor or fragmented communication between different clinical disciplines and administrative departments further exacerbates existing capacity issues.
Balancing Quality and Economy: Hospitals face the perpetual and delicate challenge of providing sufficient capacity to ensure high-quality patient care while simultaneously maintaining economic efficiency and financial viability.
The challenges described highlight the "invisible" costs of inefficient capacity management. While direct financial costs are evident ("every capacity costs," "treatments are more cost-intensive" ), the available information also points to significant indirect costs. These include "staff burnout," "treatment delays," "violations of compliance protocols" , and "dissatisfied patients and staff". This indicates that inefficient capacity management does not merely impact the financial bottom line; it erodes the human capital (e.g., staff morale and retention) and social capital (e.g., patient trust and community health outcomes) of the healthcare system. Consequently, investments in capacity optimization, including AI, yield a multifaceted return on investment that extends far beyond purely financial metrics.
This situation also underscores a notable shift from static to dynamic capacity management. Traditional methods often struggle with "unpredictable patient volumes" and "uncoordinated admission planning". In contrast, modern strategies emphasize responding to "real-time patient needs," leveraging "predictive AI forecasting," and the ability to "adjust in Real Time". This signifies a clear trend within the industry, moving away from fixed, reactive capacity planning towards more agile, data-driven, and continuously adjusting operational models. The adoption of predictive analytics and tools that provide real-time visibility is a direct response to the inherent unpredictability of healthcare demand, enabling a paradigm shift towards greater operational flexibility and responsiveness.
The Synergistic Connection: How AI Triage Optimizes Hospital Capacity
The integration of AI triage systems fundamentally transforms hospital capacity management by creating a powerful synergy between patient assessment and resource optimization. This connection is not merely additive; it creates a multiplier effect that enhances efficiency, improves patient outcomes, and reduces operational strain across the entire healthcare continuum.
Enhancing Patient Flow and Reducing Wait Times
AI-powered triage systems are instrumental in streamlining patient flow and expediting decision-making processes. They achieve this by automating initial patient assessments and intelligently prioritizing cases based on real-time data, ensuring patients are guided to the most appropriate level of care. This strategic routing significantly reduces unnecessary emergency room visits and optimizes the patient's entire care journey.
The impact of this includes:
Reduced Overcrowding and Wait Times: AI directly mitigates overcrowding in emergency departments (EDs), which is a primary cause of prolonged wait times and delayed treatment. By optimizing patient flow, AI-driven tools effectively reduce bottlenecks, facilitating faster access to care for all patients.
Improved Throughput: AI demonstrably enhances ED efficiency through proactive resource management and a reduction in patient waiting times. Empirical evidence supports this, with case studies showing AI reducing the average ED length of stay (LOS) by 4.15% and improving overall ED throughput by 15% at institutions like Mount Sinai Health System.
Expedited Decision-Making: AI provides instant recommendations based on patient inputs, enabling rapid assessment and prioritization of cases according to their severity. This ensures that high-risk patients receive immediate medical attention, while lower-risk patients are managed more efficiently, preventing critical delays.
The efficiency gains within the ED, driven by AI, create a significant ripple effect across the entire hospital system. Overcrowding in the ED is a well-documented bottleneck. AI's ability to reduce ED wait times and improve throughput has profound implications beyond the emergency department itself. An efficient ED translates directly to faster patient movement to inpatient beds, diagnostic services, or discharge, thereby preventing backlogs and improving flow throughout the entire hospital system. This positions the ED as a critical "pressure valve" for hospital capacity, where AI's impact generates cascading benefits. This represents a shift from reactive to proactive patient journey management. Traditional triage methods are often reactive, responding to patient presentations as they occur. AI, however, leverages predictive analytics to "predict patient demand" , "estimate the severity of incoming cases based on prehospital data" , and "predict patient inflow by analyzing historical admission patterns, seasonal trends, weather data, public health alerts, and even local event schedules". This signifies a fundamental transformation from merely managing the current patient load to proactively anticipating future demand and patient needs. This proactive approach enables pre-emptive resource allocation, timely staffing adjustments, and even the activation of overflow protocols, effectively transforming crisis management into strategic foresight.
Optimizing Resource Allocation (Staff, Beds, Equipment)
AI-assisted triage systems provide invaluable data-driven insights that empower healthcare administrators to strategically allocate resources across the hospital. By accurately predicting patient volumes and acuity levels, AI enables health systems to adjust staffing levels in real-time based on demand, ensuring optimal personnel deployment.
This leads to significant improvements in:
Workforce Optimization: AI can optimize staffing schedules and ensure the appropriate number of personnel are available during critical periods. This proactive approach helps prevent staff shortages and burnout, contributing to high staff satisfaction and sustained operational efficiency.
Bed Management Efficiency: AI models that integrate real-time hospital capacity data can dynamically adjust resource allocation, thereby minimizing delays in patient transfers and discharges. AI-powered bed management systems continuously monitor patient discharge predictions, inpatient bed turnover rates, and resource availability across various departments to recommend optimal bed assignments based on patient needs and specialty requirements.
Equipment Utilization: AI facilitates the more effective allocation of critical medical equipment and treatment spaces. It optimizes the use of high-demand equipment, enhancing overall resource utilization and reducing delays in diagnostic and therapeutic procedures.
Proactive Preparedness: Should an AI system detect an impending influx of patients with similar symptoms—potentially indicative of an outbreak—it can promptly alert administrators to prepare isolation units and stock necessary medications. This proactive capability significantly enhances the hospital's ability to manage emergencies and prevents critical resource shortages.
