Explainable AI in Triage: Making Black Box Decisions Transparent
The topic of explainable AI (XAI) in triage, particularly in making black box decisions transparent for clinicians, is a critical area of exploration. Let's begin by understanding the significance of this topic and then delve into the various aspects that make it essential for modern healthcare.
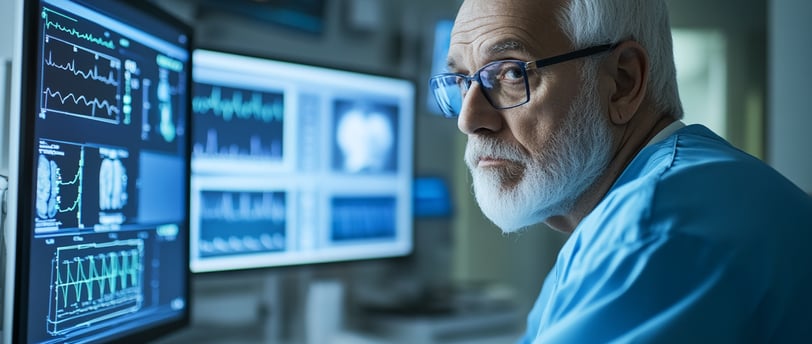
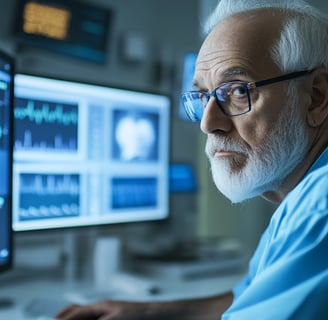
Imagine a bustling emergency room where every second counts. Clinicians must quickly assess and prioritise patients based on the severity of their conditions. Traditionally, this triage process relies on the experience and judgment of healthcare professionals. However, with the advent of artificial intelligence (AI), there's a promising new tool in the clinician's arsenal: AI-driven triage systems. These systems can analyse vast amounts of data and provide recommendations to help clinicians make more informed decisions. But there's a catch—many of these AI systems are "black boxes," meaning their decision-making processes are opaque and difficult to understand. This lack of transparency can be a significant barrier to the adoption and trust of AI in healthcare, especially in high-stakes environments like emergency rooms.
The Need for Explainable AI in Triage
The Black Box Problem
AI systems, particularly those based on machine learning (ML) and deep learning (DL), have revolutionised many industries, including healthcare. In triage, AI can analyse patient data, such as vital signs, medical history, and symptoms, to predict the likelihood of adverse outcomes and recommend appropriate care paths. However, the complexity of these models often makes it difficult for clinicians to understand how the AI arrived at its recommendations. This lack of explainability is known as the "black box" problem123.
Impact on Clinical Decision-Making
The black-box nature of AI can have several implications for clinical decision-making. First, clinicians may hesitate to trust and act on recommendations from a system they don't understand. This can lead to underutilisation of AI tools, even if they can potentially improve patient outcomes123. Second, the lack of transparency can make it challenging to identify and correct biases in the AI system, which could result in unfair or inaccurate recommendations. Finally, in cases where the AI's recommendation conflicts with a clinician's judgment, the inability to explain the AI's reasoning can make it difficult to resolve disagreements and reach a consensus on the best course of action3.
Ethical and Legal Considerations
Using black-box AI in healthcare also raises ethical and legal questions. For instance, if an AI system makes a recommendation that leads to a poor patient outcome, who is responsible? The clinician who acted on the recommendation, the developer of the AI system, or the healthcare institution that implemented the system? The lack of explainability can make it difficult to assign responsibility and accountability, which is a critical issue in medicine45. Additionally, opaque AI systems may conflict with the principle of informed consent, as patients have the right to understand the basis for their treatment recommendations67.
Strategies to Enhance Explainability
Developing Explainable AI Models
One approach to addressing the black box problem is to develop AI models that are inherently explainable. This can be achieved using more straightforward, more interpretable models, such as decision trees or linear regression, instead of complex DL models. However, there is often a trade-off between explainability and performance, as simpler models may not capture the nuances of complex medical data and more sophisticated models28.
Post-Hoc Explanation Methods
Another strategy is to use post-hoc explanation methods, which aim to explain the decisions an already trained black box model makes. These methods can provide insights into which features were most important in making a prediction, even if the model itself is complex. Examples of posthoc explanation methods include LIME (Local Interpretable Model-agnostic Explanations) and SHAP (Shapley Additive explanations), which can highlight the key factors that influenced an AI's recommendation23.
