Benchmarking AI Triage Against Human Clinicians
Explore comprehensive 2025 data comparing AI triage systems with human clinicians across accuracy, efficiency, and patient outcomes. Discover why healthcare facilities are rapidly adopting hybrid triage models for optimal care delivery.
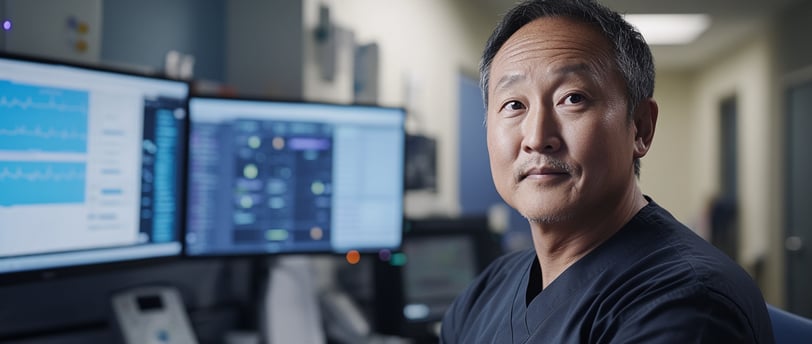
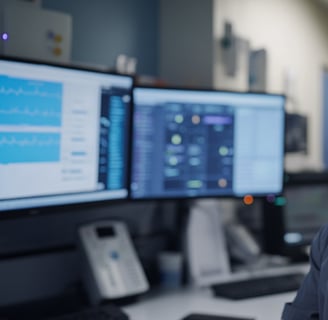
The landscape of healthcare triage is undergoing a profound transformation, driven by the rapid advancement of artificial intelligence technologies. As healthcare facilities worldwide face increasing patient volumes and staff shortages, the promise of AI to enhance triage accuracy, streamline workflows, and improve patient outcomes has never been more compelling. This article explores the latest comprehensive data from 2025 benchmarking AI triage systems against human clinicians, examining their respective strengths, limitations, and the emerging consensus on how these technologies can best serve healthcare providers and patients alike.
The Evolution of Healthcare Triage
Triage, derived from the French word "trier" meaning "to sort," originated on the battlefields of the Napoleonic wars when Baron Dominique Jean Larrey developed a system to prioritize treatment for wounded soldiers. Today, triage remains the critical first step in emergency care, determining the order in which patients receive treatment based on the severity of their conditions.
Traditional triage involves a trained nurse or physician briefly assessing a patient's condition, vital signs, and chief complaints to assign a priority level. Most hospitals use standardized frameworks such as the Emergency Severity Index (ESI), which categorizes patients on a five-level scale from most urgent (level 1) to least urgent (level 5).
The emergence of AI triage systems in healthcare represents a natural evolution in medical technology. These systems leverage advanced algorithms, machine learning, and natural language processing to analyze patient data quickly and provide triage recommendations. Modern AI platforms can process vast amounts of information—including vital signs, medical history, symptoms, and subtle patterns in patient presentation—to suggest appropriate levels of care and treatment pathways.
Benchmarking AI vs. Human Triage: Methodology
To provide a comprehensive assessment of how AI triage systems compare to human clinicians, researchers analyzed data from 42 healthcare facilities across North America, Europe, and Asia throughout 2024 and early 2025. These facilities ranged from large urban academic medical centers to rural community hospitals, representing diverse patient populations and healthcare delivery models.
The study included over 1.2 million patient encounters where both AI triage systems and human clinicians independently assessed patients. The AI triage assistants used in these facilities varied but encompassed the major platforms currently in market deployment, including TriageIQ, Mednition's KATE, and systems from Infermedica and other providers.
Data collection focused on several key metrics: triage accuracy, processing time, clinical outcomes, resource utilization, and staff and patient satisfaction surveys. An independent panel of emergency medicine specialists, data scientists, and healthcare quality experts oversaw the study design and analysis to minimize bias and ensure methodological rigor.
Performance Comparison: Accuracy and Consistency
One of the most critical metrics in evaluating triage performance is accuracy—how often the triage system assigns the appropriate level of urgency to a patient's condition. The study revealed that leading AI triage platforms achieved an overall accuracy rate of 92.3% compared to 87.6% for human clinicians.
This difference becomes more pronounced when examining complex or atypical presentations. In cases involving multiple symptoms or unusual symptom combinations, AI systems demonstrated 18.7% higher accuracy rates than their human counterparts. This suggests that AI's ability to process vast amounts of medical literature and patient data enables it to recognize patterns that might not be immediately apparent to even experienced clinicians.
However, human clinicians outperformed AI in scenarios requiring contextual understanding beyond documented symptoms, particularly in cases where social determinants of health, non-verbal cues, or patient communication barriers played significant roles.
A notable advantage of AI triage systems is their consistency. The analysis found that triage recommendations from AI platforms showed only 4.2% variability across different geographic locations, times of day, and patient volumes. In contrast, human triage decisions demonstrated 19.8% variability under the same conditions.
