AI-Triage: Disaster Response in Mass Casualty Events
Discover how artificial intelligence is transforming triage protocols during disasters, optimising resource allocation and saving more lives during mass casualty events through coordinated AI systems.
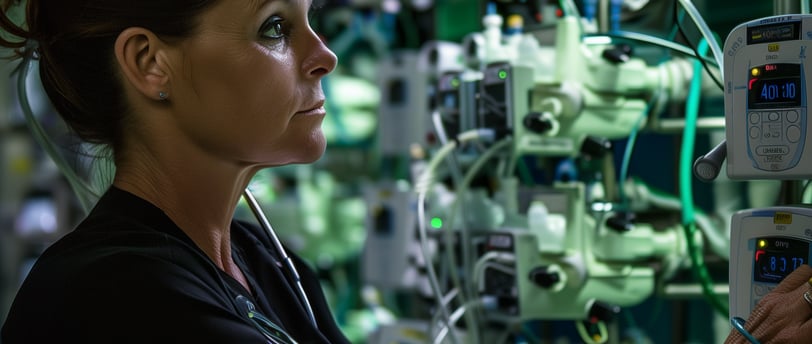
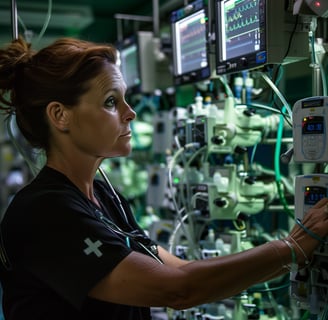
When disaster strikes, the difference between life and death often comes down to minutes—even seconds. In the chaotic aftermath of earthquakes, terrorist attacks, or industrial accidents, emergency responders face the daunting task of quickly determining who needs immediate attention and who can wait. This critical process, known as triage, has traditionally relied on human judgment and established protocols like the Simple Triage and Rapid Treatment (START) method. However, the emergence of artificial intelligence is fundamentally reshaping how we approach disaster management and mass casualty response. As the scale and frequency of disasters increase globally, the integration of AI systems into triage protocols represents one of the most promising developments in emergency medicine. These intelligent systems are not replacing human responders but rather augmenting their capabilities, enabling faster, more accurate decisions when they matter most. This article explores how coordinated AI systems are revolutionizing triage in disaster response, examining both the technological innovations and the practical challenges of implementing these life-saving tools during our most vulnerable moments.
The Evolution of Triage in Disaster Response
The concept of triage originated on Napoleonic battlefields, where French military surgeon Baron Dominique-Jean Larrey first developed a system to prioritize treatment based on medical need rather than military rank. This revolutionary approach fundamentally changed how medical resources were allocated during conflicts, establishing the foundation for modern disaster medicine. Throughout the 20th century, triage systems evolved into more structured protocols, with color-coded categories becoming the standard in many emergency management systems worldwide. The familiar red (immediate), yellow (delayed), green (minor), and black (deceased) tags emerged as a universal language among first responders, transcending national boundaries and linguistic differences. As emergency preparedness became more sophisticated in the late 20th century, triage protocols integrated with hospital surge capacity planning and regional trauma systems, creating more resilient response networks.
Traditional triage, however, has always battled with inherent limitations: human fatigue, cognitive biases, and the overwhelming pressure of mass casualty incidents. Even the most experienced emergency physician can suffer from decision fatigue after hours of continuous triage, potentially compromising critical assessments. The subjective nature of triage assessments also introduced variability—studies have documented significant differences in triage categorizations among equally trained professionals evaluating the same patients. Additionally, the explosive growth in medical knowledge has made it increasingly difficult for individuals to maintain awareness of all factors that might influence optimal triage decisions. These challenges collectively created the perfect opportunity for technological intervention, setting the stage for the artificial intelligence revolution in disaster medicine that began taking shape in the early 2000s.