Incorporating Clinician Feedback
Incorporating clinician feedback into developing and refining AI models can also enhance explainability. By involving clinicians in the process, developers can ensure that the models align with clinical knowledge and practices, making the recommendations more understandable and trustworthy. This collaborative approach can also help identify and address biases in the AI system, improving its fairness and accuracy27.
Case Studies and Examples
AI in Cardiovascular Imaging
In cardiovascular imaging, AI has shown promise in improving diagnostic accuracy and clinical efficiency. For example, AI-powered systems can quantify coronary artery stenosis from CT angiography in real-time, which would be time-consuming and challenging for human experts. However, the black-box nature of these AI models poses significant challenges, as clinicians may not fully understand or trust the AI’s outputs, impacting patient care1.
AI in Emergency Medicine
In emergency medicine, AI can enhance triage systems, improving diagnostic accuracy and optimising clinical care. However, the lack of explainability can lead to trust issues among clinicians, who may perceive AI as a black box. To address this, explainable AI (XAI) is crucial. XAI helps clinicians understand the rationale behind AI outputs, enhancing trust and supporting clinical decision-making7.
Implementing Explainable AI in Practice
Training and Education
To effectively integrate explainable AI into clinical practice, adequate training and education for healthcare professionals are essential. This includes teaching them how to interpret and use AI recommendations and communicate the limitations and uncertainties of AI systems to patients. Healthcare institutions can foster a culture of trust and responsible use of AI27 by equipping clinicians with the necessary knowledge and skills.
Regulatory Frameworks and Guidelines
Establishing standardised regulatory frameworks and guidelines for developing and using AI in healthcare is crucial. These frameworks should address issues such as transparency, accountability, and fairness, ensuring that AI systems are developed and implemented to prioritise patient safety and ethical considerations. By fostering interdisciplinary collaboration and continuous monitoring, the medical community can ensure the responsible integration of AI into clinical practice123.
Conclusion
Integrating AI in triage can revolutionise emergency care by improving diagnostic accuracy, reducing triage time, and enhancing patient outcomes. However, the black-box nature of many AI systems poses significant challenges to their adoption and trust in clinical settings. Explainable AI offers a solution by providing precise and understandable explanations for AI recommendations, enhancing clinicians' trust and facilitating ethical and legal accountability. By implementing strategies such as developing inherently explainable models, using post-hoc explanation methods, and incorporating clinician feedback, healthcare institutions can foster a culture of trust and responsible use of AI. As we move forward, it is crucial to establish regulatory frameworks and guidelines that prioritise transparency, accountability, and fairness, ensuring that AI is developed and implemented to benefit both patients and clinicians.
FAQ Section
What is explainable AI in triage?
Explainable AI (XAI) in triage refers to AI systems that provide clear and understandable explanations for their recommendations, making it easier for clinicians to trust and act on the AI's outputs123.
Why is explainability important in AI-driven triage systems?
Explainability is crucial because it enhances clinicians' trust in AI recommendations, allows for better identification and correction of biases, and facilitates ethical and legal accountability123.
What are some strategies to enhance explainability in AI?
Strategies include developing inherently explainable models, using post-hoc explanation methods, and incorporating clinician feedback into the development process283.
How can clinicians be trained to use explainable AI effectively?
Clinicians can be trained through educational programs to interpret AI recommendations, communicate limitations to patients, and understand the ethical considerations of AI use283.
What are the ethical considerations of using AI in healthcare?
Ethical considerations include assigning responsibility for AI-driven decisions, ensuring informed consent, and maintaining patient autonomy and trust123.
How does explainable AI impact patient outcomes?
Explainable AI can improve patient outcomes by enhancing diagnostic accuracy, reducing triage time, and increasing patient satisfaction and trust in the care process123.
What are the challenges of implementing explainable AI in healthcare?
Challenges include balancing explainability with performance, addressing biases in AI systems, and ensuring that AI recommendations align with clinical knowledge and practices123.
How can regulatory frameworks support the use of explainable AI in healthcare?
Regulatory frameworks can support explainable AI by addressing transparency, accountability, and fairness and fostering interdisciplinary collaboration and continuous monitoring123.
What is the role of clinician feedback in developing explainable AI?
Clinician feedback ensures that AI models align with clinical knowledge and practices, making the recommendations more understandable and trustworthy283.
How does explainable AI address the black box problem?
Explainable AI addresses the black box problem by providing precise and understandable explanations for AI recommendations, making it easier for clinicians to trust and act on the AI's outputs123.
Additional Resources
For readers interested in exploring this topic further, here are some reliable sources: