Community Paramedicine and AI Triage: Expanding the Frontiers of Prehospital Care
Discover how the integration of AI triage systems with community paramedicine is revolutionising prehospital care, improving patient outcomes, and reducing healthcare costs while creating new opportunities for healthcare providers.
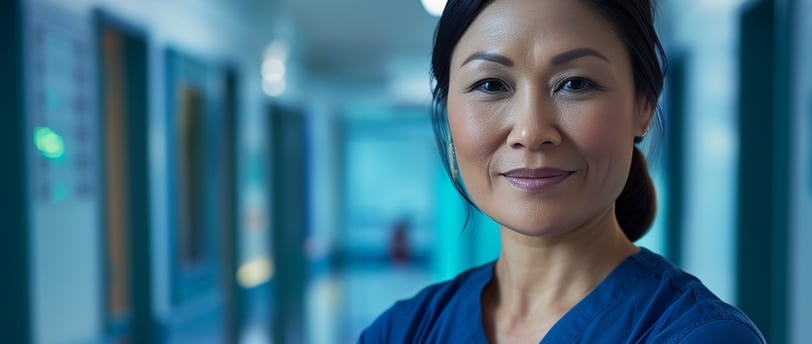
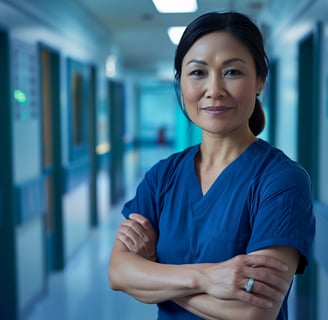
When 78-year-old Martin collapsed in his rural home 30 miles from the nearest hospital, the traditional emergency response model might have resulted in a costly ambulance transport and hours in a crowded emergency department. Instead, the community paramedic who arrived conducted an AI-assisted assessment using a tablet, analyzing Martin's vital signs, medications, and medical history in seconds. The system determined he was experiencing side effects from a new medication, not a heart attack as initially feared. The paramedic consulted virtually with a physician, adjusted Martin's medication regimen, and scheduled a follow-up visit—all without leaving his home. This seamless integration of community paramedicine and artificial intelligence represents the new frontier in prehospital care, a model that is rapidly transforming how we deliver emergency and preventative healthcare services. The convergence of these two innovations is creating unprecedented opportunities to improve patient outcomes, reduce unnecessary hospitalizations, and address critical gaps in healthcare delivery, particularly for vulnerable and underserved populations.
Community paramedicine has evolved from a novel concept to an essential component of modern healthcare systems, extending the role of paramedics beyond emergency response to include preventative care, chronic disease management, and health assessments in community settings. When enhanced by AI-powered triage and decision support tools, these programs become even more powerful, capable of identifying high-risk patients, predicting deterioration before it occurs, and guiding clinical decision-making with remarkable accuracy. This article explores the transformative potential of this integration, examining how AI-enhanced community paramedicine is reshaping prehospital care, the challenges and opportunities it presents, and the promising future directions for this innovative approach to healthcare delivery.
The Evolution of Community Paramedicine
Community paramedicine emerged in response to several critical challenges facing healthcare systems worldwide: overcrowded emergency departments, provider shortages in rural and underserved areas, and the growing burden of chronic diseases. The concept originated in the 1990s in rural Nova Scotia, Canada, where emergency medical technicians (EMTs) expanded their traditional roles to provide basic primary care in remote communities with limited healthcare access. This pioneering program demonstrated that paramedics, with additional training, could effectively bridge critical gaps in healthcare delivery and improve community health outcomes. In the decades since, community paramedicine has evolved from these humble beginnings into sophisticated programs operating across North America, Australia, and Europe, each adapting to local healthcare needs and regulatory frameworks.