AI serves as a powerful catalyst for interdepartmental coordination. Resource allocation is not confined to individual departments; it is a complex interplay across the entire hospital ecosystem. AI's capability to "coordinate ancillary services such as housekeeping or transport to expedite bed turnover" and to "dynamically adjust resource allocation" across the hospital is a key differentiator. AI functions as an intelligent orchestrator, dismantling traditional departmental silos by providing a unified, real-time view of resource availability and demand across the entire facility. This fosters a more integrated and responsive operational model. This integrated approach results in a more fluid and responsive hospital environment, where resources are not merely optimized within their respective silos but are strategically deployed across the entire patient journey, thereby improving overall system performance and reducing friction points.
Furthermore, there is a clear economic value to predictive resource deployment. Optimizing resource allocation directly impacts cost-effectiveness. By reducing "unnecessary ER visits and hospital admissions," AI contributes to "significant cost savings". Predictive AI enables hospitals to avoid both resource under-utilization (which leads to wasted resources) and over-utilization (which contributes to staff burnout and increased overtime costs) by precisely matching supply to demand. This translates directly into tangible financial benefits, positioning AI as an investment with a clear and measurable return. This economic advantage makes AI not only a clinical tool but also a strategic financial asset for healthcare organizations, enabling them to operate more sustainably amidst increasing cost pressures.
Predictive Analytics for Demand Forecasting
AI and Machine Learning algorithms are transforming demand forecasting in healthcare by analyzing vast amounts of historical patient data, real-time inputs, and a range of external factors. This enables accurate predictions of patient inflow, demand patterns, and even the acuity of incoming cases.
The impact of this predictive capability is substantial:
Anticipating Surges: Hospitals can accurately anticipate seasonal surges, daily fluctuations, and unexpected changes in patient volume. This foresight allows them to proactively plan staffing, equipment, and bed capacity accordingly, ensuring readiness for peak demands.
Proactive Staffing: Predictive analytics empowers hospitals to adjust staffing levels in advance, leading to reported reductions in emergency room wait times by more effectively matching available capacity to anticipated demand. One Canadian hospital, for instance, reported a 50% reduction in ER wait times through such an AI-based system.
Early Discharge Planning: AI can predict patient demand and facilitate early discharge planning for stable patients, which is crucial for freeing up beds and improving inpatient flow.
Optimized Scheduling: AI can predict which patients are likely to miss their appointments, enabling proactive re-allocation of those slots and significantly reducing "Did Not Attend" (DNA) rates. A six-month pilot at an Essex NHS Trust, for example, saw AI reduce DNA rates by almost 30%, allowing over 1,900 additional patients to be seen and projecting annual savings of approximately £27.5 million for that trust.
The power of AI in prediction extends beyond traditional historical data analysis to multi-factor prediction. While historical data forms a crucial foundation , AI's predictive models also integrate a broader array of factors such as "seasonal trends, weather data, public health alerts, and even local event schedules" , and "traffic" conditions. This demonstrates AI's unique capability to move beyond internal, siloed data to incorporate a broad spectrum of external, seemingly unrelated factors into its predictive models. This holistic data integration provides a far more accurate and robust forecast than traditional, more limited methods. This multi-factor predictive power enables hospitals to anticipate demand with unprecedented accuracy, fundamentally transforming operations from a reactive stance to a truly anticipatory one, leading to more resilient and efficient healthcare delivery.
The strategic impact of reducing "Did Not Attend" (DNA) rates is also noteworthy. The success of the NHS pilot in reducing DNA rates by almost 30%, thereby enabling 1,900+ extra patients to be seen , highlights a specific yet often overlooked aspect of capacity management. Reducing DNAs directly translates to increased clinic throughput, better utilization of clinician time, and significant cost savings by avoiding wasted appointments. This effectively frees up existing capacity without the need for new infrastructure development, representing a highly efficient form of capacity optimization. AI's predictive capabilities enable proactive intervention, such as offering alternative slots or making back-up bookings, which directly improves resource utilization and patient access by minimizing empty appointment slots.
Streamlining Administrative Workflows
AI-assisted triage plays a crucial role in alleviating the substantial administrative burden on healthcare staff by automating numerous patient inquiry workflows and routine tasks. These AI tools seamlessly integrate with existing electronic health records (EHRs) and other hospital systems, creating a more efficient operational environment.
This streamlining yields several significant impacts:
Reduced Administrative Strain: Nurses and call center agents are freed from spending extensive hours on basic triage questions, as AI handles these initial assessments and flags only the most complex cases for human review. This allows staff to redirect their focus to higher-value tasks and direct patient care, thereby reducing burnout and improving overall job satisfaction.
Automated Documentation: AI-powered medical scribes can efficiently manage documentation processes, enabling healthcare providers to concentrate more on patient interactions rather than being preoccupied with paperwork. Furthermore, advanced Generative AI models can summarize clinical consultations or generate initial drafts of discharge instructions, significantly easing documentation workload.
Efficient Communication: AI-driven virtual assistants can effectively communicate with patients, providing essential information regarding wait times, procedures, or aftercare instructions. AI-enabled call routing systems can significantly reduce patient wait times and frustration by directing inquiries to the correct department or individual.
Minimized Errors: The automation of workflows through AI not only accelerates processes but also significantly minimizes the risk of human error in documentation and administrative tasks.
This administrative streamlining has a profound effect on reclaiming clinician time for core competencies. The consistent emphasis in the available information on AI "freeing up professionals' time" , enabling "staff to focus on higher-value tasks" , and allowing "clinicians to focus more on patient care and less on administrative burdens" points to a critical benefit. This is not merely about achieving efficiency; it is fundamentally about optimizing the human capital within healthcare. By offloading repetitive, time-consuming administrative tasks to AI, highly trained medical professionals can dedicate their expertise to complex clinical decision-making, direct patient interaction, and empathetic care—capabilities that are uniquely human. This has profound implications for job satisfaction, professional retention, and the overall quality of human-centered care. The reduction in administrative workload directly leads to decreased clinician burnout, improved morale, and ultimately, a higher quality of direct patient care.