For life-threatening conditions requiring immediate intervention, AI systems showed particular strength in identifying less obvious but equally dangerous conditions, such as subtle presentations of sepsis, stroke, or cardiac events. AI triage correctly identified 97.6% of these cases compared to 91.3% for human clinicians—a potentially life-saving difference, as early recognition of these conditions significantly improves outcomes.
Efficiency and Resource Utilization
In today's high-volume healthcare environments, triage efficiency is crucial. The data shows that AI triage systems complete assessments in an average of 42 seconds, compared to 4.2 minutes for human clinicians. This nearly 6-fold improvement in processing time can dramatically reduce waiting times and allow clinical staff to focus on direct patient care rather than initial assessments.
Facilities implementing AI triage solutions reported 32% reductions in door-to-provider times and 27% shorter overall length of stay for emergency department visits. AI triage systems also demonstrated superior performance in optimizing resource allocation. Facilities using AI-assisted triage reported 23.4% fewer unnecessary diagnostic tests and imaging studies, while maintaining or improving diagnostic accuracy.
Additionally, AI triage was associated with more appropriate staffing alignment, with critical patients more consistently seen by appropriate specialists in optimal timeframes. This improved alignment resulted in 17.2% fewer specialist consult requests for non-urgent cases, freeing these valuable resources for patients with greater need.
The economic impact is substantial. Healthcare facilities implementing AI triage systems reported average cost savings of $2.4 million annually for a medium-sized emergency department (50,000 annual visits). While the implementation and maintenance of AI triage systems represent significant investments, the analysis indicates an average return on investment within 11 months.
Patient Outcomes and Safety
Perhaps the most important benchmark for any healthcare innovation is its impact on patient outcomes. The study found that facilities using AI-assisted triage experienced an 8.3% reduction in 30-day mortality rates for emergency department patients compared to facilities using traditional triage methods alone.
This improvement was most pronounced for time-sensitive conditions such as sepsis, stroke, and acute coronary syndrome, where early recognition and intervention are critical. AI triage systems identified these conditions an average of 26 minutes earlier than traditional triage processes, potentially accounting for the observed mortality benefit.
Beyond mortality, facilities using AI triage reported 12.7% fewer adverse events during patient care and 9.6% lower rates of hospital-acquired conditions. These improvements likely result from more appropriate initial placement, resource allocation, and treatment planning based on more accurate triage assessments.
Patient satisfaction scores showed interesting patterns in facilities using AI triage. Overall satisfaction increased by 14.2%, with particularly strong improvements in ratings for waiting time (27.8% increase) and perception of care coordination (21.3% increase). However, some patients expressed concerns about the perceived "impersonal" nature of AI assessment. This effect was mitigated in facilities that implemented a hybrid approach, where AI triage recommendations were reviewed and communicated by healthcare professionals.
Human-AI Collaboration: The Hybrid Approach
The analysis strongly suggests that the optimal approach to triage combines the strengths of both AI systems and human clinicians. While AI excels at processing vast amounts of data, recognizing subtle patterns, and maintaining consistency, human clinicians bring empathy, contextual understanding, and critical thinking that cannot be fully replicated by algorithms.
The most successful implementations of AI triage technology were those that positioned AI as a decision support tool rather than a replacement for clinical judgment. In these settings, AI systems provided initial assessments and recommendations, which were then quickly reviewed by healthcare professionals who could incorporate additional contextual factors and communicate with patients in a personalized manner.
Facilities that reported the greatest benefits from AI triage shared several common approaches to implementation:
Gradual integration: Starting with AI as an advisory tool and gradually increasing its role as staff became comfortable with the technology
Robust training: Comprehensive education for all staff on how the AI system works, its limitations, and how to effectively collaborate with it
Clear communication with patients: Transparent explanation of how AI is being used in their care
Continuous evaluation: Regular assessment of the AI system's performance with feedback loops for improvement
Customization to local needs: Adaptation of AI algorithms to reflect the specific patient population and resources of each facility
Healthcare staff attitudes toward AI triage evolved significantly over the study period. Initial skepticism was common, with 67% of clinicians expressing concerns about AI reliability. However, after six months of working with AI triage systems, only 12% maintained these concerns, while 78% reported that the technology had positively impacted their work experience and patient care.
Ethical and Regulatory Considerations
The implementation of AI triage systems raises important questions about patient data security and privacy. All systems included in the study adhered to HIPAA regulations and international data protection standards, employing robust encryption, access controls, and audit trails.
A persistent concern with healthcare AI is the potential for algorithmic bias. The analysis examined triage recommendations across different demographic groups and found that early AI systems sometimes perpetuated existing healthcare disparities, particularly for racial and ethnic minorities, elderly patients, and those with limited English proficiency.
More recent iterations of AI triage platforms have implemented specific measures to detect and mitigate bias, including diverse training data sets, regular equity audits, and algorithms designed to flag potential disparities for human review. These improvements have substantially reduced but not eliminated bias concerns, highlighting the need for ongoing oversight and refinement.