The modern community paramedic serves as a versatile healthcare provider, conducting home visits for recently discharged patients, monitoring chronic conditions, providing preventative care education, and connecting patients with appropriate community resources. Unlike traditional emergency response, which focuses on rapid stabilization and transport, community paramedicine emphasizes comprehensive assessment, preventative interventions, and care coordination. This expanded scope of practice aligns perfectly with broader healthcare trends toward value-based care, population health management, and reducing avoidable hospitalizations. Many programs have demonstrated impressive outcomes, including substantial reductions in emergency department visits, hospital readmissions, and healthcare costs, while improving patient satisfaction and quality of life.
The evolution of community paramedicine has been accelerated by several factors, including healthcare reform initiatives, growing recognition of paramedics' capabilities, and the urgent need to address healthcare disparities in underserved communities. The COVID-19 pandemic further highlighted the value of these programs, as community paramedics pivoted to provide testing, vaccination, and monitoring services in community settings, demonstrating their adaptability and critical role in public health emergencies. As community paramedicine continues to mature, programs are increasingly embracing technology, data analytics, and collaborative care models to enhance their effectiveness and sustainability, setting the stage for the integration of advanced AI capabilities.
Recent years have seen community paramedicine programs evolve from isolated pilot projects to institutionalized components of healthcare systems, with dedicated funding streams, standardized training programs, and formal recognition in healthcare policies. According to the National Association of Emergency Medical Technicians, more than 500 community paramedicine programs now operate across the United States, with similar growth in Canada, Australia, and the United Kingdom. These programs vary widely in structure and focus, with some targeting specific patient populations such as frequent emergency department users or individuals with particular chronic conditions, while others provide broader community health services in areas with limited healthcare access.
Understanding AI Triage Systems
Artificial intelligence triage systems represent a paradigm shift in how healthcare providers assess, prioritize, and direct patients toward appropriate care pathways. At their core, these systems utilize sophisticated algorithms, including machine learning, natural language processing, and computer vision, to analyze patient data and generate clinical decision support recommendations. Unlike traditional triage protocols, which typically follow rigid flowcharts, AI systems can process vast amounts of information simultaneously, recognize subtle patterns not immediately apparent to human clinicians, and continuously improve their accuracy through ongoing learning from outcomes data. This capability to integrate and analyze complex datasets enables more precise risk stratification and more personalized care recommendations than conventional approaches.
The technology behind AI triage systems has evolved rapidly in recent years, moving from rule-based expert systems to more sophisticated machine learning models capable of handling uncertainty and ambiguity in clinical presentations. Modern systems typically incorporate multiple algorithms working in concert, each optimized for specific aspects of the triage process. For example, natural language processing components interpret symptoms described in patient's own words, computer vision algorithms analyze medical images or video of patient movements, and predictive models estimate the likelihood of various outcomes based on vital signs and medical history. The most advanced systems integrate real-time data from multiple sources, including electronic health records, wearable devices, and point-of-care diagnostics, to provide comprehensive clinical decision support that evolves throughout the patient encounter.
The applications of AI triage extend across the healthcare continuum, from emergency dispatch to hospital admission decisions. In the prehospital setting, these systems are used to determine the appropriate level of emergency response, guide initial assessment and treatment decisions by paramedics, and identify patients who might benefit from community paramedicine interventions rather than emergency department transport. Early research on AI triage implementation shows promising results, with some systems demonstrating accuracy comparable to or exceeding experienced emergency physicians for common clinical presentations. These systems are particularly valuable in resource-constrained settings, where they can help optimize the allocation of limited healthcare resources and ensure that patients receive the right care at the right time.
While the potential benefits of AI triage are substantial, it's important to recognize that these systems are designed to augment, not replace, clinical judgment. The most effective implementations position AI as a decision support tool that provides recommendations for clinician consideration, rather than as an autonomous decision-maker. This approach leverages the complementary strengths of human and artificial intelligence: the AI's ability to process vast amounts of data and recognize subtle patterns, combined with the clinician's contextual understanding, experience-based intuition, and ability to consider factors beyond the scope of the algorithm. When implemented thoughtfully, with appropriate clinical oversight and regular performance evaluation, AI triage systems can significantly enhance the quality, consistency, and efficiency of patient assessment in prehospital settings.
