Digital Health Equity: Ensuring AI Triage Serves All
Explore the critical role of AI in triage systems and the importance of ensuring digital health equity across all communities. Discover how biases, data gaps, and accessibility challenges can be addressed to create more inclusive healthcare solutions.
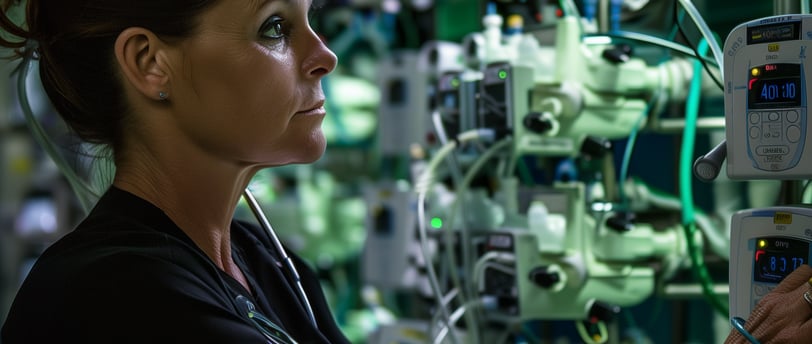
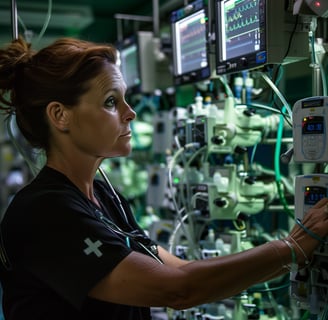
Imagine a world where the quality of healthcare you receive is determined not by your zip code, skin colour, or economic status but by the simple fact that you are human. This is the promise of AI-driven triage systems, which aim to revolutionise healthcare by providing efficient, accurate, and equitable care. However, the reality is often more complex. As we delve into the intricacies of AI in triage, we must confront the challenges of digital health equity and ensure that these innovative systems serve all communities equally.
In this article, we will explore the current landscape of AI in triage, the barriers to equitable healthcare, and the strategies needed to overcome these obstacles. From addressing biases in algorithms to ensuring data inclusivity and enhancing accessibility, we will provide a comprehensive overview of what it takes to achieve digital health equity.
The Role of AI in Triage Systems
Revolutionising Healthcare Delivery
AI-driven triage systems are transforming healthcare by streamlining patient assessment and prioritisation. These systems use machine learning algorithms to analyse patient data, symptoms, and medical history to determine the urgency and severity of a patient's condition. For instance, hospitals like Massachusetts General Hospital have implemented AI triage systems to reduce wait times and improve patient outcomes.
Benefits and Challenges
The benefits of AI in triage are numerous. They include increased efficiency, reduced workload for healthcare professionals, and the potential for more accurate diagnoses. However, these benefits are not without their challenges. One of the most significant concerns is the potential for bias in AI algorithms, which can lead to disparities in healthcare delivery.
Case Study: AI Triage in Action
To show the potential of AI triage, I'd like you to look at the example of Cleveland Clinic, which has integrated AI into its emergency department. The system analyses patient data in real time, allowing for quicker and more accurate triage decisions. This has led to a 30% reduction in wait times and improved patient satisfaction.
Addressing Bias in AI Algorithms
Sources of Bias
Bias in AI algorithms can stem from various sources, including biased training data, proxies for protected characteristics, and feedback loops that reinforce existing disparities. For example, if an AI system is trained on data that predominantly represents one demographic group, it may perform poorly for other groups.
Mitigating Bias
It is crucial to ensure diverse and representative training data to mitigate bias. This involves collecting data from various populations and continuously monitoring the algorithm's performance across different demographic groups. Additionally, implementing fairness constraints and regular audits can help identify and address biases.
Ethical Considerations
The ethical implications of bias in AI triage systems are profound. Disparities in healthcare delivery can exacerbate health inequalities and worsen outcomes for marginalised communities. It is essential to prioritise ethical considerations when developing and deploying AI triage systems.
Ensuring Data Inclusivity
The Importance of Representative Data
Representative data is the cornerstone of inclusive AI systems. Ensuring that the data used to train AI algorithms is diverse and representative of the entire population is crucial for accurate and equitable healthcare delivery. This includes data on race, ethnicity, gender, age, and socioeconomic status.
Strategies for Data Collection
To achieve data inclusivity, healthcare organisations must adopt proactive data collection strategies. These include partnering with community organisations to reach underserved populations, using inclusive language in data collection forms, and ensuring that data is collected in a culturally sensitive manner.
Overcoming Data Gaps
Data gaps can occur when specific populations are underrepresented in healthcare data. To overcome these gaps, it is essential to invest in community outreach and engagement and leverage alternative data sources, such as social determinants of health data.
Enhancing Accessibility
Digital Divide and Healthcare Access
The digital divide—the gap between those with access to digital technologies and those without—is a significant barrier to equitable healthcare. AI triage systems that rely on digital platforms may inadvertently exclude individuals who lack access to the Internet or digital devices.
Designing for Accessibility
To enhance accessibility, AI triage systems must be designed with inclusivity in mind. This includes ensuring that systems are compatible with assistive technologies, providing multilingual support, and offering offline or low-tech alternatives for individuals without internet access.
Community Engagement
Engaging with communities is crucial for understanding their unique needs and challenges. By involving community members in designing and implementing AI triage systems, healthcare organisations can ensure these systems are accessible and effective for all.
Conclusion
In conclusion, AI-driven triage systems hold immense potential to revolutionise healthcare delivery. However, achieving digital health equity requires a concerted effort to address biases, ensure data inclusivity, and enhance accessibility. By prioritising these aspects, we can create healthcare solutions that serve all communities equally, regardless of their background or circumstances.
As we progress, healthcare organisations, policymakers, and technology developers must work together to build a more equitable healthcare system. The future of healthcare is in our hands, and it is our responsibility to ensure that it is fair, inclusive, and accessible to all.
FAQ Section
What is AI triage?
AI triage is the use of artificial intelligence to prioritise patients based on the severity of their condition.
How does AI triage improve healthcare delivery?
AI triage improves healthcare delivery by increasing efficiency, reducing workload for healthcare professionals, and providing more accurate diagnoses.
What are the challenges of AI triage?
Challenges include bias in algorithms, data inclusivity, and accessibility issues.
How can bias in AI algorithms be mitigated?
Bias can be mitigated through diverse training data, fairness constraints, and regular audits.
Why is representative data important?
Representative data ensures that AI systems are accurate and equitable for all populations.
What strategies can be used to overcome data gaps?
Strategies include community outreach, inclusive language, and leveraging alternative data sources.
How does the digital divide affect healthcare access?
The digital divide can exclude individuals who lack access to digital technologies from AI triage systems.
What are some ways to enhance accessibility?
Ways to enhance accessibility include designing for assistive technologies, providing multilingual support, and offering offline alternatives.
Why is community engagement critical?
Community engagement ensures that AI triage systems are accessible and effective for all communities.
What are the ethical considerations of AI triage?
Ethical considerations include ensuring fairness, transparency, and accountability in AI systems.
Additional Resources
World Health Organization (WHO) - For global health statistics and initiatives.
Centers for Disease Control and Prevention (CDC) - For public health information and resources.
Healthcare Information and Management Systems Society (HIMSS) - For insights into healthcare IT and digital health.