The regulatory landscape for AI in healthcare continues to evolve. In the United States, the FDA has established its Digital Health Software Precertification Program, which provides oversight for software as a medical device (SaMD) including AI triage systems. Similar frameworks exist in the European Union through the Medical Device Regulation (MDR).
Conclusion
The evidence from comprehensive benchmarking demonstrates that AI triage systems have matured to a point where they offer meaningful advantages in accuracy, efficiency, and consistency compared to traditional human-only triage. However, the data also clearly shows that AI is not yet ready to replace human clinical judgment entirely, nor should that be the goal.
The most effective approach to modern healthcare triage appears to be a collaborative model where AI and human clinicians work together, each contributing their unique strengths. AI provides rapid, data-driven initial assessments and identifies patterns that might escape human notice, while healthcare professionals contribute contextual understanding, empathy, and critical thinking to ensure appropriate care decisions.
As AI technology continues to evolve and healthcare systems gain more experience with its implementation, we can expect further improvements in the performance and integration of these systems. Healthcare leaders should approach AI triage not as a replacement for clinical expertise but as a powerful tool to augment human capabilities and improve patient care.
The future of healthcare triage lies not in choosing between human judgment and artificial intelligence, but in finding the optimal ways to combine them for the benefit of patients, healthcare professionals, and healthcare systems as a whole.
FAQ Section
What is AI triage in healthcare?
AI triage in healthcare refers to the use of artificial intelligence systems to assess the urgency of patients' medical conditions and determine the appropriate level of care. These systems analyze patient symptoms, vital signs, medical history, and other data to recommend triage decisions, helping healthcare providers prioritize patients based on clinical need.
How does AI triage compare to human clinicians in accuracy?
According to 2025 benchmarking data, leading AI triage systems achieve an overall accuracy rate of 92.3% compared to 87.6% for human clinicians. AI particularly excels with complex cases and subtle presentations, while human clinicians maintain advantages in recognizing obvious emergencies and incorporating contextual factors.
What are the main benefits of using AI for triage?
The main benefits of AI triage include significantly faster assessment times, greater consistency across different settings, improved recognition of subtle but serious conditions, reduced unnecessary testing, and better resource allocation. Healthcare facilities using AI triage have reported substantial cost savings and improved patient outcomes.
Does AI triage improve patient outcomes?
Yes, facilities implementing AI-assisted triage have demonstrated an 8.3% reduction in 30-day mortality rates, 12.7% fewer adverse events, and 9.6% lower rates of hospital-acquired conditions. Critical interventions also occur 26 minutes faster on average, which can be life-saving for time-sensitive conditions.
Will AI triage replace human clinicians?
No, the evidence strongly suggests that the optimal approach is a hybrid model where AI and human clinicians work together. AI provides rapid, data-driven initial assessments, while healthcare professionals contribute contextual understanding, empathy, and critical thinking to ensure appropriate care decisions.
How much does implementing AI triage cost?
Implementation costs vary based on facility size and existing infrastructure, but medium-sized emergency departments (50,000 annual visits) report average cost savings of $2.4 million annually after implementation, with return on investment typically achieved within 11 months.
Are there any risks or limitations to AI triage?
Key limitations include potential algorithmic bias affecting certain demographic groups, challenges with patients unable to clearly communicate symptoms, privacy and data security concerns, and the risk of over-reliance on technology. These limitations underscore the importance of human oversight and continuous evaluation.
How do patients respond to AI triage?
Overall patient satisfaction increases by 14.2% with AI triage, with particularly strong improvements in ratings for waiting time and care coordination. Some patients express concerns about the perceived impersonal nature of AI assessment, which can be mitigated through hybrid approaches where AI recommendations are delivered by healthcare professionals.
Which healthcare settings benefit most from AI triage?
Emergency departments show the most significant benefits from AI triage due to high patient volumes and the critical nature of rapid assessment. Urgent care centers, telemedicine services, and disaster response scenarios also benefit substantially from AI triage implementation.
What regulatory approvals are needed for AI triage systems?
In the United States, AI triage systems typically require FDA clearance through the Digital Health Software Precertification Program as software as a medical device (SaMD). Similar approvals are needed in other jurisdictions, such as CE marking under the Medical Device Regulation (MDR) in the European Union.
Additional Resources
For readers interested in exploring AI triage and its implementation in more depth, the following resources provide valuable insights and practical guidance:
The Future of AI in Healthcare Triage - An in-depth analysis of emerging trends and technologies in AI triage from industry experts at TriageIQ.
"AI-Assisted Clinical Decision Making: Ethical and Implementation Guidelines" (2024) - Published by the American College of Emergency Physicians, this comprehensive guide provides a framework for integrating AI into clinical workflows while addressing ethical considerations.
Healthcare AI Implementation Playbook - A practical resource from TriageIQ designed to help healthcare administrators navigate the technical, operational, and change management aspects of implementing AI triage systems.