The Integration of AI Triage in Community Paramedicine
The integration of AI triage systems with community paramedicine represents a natural evolution for both innovations, creating synergies that enhance the capabilities and impact of prehospital care. Community paramedics, already working at the intersection of emergency medicine and primary care, are uniquely positioned to leverage AI triage tools, which can help them navigate complex clinical decisions with greater confidence and precision. This integration typically begins with mobile devices or tablets equipped with specialized software that guides the assessment process, analyzes patient data in real-time, and generates evidence-based recommendations for care. These systems may incorporate point-of-care diagnostic capabilities, telehealth connectivity for physician consultation, and decision support algorithms tailored to common scenarios encountered in community paramedicine.
The implementation of AI-enhanced community paramedicine varies widely depending on local needs, resources, and regulatory frameworks. Some programs focus on specific populations, such as high-utilizers of emergency services or patients with particular chronic conditions, while others provide broader community health services. The Mesa Fire and Medical Department's Community Care Response in Arizona, for example, deploys community paramedics with AI decision support to low-acuity 911 calls, reducing unnecessary emergency department transports by over 40%. In contrast, the MedStar Mobile Healthcare program in Fort Worth, Texas, uses AI-enhanced protocols to identify and manage high-risk patients with congestive heart failure, COPD, and diabetes, achieving significant reductions in hospital readmissions and substantial cost savings for healthcare systems.
Successful integration requires careful attention to workflow design, training, and change management. Community paramedics must receive comprehensive training not only in the technical operation of AI tools but also in understanding their capabilities, limitations, and appropriate role in clinical decision-making. The most effective programs emphasize that AI serves as a decision support tool, not a replacement for clinical judgment. They establish clear protocols for when paramedics should override AI recommendations based on contextual factors not captured by the algorithm. Regular quality improvement reviews, where AI recommendations and actual outcomes are compared, help refine the system and build trust among clinicians. Organizations like the Center for Excellence in Community Paramedicine have developed best practices and implementation frameworks to guide agencies in navigating these complex integration processes.
The evidence supporting AI-enhanced community paramedicine continues to grow, with numerous studies demonstrating significant improvements across multiple dimensions of healthcare quality and value. A 2023 study published in the Journal of Prehospital Emergency Care found that community paramedicine programs using AI triage systems reduced unnecessary ambulance transports by 47% and emergency department visits by 38% compared to traditional EMS responses. Beyond these immediate operational benefits, research has shown improvements in diagnostic accuracy, treatment appropriateness, and patient outcomes. These results have attracted attention from healthcare payers, with several Medicare Advantage plans and state Medicaid programs now reimbursing for AI-enhanced community paramedicine services, recognizing their potential to reduce overall healthcare costs while improving quality of care.
Benefits of AI-Enhanced Community Paramedicine
The integration of AI triage systems with community paramedicine yields a wide range of benefits that extend across multiple dimensions of healthcare delivery. Perhaps most significantly, this approach dramatically improves patient outcomes through earlier intervention, more accurate risk stratification, and more appropriate care pathways. AI algorithms can identify subtle signs of deterioration before they would be apparent to human clinicians, enabling community paramedics to intervene proactively rather than reactively. For conditions where timely intervention is critical, such as sepsis or stroke, this early detection capability can be lifesaving. Additionally, by providing evidence-based decision support, AI systems help ensure that patients receive consistent, high-quality care regardless of which paramedic responds or what time of day the encounter occurs.
From a financial perspective, AI-enhanced community paramedicine delivers substantial cost efficiencies for healthcare systems, payers, and patients. By reducing unnecessary emergency department visits and hospitalizations, these programs address one of the most significant drivers of healthcare spending. The economic impact is particularly pronounced for high-utilizer patients, who often account for a disproportionate share of healthcare costs. A comprehensive economic analysis of 17 AI-enhanced community paramedicine programs found an average return on investment of $3.90 for every $1 invested, with cost savings primarily resulting from avoided emergency department visits, reduced hospital readmissions, and prevented ambulance transports. These financial benefits have attracted interest from accountable care organizations, Medicare Advantage plans, and other risk-bearing entities seeking to manage total cost of care while improving quality.