Moreover, administrative automation creates a valuable data feedback loop. AI automates documentation and integrates seamlessly with EHRs , which means that more accurate and complete data is captured. The data generated and streamlined by these administrative AI tools then feeds directly back into the AI triage and capacity management systems, continuously improving their predictive accuracy and decision-making capabilities. This establishes a virtuous cycle where administrative efficiency directly enhances clinical intelligence. The administrative benefits of AI are therefore not isolated; they contribute to the overall intelligence and responsiveness of the entire AI-driven healthcare ecosystem, making the system progressively smarter over time.
Table 1: Key Benefits of AI Triage on Hospital Capacity Management
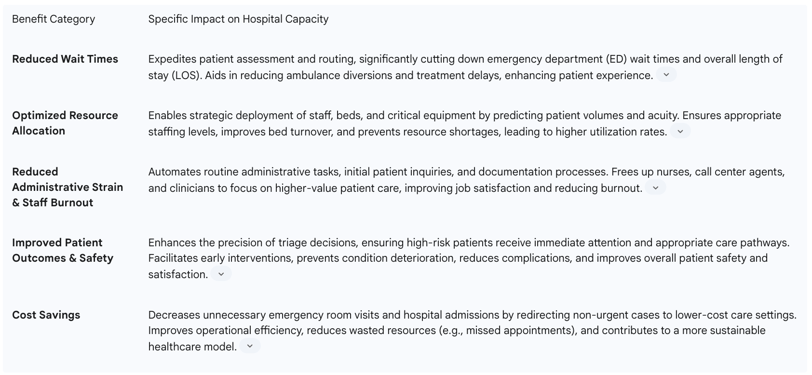
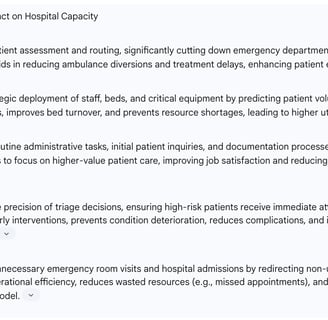
Data Foundations: Information Driving AI Triage for Capacity Insights
The efficacy of AI triage systems in optimizing hospital capacity is fundamentally dependent on the quality, volume, and diversity of the data they process. These systems leverage sophisticated machine learning and natural language processing capabilities to analyze a wide array of information, transforming raw data into actionable insights for capacity management.
The types of data utilized by AI-driven triage systems, and their contribution to real-time insights, include:
Vital Signs: Real-time physiological data, such as heart rate, respiratory rate, and oxygen saturation, can be captured through various monitoring devices, including wearables. These data points are critical for identifying patients at immediate risk of deterioration, enabling proactive intervention in conditions like acute heart failure or respiratory distress.
Symptoms and Patient Complaints: Patient-reported symptoms and chief complaints, often initially captured in unstructured formats like free-text notes or chatbot interactions, are processed by Natural Language Processing (NLP) algorithms. NLP allows AI systems to interpret these textual inputs, discerning nuances that might be overlooked in structured data alone and hinting at subtle risk factors or underlying conditions.
Medical History: Comprehensive medical history, including past diagnoses, chronic conditions, and previous treatments, is crucial for contextualizing current symptoms and predicting potential risks. The integration of Electronic Health Record (EHR) data with real-time inputs allows AI-driven triage systems to adapt more effectively to a wide array of patient presentations, enhancing predictive accuracy, especially for patients with pre-existing conditions.
Demographics: Patient demographic information (e.g., age, gender, location, socioeconomic factors) provides essential context for risk categorization and understanding population-level health trends.
Electronic Health Records (EHRs): EHRs serve as a rich repository of structured and unstructured patient data, including diagnoses, medications, lab results, and clinical notes. AI algorithms can clean, manage, and analyze these diverse datasets to identify dynamic patterns, improve clinical care processes, and provide sophisticated algorithms for better outcome prediction.
Real-time Clinical Data: Beyond vital signs, this includes real-time updates on patient status, ongoing treatments, and current resource availability within the hospital. This dynamic data allows AI to adjust prioritization and resource allocation based on current conditions, such as patient load and bed availability.
Environmental and External Factors: AI's predictive models extend beyond internal clinical data to incorporate external factors that influence patient demand, such as seasonal trends, weather conditions, public health alerts, and even local event schedules and traffic patterns. This holistic approach provides a more accurate forecast of patient influx.
Medical Images and Scans: AI can interpret complex medical images like X-rays, MRI scans, and brain scans to detect fractures, identify early signs of diseases, or spot subtle lesions that might be missed by human review. This diagnostic support directly impacts the speed and accuracy of triage.
Lab Results and Genomic Data: Integration of lab results and genomic data provides deeper insights into a patient's condition and predisposition to certain diseases, enabling more personalized and predictive care pathways.
Biometric Measurements and Voice/Sound Inputs: Advanced multimodal AI systems can process biometric measurements and voice/sound inputs, further enriching the data profile for comprehensive diagnostic capabilities and enhanced security.
Patient Discharge Predictions and Inpatient Bed Turnover: AI systems monitor and predict patient discharge patterns and inpatient bed turnover rates across departments, providing crucial information for optimizing bed assignments and coordinating ancillary services to expedite patient flow.