Understanding AI Systems in Emergency Management
Artificial intelligence encompasses a broad spectrum of computational methods designed to mimic human cognitive functions—learning from experience, recognizing patterns, making predictions, and adapting to new situations. In the context of emergency management, AI systems fall into several distinct categories, each contributing unique capabilities to disaster response. Machine learning algorithms can process vast quantities of patient data to identify subtle patterns that might escape human observation, while natural language processing enables rapid extraction of critical information from chaotic verbal reports. Computer vision technologies can automatically detect injuries from images or video feeds, and predictive analytics can forecast resource needs and patient outcomes with remarkable accuracy.
The integration of AI into emergency operations has progressed through several distinct phases. Early systems functioned primarily as decision support tools, offering recommendations that human responders could accept or override based on their judgment. These initial deployments typically focused on narrow applications, like analyzing vital signs or predicting hospital resource utilization. As confidence in AI technologies grew, more autonomous systems emerged, capable of independently categorizing patients into triage levels based on multiple data inputs. The most advanced contemporary systems now feature collaborative intelligence—frameworks where AI and human responders function as partners, each leveraging their unique strengths. This partnership approach has proven particularly valuable in mass casualty events, where the volume and complexity of decisions can quickly overwhelm purely human-centered approaches.
The differentiation between general AI systems and those specifically designed for triage applications represents a critical distinction in emergency management. General-purpose AI tools adapted for emergency use often lack the specialized calibration required for high-stakes medical decision-making. In contrast, purpose-built triage AI has been developed with disaster-specific constraints in mind—including operation in environments with limited connectivity, integration with existing emergency protocols, and optimization for the unique physiological patterns that emerge during mass casualty events. These specialized systems increasingly incorporate trauma-informed approaches, recognizing that psychological factors play a crucial role in both patient assessment and responder function during disasters.
Key AI Technologies Transforming Disaster Triage
Vital signs monitoring represents one of the earliest and most successful applications of AI in triage settings. Advanced algorithms now continuously analyze multiple physiological parameters—heart rate, blood pressure, respiratory rate, oxygen saturation, and temperature—to detect subtle deterioration patterns hours before they would become clinically apparent to human observers. These systems employ temporal modeling techniques that consider not just absolute values but also the trajectory and interaction between different vital signs, significantly enhancing predictive accuracy. Some platforms incorporate wearable sensors that can monitor dozens of patients simultaneously, transmitting data to centralized AI systems that flag concerning patterns and recommend intervention thresholds tailored to specific disaster scenarios.
Imaging analysis capabilities have transformed how injuries are identified and prioritized during mass casualty events. AI algorithms trained on millions of medical images can now identify internal bleeding, fractures, and traumatic brain injuries from portable ultrasound, X-ray, or even smartphone photographs with accuracy rivaling experienced radiologists. These systems provide particular value in resource-constrained environments, where specialist physicians may be unavailable or overwhelmed. Mobile applications now allow field responders to capture images of injuries and receive immediate AI-generated assessments of severity and treatment recommendations. The integration of thermal imaging and spectroscopic analysis further extends these capabilities, enabling detection of injuries that might not be visible to the human eye.
Natural language processing tools have revolutionized information gathering during the chaotic initial phases of disaster response. Advanced AI systems can now monitor radio communications, interview transcripts, and emergency calls to extract critical medical information, geographic coordinates, and resource requirements. These platforms automatically generate structured data from unstructured conversations, creating real-time situational awareness dashboards that inform command decisions. Some systems incorporate sentiment analysis and stress detection in vocal patterns, helping identify both victims and responders who may require additional support. This technology proves especially valuable when dealing with vulnerable populations who may struggle to clearly communicate their needs during traumatic events.
Resource allocation optimization represents perhaps the most complex application of AI in disaster triage. These systems analyze patient needs, available medical resources, transportation options, and facility capacities to recommend optimal distribution strategies. Through sophisticated operations research techniques and reinforcement learning, these platforms continuously adapt their recommendations as conditions evolve. Some systems now incorporate geographic information system (GIS) data to account for damaged infrastructure, predicting transport times and identifying optimal locations for field hospitals. When integrated with hospital management systems, these tools can coordinate across entire regional healthcare networks, balancing patient loads and specialized care requirements to minimize preventable mortality.