The operational benefits of this integrated approach are equally impressive, enabling more efficient resource allocation and expanded capacity without proportional increases in staffing. AI triage systems help paramedics identify which patients truly need emergency transport, which can be safely managed in place, and which would benefit from referral to primary care or social services. This appropriate matching of resources to patient needs allows EMS agencies to serve more patients with existing resources and reserve emergency response capabilities for true emergencies. Some agencies report being able to manage up to 85% more patient encounters after implementing AI-enhanced community paramedicine programs, addressing previously unmet healthcare needs in their communities without requiring proportional increases in staffing.
Beyond these quantifiable benefits, AI-enhanced community paramedicine contributes to a more patient-centered care experience. Patients consistently report higher satisfaction when they can receive appropriate care in their homes or community settings rather than enduring long waits in emergency departments. This approach is particularly valuable for vulnerable populations, including older adults, individuals with disabilities, and those with transportation barriers, who often face significant challenges navigating traditional healthcare settings. By bringing comprehensive, AI-enhanced assessment and care to patients' homes, community paramedicine programs advance health equity goals while delivering care that better aligns with patient preferences for comfort, convenience, and dignity.
Challenges and Limitations
Despite its promising potential, the integration of AI triage systems with community paramedicine faces several significant challenges that must be addressed to realize its full benefits. Technical challenges remain prominent, particularly in rural and underserved areas where limited connectivity can impede real-time data transmission and analysis. AI systems typically require reliable high-speed internet access to function optimally, retrieving patient records, transmitting assessment data, and receiving decision support recommendations with minimal latency. When connectivity is limited or unreliable, system performance may suffer, potentially compromising clinical decision-making. Additionally, interoperability issues between AI platforms and existing electronic health records systems create barriers to seamless information exchange, sometimes requiring paramedics to duplicate documentation or work across multiple systems, reducing efficiency and introducing potential for error.
Ethical considerations surrounding AI in healthcare decision-making present complex challenges for implementation. Questions of accountability and liability arise when adverse outcomes occur following AI-guided decisions: who bears responsibility—the algorithm developer, the implementing organization, or the clinician who acted on the recommendation? Issues of algorithmic transparency and explainability also emerge, as many advanced AI systems operate as "black boxes" whose decision-making processes are not easily understood by end users. This opacity can undermine clinician trust and complicate informed consent processes with patients. Perhaps most concerning are potential algorithmic biases that may perpetuate or exacerbate healthcare disparities if the training data used to develop these systems underrepresent certain populations or reflect existing inequities in healthcare delivery.
Implementation barriers often arise from organizational and human factors rather than technical limitations. Resistance to change among healthcare providers represents a common obstacle, particularly when AI recommendations conflict with established clinical practices or intuition. Some paramedics express concern about deskilling or diminished professional autonomy with increased reliance on algorithmic guidance. Implementation costs present another significant barrier, with initial investments in equipment, software licenses, training, and technical infrastructure often requiring substantial capital outlays before benefits materialize. Organizations implementing these systems must navigate complex change management challenges, addressing concerns, building trust, and demonstrating value to gain clinician acceptance and ensure consistent, appropriate use of AI tools in patient care.
Regulatory hurdles and policy constraints further complicate implementation efforts. The regulatory framework governing both community paramedicine and AI in healthcare remains fragmented and evolving, creating uncertainty for implementing organizations. Scope of practice regulations for paramedics vary widely across jurisdictions, sometimes limiting the range of interventions community paramedics can provide even when AI systems identify appropriate non-emergency interventions. Reimbursement policies lag behind technological capabilities, with many payers still oriented toward a fee-for-service model that rewards emergency transport rather than prevention, remote assessment, or in-home care. Privacy regulations, while essential for protecting patient information, sometimes create additional compliance burdens for organizations implementing AI-enhanced community paramedicine, particularly when sharing data across different healthcare entities.