The effectiveness of AI is directly proportional to the volume, variety, and quality of data it processes. The ability to integrate a blend of real-time data (e.g., vital signs, current symptoms) with extensive historical data (e.g., EHRs, past diagnoses) is crucial for generating accurate predictions and nuanced insights. Furthermore, NLP's capability to extract meaningful insights from unstructured data, such as patient notes and complaints, unlocks a rich and previously underutilized source of information. This comprehensive data foundation is what allows AI triage to provide a truly holistic and dynamic view of patient needs and hospital capacity.
Table 2: Data Inputs for AI-Driven Capacity Insights
The following table outlines the diverse data inputs that fuel AI-driven triage systems, enabling them to generate comprehensive insights for hospital capacity management.
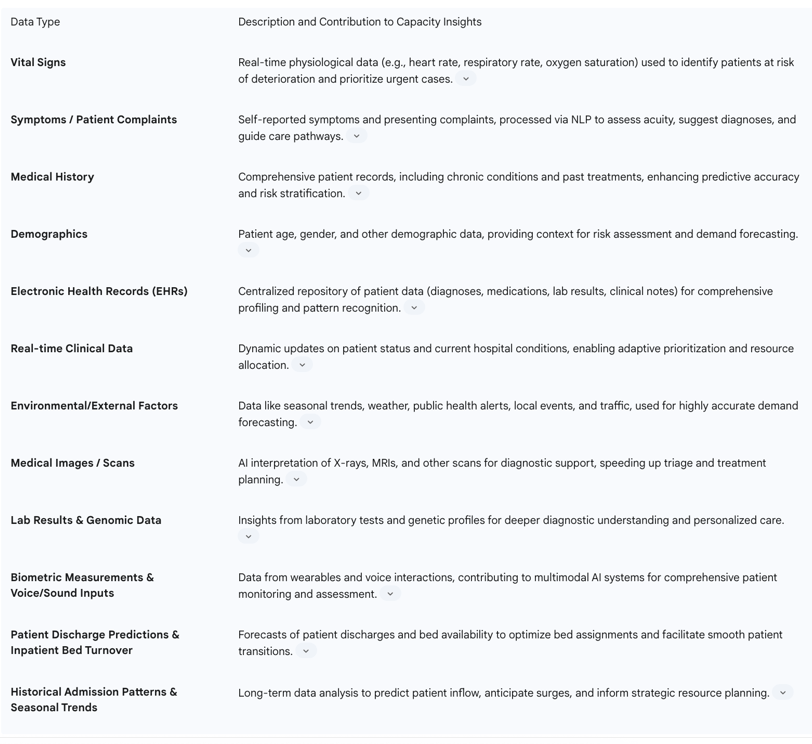
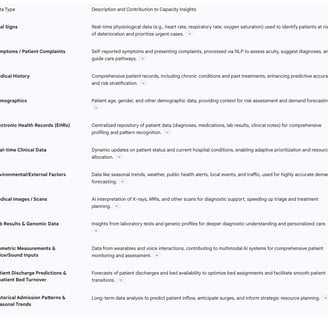
Real-World Applications and Case Studies
The theoretical benefits of AI triage and its connection to hospital capacity management are increasingly being validated through numerous real-world applications and successful pilot programs across the globe. These case studies demonstrate tangible, quantifiable improvements in efficiency, patient outcomes, and operational cost-effectiveness.
Several companies are already improving patient outcomes by reducing unnecessary and inefficient care pathways through intelligent navigation. These include firms such as Ada, Abi Health, Healthily, Rapid Health, Infermedica, Klinik, Mediktor, and Visiba, with their solutions being adopted in various parts of the NHS and worldwide. Clearstep's Smart Care Routing™ is another example of an AI triage assessment tool that optimizes patient flow by guiding individuals to the right level of care, reducing delays, and prioritizing critical cases.
Specific examples of AI in action include:
Emergency Department Flow Optimization:
Mount Sinai Health System (New York) implemented AI to predict emergency room patient flow and bed availability, resulting in a notable 15% improvement in ER throughput.
A Canadian hospital utilized an AI-based system to predict emergency department arrivals and inpatient bed demand, enabling them to adjust staffing in advance. This led to a reported 50% reduction in ER wait times by better matching capacity to demand.
A study involving the Mayo Clinic redesigned an ED's vertical processing pathway using machine learning (ML) model recommendations. This resulted in a reduction of the average ED length of stay (LOS) by 10.75 minutes (4.15%).
Appointment Scheduling and No-Show Reduction: A UK startup, Deep Medical, developed AI software in partnership with clinicians to predict which patients are likely to miss appointments by analyzing factors such as appointment history, demographics, and even weather and traffic. In a six-month pilot at an
Essex NHS Trust, this AI-driven approach cut "Did Not Attend" (DNA) rates by almost 30%, enabling an extra 1,900+ patients to be seen and projecting annual savings of approximately £27.5 million for that trust. Following this success, the NHS is scaling it to more hospitals.
Surgical Scheduling Optimization: Hospitals in London and Manchester have partnered with AI firms to optimize surgical schedules. By analyzing years of surgical data (e.g., case length, surgeon availability), AI builds predictive scheduling systems that can dynamically adjust schedules in real-time if a surgery runs long or an emergency case arises, automatically suggesting reallocation of cases to different theaters or times. This has led to fewer last-minute cancellations and better utilization of operating rooms (less downtime).
Patient Prioritization for Waiting Lists: An AI-powered software, trained on over 200 million records, is used in hospitals to prioritize high-risk patients on waiting lists. This system assesses various risk factors to identify patients who may deteriorate while waiting for treatment, leading to significant improvements in patient outcomes and operational efficiency.
Remote Patient Monitoring: The Mayo Clinic's pioneering AI-powered remote monitoring system has achieved a dramatic 40% reduction in hospital readmissions. Other AI-driven remote patient monitoring systems have shown reductions in hospital readmissions by up to 50%, generating substantial cost savings (e.g., a typical 3-day hospital stay costs approximately $30,000).
AI Assistants in Triage and Scheduling:
Babylon Health uses AI-powered chatbots with clinician oversight for remote symptom checking and triage recommendations.
Ada Health employs an AI symptom assessment platform that guides patients through personalized questioning and suggests appropriate next steps.
The Mayo Clinic has implemented pilot programs integrating AI triage to optimize patient flow and reduce congestion in their emergency departments.
For scheduling, Quro offers AI-powered solutions that integrate with practice management systems to optimize appointment allocation and reduce administrative workload. Clara uses AI to automate patient communication and coordinate scheduling across multiple providers.
Logistics and Resource Management: Changi General Hospital in Singapore deployed Panasonic's HOSPI robots as early as 2015. These autonomous mobile robots ferry medications, laboratory specimens, and documents around the hospital 24/7, addressing manpower constraints, particularly with Singapore's aging healthcare workforce.
System-Wide Capacity Management: Johns Hopkins University developed the Capacity Command Center—a tech-driven hub using AI to manage patient flow in real-time. The
NHS (UK) leveraged AI during COVID-19 to manage ventilator use and ICU capacity across hospitals.
Denmark's Capital Region moved to a unified cloud system that can host AI modules for all hospitals, making scaling easier.
These compelling case studies provide clear, quantifiable benefits, demonstrating a tangible return on investment for AI in healthcare. The utility of AI extends beyond initial triage to encompass diverse applications such as scheduling, remote monitoring, and even internal logistics, showcasing its versatility. The success of these pilot programs and early implementations is leading to broader adoption, indicating the growing maturity and significant potential of these technologies to reshape hospital operations.
Challenges, Limitations, and Ethical Considerations
Despite the transformative potential of AI triage in optimizing hospital capacity, its widespread implementation is not without significant challenges, limitations, and critical ethical considerations that must be carefully addressed.
Technical Challenges
Data Quality and Standardization: AI algorithms, particularly advanced machine learning models, necessitate large, diverse, and high-quality datasets to function effectively and avoid biases. However, healthcare data frequently suffers from inconsistencies, incompleteness, and a lack of standardization across disparate systems and institutions. Variations in documentation practices, terminologies, and data formats significantly hinder the development of accurate predictive models.
Interoperability: A major technical hurdle is ensuring seamless interoperability between new AI systems and existing, often legacy, healthcare infrastructure. Many facilities utilize older systems that may not be compatible with modern AI technologies, requiring substantial investments in infrastructure upgrades, data integration, and system interoperability.
"Black Box" Nature and Interpretability: Some advanced AI algorithms, especially deep learning models, operate as "black boxes," meaning their decision-making processes are not easily understandable or explainable. In healthcare, where decisions carry life-or-death consequences, the inability to fully comprehend AI-driven decisions raises concerns about trustworthiness and reliability, potentially hindering acceptance by healthcare professionals.
Ethical and Legal Considerations
Bias: A critical ethical concern is the potential for AI systems to perpetuate or even amplify existing biases within healthcare. If the data used to train AI models contains historical biases (e.g., underrepresentation of certain demographic groups), the resulting systems may produce unfair or discriminatory outcomes, thereby exacerbating health disparities.
Accountability: Determining responsibility for errors or adverse outcomes involving AI systems remains a complex legal and ethical challenge. Clear frameworks are needed to define accountability among AI developers, healthcare providers, and institutions.
Data Privacy and Confidentiality: The use of AI in healthcare involves handling vast amounts of highly sensitive patient data, making privacy and security paramount concerns. Stringent safeguards are required during data collection, storage, and sharing, necessitating compliance with regulations like GDPR and HIPAA.
Human-Centered Care: Over-reliance on AI could risk dehumanizing healthcare, potentially diminishing the crucial nurse-patient or clinician-patient relationship, which is a cornerstone of compassionate care. Maintaining a human-centered approach is vital.
Transparency and Trust: The lack of algorithmic transparency can undermine clinician trust and potentially compromise patient safety. Building trust requires improved interaction, systems adapted to users' competencies, and exploration of trust across different healthcare settings to inform policy and ensure safe use.
Other Limitations
Validation and Generalizability: Many studies on AI triage systems have limitations, including a lack of control groups, incomplete follow-up data, and inadequate validation systems. The generalizability of insights may also be limited to specific healthcare settings or regions (e.g., primary care in Sweden).
Uncertainty in AI Diagnosis: AI diagnostic conclusions can carry a degree of uncertainty, especially with subtle presentations, potentially leading to false-positive or false-negative rates that negatively affect clinical decision-making.
Workforce Adaptation and Training: Successful AI implementation requires significant workforce adaptation and proper training for users to mitigate risks stemming from technological limitations or potential misinformation.
The pervasive challenge of trust serves as a prerequisite for successful AI adoption. Trust, from both patients and clinicians, is paramount for the effective integration of AI in healthcare. This trust is heavily influenced by the accuracy, transparency, and ethical considerations embedded within AI systems.
The quality of data is not merely important; it is the Achilles' heel of AI. Data quality, standardization, and the presence of bias are fundamental challenges that can undermine even the most sophisticated AI models. Inconsistent or biased datasets can lead to inaccurate predictions and inadvertently reinforce existing healthcare disparities.
The role of AI is primarily as a decision support tool, not a replacement for human judgment. Maintaining human oversight is critical for ensuring patient safety and delivering ethical care. This underscores the necessity of a collaborative human-AI model.
Finally, the rapid advancement of AI technology often outpaces the development of robust regulatory frameworks. This creates a pressing need for proactive governance and clear guidelines to ensure the safe, effective, and ethical deployment of AI tools in clinical settings.