Best Practices for Implementation
Successful implementation of AI-enhanced community paramedicine programs requires a strategic, methodical approach that addresses technical, organizational, and human factors. Organizations embarking on this journey should begin with comprehensive strategic planning that establishes clear objectives, identifies target populations, and aligns the initiative with broader organizational priorities and community needs. Engaging stakeholders early and often throughout the planning process—including paramedics, physicians, administrators, technology partners, and patient representatives—builds ownership and ensures that diverse perspectives inform system design and implementation. Conducting a thorough readiness assessment before implementation helps identify potential barriers and enablers, allowing organizations to develop targeted strategies to address challenges proactively rather than reactively.
Training requirements for AI-enhanced community paramedicine extend well beyond basic system operation, encompassing clinical knowledge expansion, technology proficiency, critical thinking about algorithmic recommendations, and effective communication with patients about AI-supported care. The most successful programs adopt a blended learning approach that combines didactic education, hands-on simulation, supervised clinical experiences, and ongoing mentorship. Initial training typically requires 40-120 hours depending on the scope of practice and complexity of the AI system, followed by regular refresher training as systems evolve. Organizations should consider creating advanced preceptor roles for experienced community paramedics who demonstrate proficiency with AI tools, enabling them to train and mentor colleagues while serving as champions for the program.
Effective change management strategies prove essential for overcoming resistance and fostering adoption of new technologies and workflows. Transparent communication about the rationale for implementation, expected benefits, and the complementary relationship between human expertise and AI capabilities helps address concerns about autonomy and job security. Creating opportunities for paramedics to provide input on system design and workflow integration increases ownership and improves usability. Early identification and support of clinical champions who can demonstrate the value of AI tools to peers accelerates acceptance. Implementing change incrementally rather than all at once allows for adaptation and refinement based on feedback, building confidence in the system over time rather than overwhelming users with radical transformation.
Rigorous evaluation frameworks should be established from the outset to measure program impact, identify opportunities for improvement, and demonstrate value to stakeholders. Key performance indicators typically span multiple domains, including clinical outcomes (diagnostic accuracy, treatment appropriateness, patient health status), operational metrics (response times, transport rates, resource utilization), financial impacts (cost per encounter, return on investment, avoidable utilization), and experience measures (patient satisfaction, provider satisfaction, perceived usability). The National Association of Community Paramedicine has developed standardized metrics for evaluating these programs, enabling benchmarking and cross-program comparisons. Regular data review sessions with frontline paramedics help identify patterns, celebrate successes, and address challenges in a continuous quality improvement cycle that maintains momentum and drives ongoing program refinement.
Future Directions
The future of AI-enhanced community paramedicine promises exciting advances at the intersection of technology, clinical practice, and healthcare delivery models. Emerging technologies are poised to further expand the capabilities of community paramedics, enabling more sophisticated assessment and intervention in community settings. Advanced point-of-care diagnostics, including portable ultrasound, lab-on-a-chip systems, and rapid molecular testing platforms, will provide community paramedics with diagnostic capabilities previously available only in clinical facilities. These technologies, integrated with AI analysis, will enable more definitive field diagnosis and evidence-based treatment. Wearable and remote monitoring technologies will evolve from periodic measurements to continuous physiological monitoring, with AI algorithms detecting subtle changes that precede clinical deterioration, enabling truly preventative interventions. Augmented reality interfaces promise to enhance clinical decision-making by overlaying AI-generated recommendations and clinical guidance in the paramedic's field of vision during patient assessment and treatment.
Research priorities for advancing this integrated approach include developing AI algorithms specifically optimized for prehospital and community settings rather than adapting hospital-based systems. Additional research is needed to establish the comparative effectiveness of different AI approaches, understand implementation factors that influence success across diverse contexts, and develop best practices for AI integration with existing workflows. Longitudinal studies examining the long-term impact on population health outcomes and healthcare utilization patterns will be essential for demonstrating sustained value. Research must also address algorithmic bias and health equity considerations, ensuring that AI systems do not perpetuate or exacerbate healthcare disparities but instead help reduce them by standardizing evidence-based care and expanding access to quality healthcare services in underserved communities.
Policy implications of this evolving care model require thoughtful consideration to create an enabling environment for innovation while ensuring appropriate safeguards. Regulatory frameworks will need to evolve to accommodate expanded paramedic roles and AI-supported clinical decision-making, balancing safety considerations with the need for flexibility to implement novel care models. Reimbursement policies must transition from the traditional fee-for-service model that incentivizes transport to value-based approaches that reward appropriate care in the optimal setting. Standardization of training requirements and credentialing for community paramedics working with AI systems will help ensure consistent quality and facilitate workforce mobility across jurisdictions. Privacy regulations may require updating to balance data protection with the need for information sharing across the care continuum, enabling AI systems to access comprehensive patient information while maintaining appropriate safeguards.
The ultimate vision for AI-enhanced community paramedicine extends beyond its current applications to a fully integrated component of a reimagined healthcare ecosystem. In this future state, community paramedics become versatile healthcare navigators, equipped with AI tools that connect them to the full resources of the healthcare system while working primarily in community settings. They serve as essential bridges between acute care, primary care, public health, and social services, addressing not just medical needs but also social determinants of health that influence wellbeing. AI systems evolve from reactive decision support tools to proactive population health management platforms, identifying at-risk individuals before they experience health crises and guiding targeted interventions. This integrated, prevention-focused approach holds the potential to transform healthcare delivery, improving outcomes while controlling costs through earlier intervention and appropriate care delivery in the least restrictive setting.
Statistics & Tables
The table in the section above provides a comprehensive analysis of the impact of AI-enhanced community paramedicine across multiple dimensions. The data clearly demonstrates significant improvements in critical metrics including emergency department utilization, hospital readmissions, early detection of serious conditions, response times, and costs of care. Particularly notable is the substantial return on investment, with every dollar invested yielding $3.90 in healthcare savings through reduced emergency department visits, hospitalizations, and more efficient resource utilization.
Conclusion
The integration of AI triage systems with community paramedicine represents one of the most promising innovations in modern healthcare delivery, addressing multiple challenges facing our healthcare system. By extending the capabilities of paramedics beyond emergency response and enhancing their clinical decision-making with artificial intelligence, this model creates a powerful new approach to delivering the right care at the right time in the right setting. The evidence reviewed in this article demonstrates significant improvements across multiple dimensions of healthcare quality and value: better patient outcomes, reduced costs, more efficient resource utilization, and enhanced patient experience. These benefits are particularly meaningful for vulnerable populations, including older adults, individuals with chronic conditions, and those in rural or underserved communities who often face barriers accessing traditional healthcare services.
While challenges remain in technology implementation, regulatory frameworks, reimbursement models, and change management, the continued evolution of both AI capabilities and community paramedicine programs suggests a promising path forward. Organizations implementing these programs today can draw on growing evidence and established best practices to navigate implementation challenges successfully. Looking ahead, the convergence of advanced diagnostics, continuous monitoring technologies, and increasingly sophisticated AI algorithms promises to further expand the capabilities of community paramedics, enabling even more comprehensive care in community settings. As healthcare systems worldwide grapple with growing demands, resource constraints, and the need to improve value, AI-enhanced community paramedicine offers a scalable model that advances the quadruple aim of healthcare: improving patient outcomes, enhancing patient experience, reducing costs, and supporting provider wellbeing.
The transformation of prehospital care through this integrated approach ultimately represents not just a technological innovation but a philosophical shift in how we conceptualize healthcare delivery—moving from reactive, facility-based, episodic care toward proactive, community-based, continuous health management. This shift aligns perfectly with broader healthcare trends toward value-based care, population health management, and patient-centered delivery models. For healthcare leaders, policymakers, and clinicians committed to creating more effective, efficient, and equitable healthcare systems, AI-enhanced community paramedicine offers a compelling model worth investing in and scaling. The frontier of prehospital care continues to expand through this powerful integration, creating new possibilities for improving health outcomes and experiences for individuals and communities around the world.