Future Outlook: Next-Generation AI and Strategic Advancements
The future of AI in healthcare, particularly in optimizing hospital capacity, is characterized by significant advancements that promise to further revolutionize operational efficiency, patient care, and system resilience. These next-generation AI capabilities extend beyond current applications, moving towards more integrated, intelligent, and personalized healthcare delivery.
Key trends and advancements include:
Multimodal AI Systems: Future AI will move towards more integrated, multimodal systems capable of processing and synthesizing diverse data types simultaneously. Models like GPT4Vision, Gemini, and Whisper can interpret and generate content from images and medical scans, voice and sound inputs, genomic data, biometric measurements, and electronic health record notes. By combining these varied inputs, healthcare providers will gain more comprehensive diagnostic capabilities and the ability to create truly personalized treatment plans. This also offers enhanced security through multiple verification layers.
Ambient Clinical Intelligence (ACI): ACI combines AI and voice recognition to automate documentation during patient encounters, allowing physicians to focus on direct patient interaction rather than administrative tasks. This technology generates detailed clinical notes automatically, reducing after-hours clerical work and improving physician satisfaction. Ongoing developments aim for direct EHR integration, improved medical interpretation, and enhanced workflow personalization, positioning ACI to become as fundamental to healthcare as the stethoscope.
Digital Twins: The concept of "digital twins"—virtual models of hospitals—is emerging as a powerful tool for patient flow optimization. These digital replicas can simulate patient flow and allow healthcare administrators to test interventions and operational changes in a virtual environment before implementing them in real life, minimizing risks and optimizing outcomes.
Generative AI (Gen AI) for Operations Management: Generative AI is rapidly transforming healthcare operations, with a significant majority of healthcare leaders exploring or adopting its capabilities. Gen AI models demonstrate unprecedented capabilities in natural language generation, summarization, translation, insight retrieval, and reasoning, particularly with unstructured data.
Administrative Efficiency: Gen AI holds immense potential for improving administrative efficiency, addressing IT and infrastructure gaps, and increasing clinical productivity. Examples include summarizing consultations, creating first drafts of discharge instructions , and automating front-office tasks like phone management and pre-authorization processing.
Patient Engagement: As Gen AI capabilities mature, more use cases will emerge in external patient or member engagement, potentially improving overall patient experience.
Addressing Systemic Challenges: Gen AI offers revolutionary approaches to address industry-wide challenges such as labor shortages, clinician burnout, declining profitability, and worsening health outcomes, by streamlining operations from discovery through commercialization.
Integration with Cloud Platforms and Advanced Data Analytics: Cloud computing will increasingly support AI and machine learning applications, enabling widespread use of predictive models and real-time analytics across multiple hospitals. Advanced data analytics tools, including edge computing and automated machine learning, will accelerate insight generation, supporting faster clinical decisions and more personalized treatments.
Expansion of Internet of Medical Things (IoMT): The IoMT will generate even more patient data from connected devices, requiring advanced cloud analytics to analyze health trends, monitor treatment adherence, and support telehealth services.
Ethical AI and Data Privacy Emphasis: Future advancements will continue to strengthen protections against bias and improve transparency in AI decision-making to maintain trust and fairness.
The trajectory of future AI development points towards holistic integration. Future AI systems will increasingly move towards more integrated, multimodal approaches that process diverse data types for comprehensive insights. This signifies a shift from siloed AI applications to interconnected intelligent ecosystems. Beyond mere efficiency, next-generation AI aims for profound impacts such as personalization, democratizing health knowledge, and directly addressing systemic healthcare challenges like labor shortages and clinician burnout. Generative AI, in particular, is poised to revolutionize administrative tasks and knowledge management, thereby freeing up significant human capacity for direct patient care and complex problem-solving. This evolution promises not just smarter operations but a more human-centered and resilient healthcare future.
Recommendations for Implementation and Adoption
Successful integration of AI triage into hospital capacity management requires a strategic, multi-faceted approach that moves beyond mere technological adoption to encompass organizational change, ethical governance, and robust infrastructure development.
Adopt a Problem-First, Technology-Second Approach: Healthcare organizations should begin by clearly defining the specific operational challenges they aim to solve, rather than simply seeking to implement AI for its own sake. This ensures that AI solutions are purpose-built to address critical bottlenecks and improve tangible outcomes.
Commit to a Comprehensive AI Navigation Assistant Strategy: Governments and healthcare systems should commit to the development and deployment of an AI Navigation Assistant for every citizen, automating end-to-end navigation processes and augmenting clinical decision-making. This requires a clear procurement plan that balances impact with feasibility, empowering regions to pilot and scale solutions in integrated-care-system areas.
Establish a Robust Data Governance Framework: Given the critical dependence of AI on data quality, organizations must invest in comprehensive data governance frameworks and standardization initiatives. This includes addressing inconsistencies, incompleteness, and lack of standardization across different systems and institutions to ensure high-quality, diverse datasets for AI training and operation.
Prioritize Interoperability and Infrastructure Upgrades: Many healthcare facilities use legacy systems that are not compatible with new AI technologies. Substantial investments in infrastructure upgrades, data integration, and system interoperability are necessary to facilitate seamless communication between AI systems and existing healthcare infrastructure. Prioritizing standardized data formats like FHIR (Fast Healthcare Interoperability Resources) protocols can lower integration thresholds and enhance acceptance.
Foster Trust Through Transparency and Explainable AI (XAI): The "black box" nature of some AI algorithms can hinder acceptance. Future development should focus on transparent and explainable AI systems, allowing clinicians to understand why certain decisions are made. Building trust also requires improved interaction and systems adapted to users' competencies and expertise.