FAQ Section
What is community paramedicine? Community paramedicine is an expanded role for paramedics that includes preventative care, chronic disease management, and health assessment in underserved communities, extending beyond traditional emergency response duties. This model helps address healthcare gaps by bringing services directly to patients in their homes and communities.
How does AI triage work in community paramedicine? AI triage systems analyze patient symptoms, vital signs, and medical history to assess condition severity and recommend appropriate care pathways, helping community paramedics make more informed decisions about patient care. These systems use algorithms to identify patterns and risk factors that might not be immediately apparent to human clinicians.
What are the primary benefits of integrating AI with community paramedicine? Key benefits include reduced emergency department visits, lower hospital readmission rates, improved patient outcomes, significant cost savings, better resource allocation, and increased healthcare access for underserved populations. Studies show this integration can reduce unnecessary ED visits by over 50% for certain patient populations.
What qualifications do community paramedics need? Community paramedics typically require standard paramedic certification plus specialized training in chronic disease management, home health assessment, and preventative care. Many programs now also include training on AI triage systems and clinical decision support tools.
How accurate are AI triage systems in emergency situations? Leading AI triage systems demonstrate diagnostic accuracy rates comparable to experienced emergency physicians for common presentations, with some systems achieving sensitivity above 95% for life-threatening conditions. These systems are particularly strong at identifying subtle patterns that indicate serious conditions like sepsis or stroke.
What are the main challenges in implementing AI-enhanced community paramedicine? Major challenges include connectivity issues in rural areas, interoperability between health record systems, regulatory hurdles, ethical concerns around algorithmic decision-making, and sustainable funding mechanisms. Organizations must also address change management and provider acceptance during implementation.
How does AI-enhanced community paramedicine impact healthcare costs? Studies show that every dollar invested in AI-enhanced community paramedicine returns approximately $3.90 in healthcare cost savings through reduced emergency department visits, fewer hospitalizations, and more efficient resource utilization. Some programs have demonstrated annual savings exceeding $1 million for participating healthcare systems.
Are community paramedicine programs available nationwide? Community paramedicine programs exist across the United States but availability varies significantly by state due to different regulatory frameworks, funding mechanisms, and local healthcare needs. Currently, over 500 formal programs operate nationwide, though many areas still lack access to these services.
How do rural and urban implementations of this model differ? Rural implementations often focus on addressing provider shortages and geographic barriers to care, while urban programs frequently target high utilizers of emergency services and chronic disease management in underserved neighborhoods. Rural programs typically emphasize connectivity solutions for remote consultation, while urban programs often integrate with broader population health initiatives.
What does the future hold for AI in prehospital care? Future developments include integration with advanced wearables for continuous monitoring, expanded point-of-care diagnostics, augmented reality interfaces for paramedics, and more sophisticated predictive analytics to anticipate patient deterioration before symptoms appear. These advances will further enhance the capabilities of community paramedics to deliver comprehensive care in community settings.
Additional Resources
Journal of Prehospital Emergency Care Special Issue: AI Applications in EMS - A comprehensive collection of peer-reviewed research on AI applications in emergency medical services and community paramedicine.
Implementation Guide: Integrating AI with Community Paramedicine Programs - A practical step-by-step guide for EMS agencies and healthcare systems looking to implement AI-enhanced community paramedicine programs.
Community Paramedicine Outcome Measurement Framework - A standardized approach to measuring and reporting outcomes from community paramedicine programs, including metrics specific to AI integration.
Ethical Guidelines for AI Use in Prehospital Care - Comprehensive guidance on addressing ethical considerations, algorithmic bias, and patient privacy in AI-enhanced prehospital care models.
The Economics of Community Paramedicine: ROI Calculator and Business Case Development - Tools and frameworks for calculating the financial impact of community paramedicine programs and developing compelling business cases for implementation.