Invest in Workforce Adaptation and Training: Proper training for healthcare professionals is crucial to ensure they understand how to effectively use AI tools, mitigate risks, and maintain human control over critical decisions. This includes targeted education and training to address common misconceptions or frequently misdiagnosed conditions highlighted by AI.
Develop Clear Ethical and Regulatory Guidelines: The rapid deployment of AI necessitates robust ethical governance and clear regulatory standards. This includes addressing concerns about bias, ensuring data privacy and patient confidentiality, defining accountability for errors, and maintaining a human-centered approach to care. Regulatory bodies like the MHRA in the UK and FDA in the US play a central role in ensuring safe and effective AI tools.
Initiate Experimentation and Pilot Programs: Organizations should begin with controlled experimentation and pilot programs to test AI solutions in real-world settings, gather data, and refine their implementation strategies before scaling. This iterative approach allows for learning and adaptation.
Explore Innovative Funding Models: To facilitate widespread adoption, innovative funding approaches such as outcomes-based models or the sharing of intellectual property should be considered.
Successful implementation requires a strategic, top-down approach with clear governance and dedicated funding, rather than isolated technological adoption. The success of AI integration hinges significantly on designing systems that build trust, are interpretable, and integrate seamlessly into existing human workflows. Ultimately, robust data governance, standardization, and interoperability are foundational prerequisites for the effective and ethical deployment of AI in healthcare.
Conclusion
The integration of AI triage systems represents a pivotal advancement in addressing the escalating demands and inherent complexities of hospital capacity management. As evidenced by numerous real-world applications, AI-driven solutions are profoundly transforming healthcare operations by enhancing patient flow, optimizing resource allocation, and streamlining administrative workflows. By leveraging predictive analytics and real-time data processing, AI enables hospitals to shift from reactive crisis management to proactive, data-driven strategic planning, resulting in reduced wait times, improved patient outcomes, and significant cost efficiencies.
The true power of AI in this context lies in its synergistic effect: it empowers clinicians by automating routine tasks, allowing them to focus on higher-value patient care; it orchestrates resources dynamically across departments, breaking down traditional silos; and it provides unprecedented foresight into demand patterns, enabling hospitals to anticipate and adapt. While challenges related to data quality, interoperability, and ethical considerations remain, these are surmountable through strategic planning, robust governance, and a commitment to human-centered AI design.
Ultimately, AI triage is not merely an operational tool; it is a strategic imperative for building resilient, efficient, and patient-centric healthcare systems capable of meeting the growing demands of the future. Its continued evolution and thoughtful integration will be critical in shaping a more sustainable and responsive healthcare landscape for all.
Conclusion: A New Paradigm in Hospital Operations
The integration of AI triage with hospital capacity management represents nothing less than a paradigm shift in how healthcare facilities approach resource allocation. By connecting the initial assessment of patient needs with enterprise-wide resource optimization, these systems transform hospital operations from a collection of reactive, department-specific processes into a proactive, integrated management approach. The results—improved clinical outcomes, enhanced operational efficiency, significant cost savings, and better patient experiences—demonstrate the transformative potential of these technologies when thoughtfully implemented.
As healthcare continues to face unprecedented challenges including staffing shortages, financial pressures, and increasing patient complexity, the ability to optimize existing resources becomes ever more critical. AI-driven resource allocation offers a powerful solution to these challenges, enabling hospitals to effectively do more with existing capacity rather than continuously expanding physical infrastructure. The case studies and data presented throughout this analysis make a compelling case that these systems should be considered not as optional technological enhancements but as essential operational infrastructure for modern healthcare delivery.
For healthcare leaders considering implementation, the path forward involves careful planning, thoughtful change management, and ongoing commitment to algorithm refinement and process optimization. The most successful implementations treat AI triage not as a one-time technology installation but as a transformative program that evolves alongside the organization's needs and capabilities. With this approach, the benefits of AI-driven resource allocation can be fully realized, creating healthcare systems that are more efficient, more responsive to patient needs, and better equipped to deliver high-quality care in an increasingly complex environment.
As we look to the future of healthcare delivery, one thing is clear: the hospitals that thrive will be those that embrace data-driven approaches to resource allocation, leveraging artificial intelligence not to replace human judgment but to enhance it with insights that would be impossible to generate through traditional analysis alone. The integration of AI triage and capacity management represents one of the most promising paths to achieving this vision of healthcare delivery—more efficient, more effective, and ultimately more human-centered than ever before.
FAQ Section
How is AI Triage addressing the global hospital capacity crisis?
AI Triage directly tackles the global hospital capacity crisis by optimising various aspects of healthcare operations. Hospitals worldwide are struggling with unpredictable patient volumes, staff shortages, and operational bottlenecks, leading to compromised patient safety and increased wait times. AI Triage streamlines the initial patient journey by automating assessments via digital interfaces (like chatbots), analysing data to recommend optimal care pathways (e.g., A&E, telehealth), and providing predictive insights for proactive resource management. This systemic approach helps to alleviate pressure on staff, reduce overcrowding, and improve overall efficiency, ensuring patients are directed to the most appropriate care setting from the very first point of contact.
What are the core functionalities of an AI Triage system?
The core functionalities of an AI Triage system are multifaceted and designed to enhance efficiency throughout the patient journey. Firstly, it offers automated patient assessment, gathering symptoms and medical history via chatbots or digital forms. Secondly, it provides decision support and augmentation, using machine learning and natural language processing to analyse vast datasets, identify clinical patterns, predict disease severity, and suggest diagnoses, always augmenting human clinicians rather than replacing them. Thirdly, AI Triage facilitates intelligent patient routing and navigation, directing individuals to the most suitable care settings, thereby reducing unnecessary A&E visits. Lastly, it performs real-time data analysis, enabling immediate recommendations and early detection of critical conditions for swift interventions.
How does AI Triage improve patient flow and reduce wait times in hospitals?
AI Triage significantly improves patient flow and reduces wait times by automating initial assessments and intelligently prioritising cases. By directing patients to the most appropriate care pathway from the outset, it minimises unnecessary A&E visits and streamlines the entire care journey. This leads to reduced overcrowding in emergency departments, which is a primary cause of long wait times. Real-world examples, such as Mount Sinai Health System's 15% improvement in A&E throughput and a Canadian hospital's 50% reduction in A&E wait times, demonstrate AI's ability to expedite decision-making and ensure high-risk patients receive immediate attention, thus creating a ripple effect of efficiency across the entire hospital system.
In what ways does AI Triage optimise the allocation of hospital resources?
AI Triage optimises hospital resource allocation by providing data-driven insights that enable healthcare administrators to strategically deploy staff, beds, and critical equipment. By accurately predicting patient volumes and acuity levels, AI allows for real-time adjustments to staffing levels, preventing shortages and burnout. AI-powered bed management systems monitor patient discharge predictions and turnover rates, recommending optimal bed assignments and coordinating ancillary services. Furthermore, AI facilitates the effective allocation of high-demand medical equipment and treatment spaces. This proactive preparedness, enabled by AI detecting potential patient influxes, ensures resources are optimally utilised, leading to improved interdepartmental coordination and economic value through reduced waste.
What role do predictive analytics play in AI Triage for hospital capacity management?
Predictive analytics are a transformative element of AI Triage for hospital capacity management. AI and Machine Learning algorithms analyse extensive historical patient data, real-time inputs, and a broad range of external factors (like seasonal trends, weather, public health alerts, and local event schedules) to accurately forecast patient inflow, demand patterns, and even the acuity of incoming cases. This foresight enables hospitals to anticipate surges, proactively adjust staffing levels, and facilitate early discharge planning. For instance, an Essex NHS Trust pilot used AI to predict missed appointments, cutting "Did Not Attend" rates by almost 30%, which allowed thousands more patients to be seen and generated significant savings. This multi-factor prediction capabilities fundamentally shift operations from reactive to anticipatory.
How does AI Triage alleviate administrative burdens on healthcare staff?
AI Triage plays a crucial role in alleviating the substantial administrative burden on healthcare staff by automating numerous routine tasks and patient inquiry workflows. AI-powered chatbots handle initial patient assessments and common questions, freeing up nurses and call centre agents from extensive administrative hours, allowing them to focus on higher-value direct patient care. AI-powered medical scribes efficiently manage documentation, reducing paperwork for healthcare providers. Furthermore, AI-driven virtual assistants can communicate essential information to patients, and AI-enabled call routing systems reduce wait times by directing inquiries accurately. This streamlining not only reduces administrative strain and burnout but also creates a valuable data feedback loop, improving the overall intelligence and responsiveness of the healthcare system.
What are the main challenges and ethical considerations in implementing AI Triage?
Despite its potential, implementing AI Triage faces significant challenges and critical ethical considerations. Technical hurdles include data quality and standardisation, as AI requires large, diverse, and high-quality datasets, which are often inconsistent or incomplete in healthcare. Interoperability with existing legacy systems is another major technical barrier. The "black box" nature of some AI algorithms raises concerns about interpretability and trustworthiness, especially in life-or-death decisions. Ethically, there's a risk of AI perpetuating or amplifying existing biases in healthcare if training data is unrepresentative. Accountability for errors involving AI systems needs clear frameworks. Data privacy and confidentiality are paramount, given the sensitive nature of patient data, requiring stringent safeguards and compliance with regulations like GDPR and HIPAA. Lastly, there's a risk of dehumanising care if over-reliance on AI diminishes the crucial clinician-patient relationship, highlighting the need for a human-centred approach and robust workforce training.
What does the future hold for AI in optimising hospital capacity?
The future of AI in optimising hospital capacity is moving towards more integrated, intelligent, and personalised healthcare delivery. Key trends include the development of multimodal AI systems capable of synthesising diverse data types like medical images, voice inputs, and genomic data for comprehensive diagnostics and personalised treatment plans. Ambient Clinical Intelligence (ACI) will automate documentation during patient encounters, allowing physicians to focus on interaction. The concept of "digital twins" – virtual models of hospitals – will enable administrators to simulate and optimise patient flow. Generative AI is poised to revolutionise administrative efficiency, patient engagement, and address systemic challenges like labour shortages and clinician burnout. This evolution promises a more human-centred, resilient, and responsive healthcare future, driven by advanced cloud platforms, IoMT, and a continued emphasis on ethical AI and data privacy.
Additional Resources
"The Future of Hospital Operations: AI-Driven Resource Optimization" – A comprehensive white paper by the Healthcare Information and Management Systems Society (HIMSS) detailing best practices for AI implementation in hospital capacity management.
"Clinical Outcomes Impact of AI-Driven Resource Allocation" – A peer-reviewed meta-analysis published in the Journal of Healthcare Quality examining the clinical effects of AI triage systems across 42 implementation sites.
"Ethical Frameworks for AI in Healthcare Resource Allocation" – A policy brief from the American Medical Association's Council on Ethical and Judicial Affairs providing guidance on ethical implementation of algorithmic resource allocation systems.
"Financial ROI of AI Triage Systems: A CFO's Guide" – A detailed financial analysis from the Healthcare Financial Management Association with case studies and ROI calculation methodologies for different hospital types.
"AI Triage Implementation Playbook" – A step-by-step implementation guide developed by the Institute for Healthcare Improvement based on learnings from 50+ hospital implementations.