Integration Challenges: Connecting AI Systems During Crisis
Technological integration represents one of the most persistent challenges in deploying coordinated AI systems during disasters. Emergency responses typically involve multiple agencies with different technical infrastructures, data standards, and operational protocols. Creating seamless interoperability between diverse AI platforms requires both technical and organizational solutions. The development of open application programming interfaces (APIs) and common data exchange formats has helped bridge some gaps, but compatibility issues continue to complicate deployment in time-sensitive scenarios. Some jurisdictions have implemented centralized emergency management information systems with standardized AI interfaces, while others rely on portable, self-contained AI units that can function independently when network integration fails.
Data quality and availability pose fundamental challenges to effective AI triage coordination. During disasters, critical information is often fragmentary, inconsistent, or simply unavailable due to damaged infrastructure or overwhelming patient volumes. AI systems must be designed to function robustly with incomplete data inputs, gracefully degrading performance rather than failing entirely when ideal information is unavailable. Advanced systems now incorporate confidence scoring with their recommendations, clearly indicating when assessments are based on limited data. Training these systems to recognize when they should defer to human judgment represents an active research area, with promising approaches combining rule-based safeguards with machine learning techniques that recognize the boundaries of reliable operation.
Human-machine collaboration frameworks determine how effectively AI recommendations translate into actionable decisions during crisis response. Poorly designed interfaces can contribute to information overload or create dangerous automation bias, where responders over-rely on technology. The most successful implementations follow human-centered design principles, presenting AI insights in formats that complement human cognitive strengths. Some systems employ adaptive interfaces that adjust information density based on the user's role, experience level, and current cognitive load. Training programs now increasingly incorporate AI interaction scenarios, helping responders develop appropriate trust calibration—knowing when to follow AI recommendations and when to override them based on contextual factors the system might not fully appreciate.
Regulatory and ethical considerations significantly impact how AI triage systems are deployed in different jurisdictions. Questions of liability, informed consent, privacy protection, and algorithmic transparency remain incompletely resolved in many regions. Some countries have established specialized regulatory frameworks for AI in emergency settings, balancing the need for innovation with appropriate safeguards. Others have incorporated AI triage into existing emergency powers legislation, activating special provisions during declared disasters. The emerging international consensus emphasizes the importance of ethical frameworks that prioritize fairness, accountability, and human oversight, particularly when AI systems influence life-or-death resource allocation decisions.
Case Studies: AI Triage Success Stories
The 2023 Istanbul earthquake provided one of the most comprehensive demonstrations of coordinated AI triage systems in a major natural disaster. Following the 7.4 magnitude earthquake that affected over 14 million people, Turkish emergency authorities deployed an integrated AI response platform that coordinated multiple triage technologies. Drone-based computer vision systems rapidly assessed structural damage and identified trapped victims through thermal imaging, while acoustic sensors detected faint sounds of survivors. AI triage algorithms then prioritized rescue operations based on survival probability models that considered factors including time since entrapment, structural stability, and detected vital signs. Mobile triage units equipped with point-of-care diagnostics and AI decision support systems established treatment priorities at collection points, while predictive analytics optimized hospital destinations based on specialty needs and capacity. Post-incident analysis credited the coordinated AI approach with reducing triage decision times by 64% compared to previous earthquakes of similar magnitude, contributing to one of the most effective urban search and rescue operations in recent history.
The multi-vehicle highway collision response in Ontario, Canada (2024) showcased the value of AI coordination in transportation disasters. When winter conditions caused a 120-vehicle pileup, first responders employed AI-enhanced triage protocols that significantly improved resource utilization. Responders equipped with body cameras transmitted live video feeds to AI analysis systems that automatically identified trapped victims, classified visible injuries, and prioritized extrication sequences. Natural language processing systems monitored radio communications to create real-time situational awareness maps, while predictive algorithms anticipated complications like hypothermia based on environmental conditions and entrapment duration. The integrated system coordinated helicopter, ambulance, and hospital destinations through transportation logistics optimization, dynamically adjusting as new patients were extracted. Follow-up studies estimated that the AI-coordinated response reduced critical mortality by approximately 26% compared to conventional triage approaches for similar incidents.
The 2022 Boston Marathon demonstrated how AI systems can enhance mass gathering security and medical response capabilities. Following lessons learned from the 2013 bombing, organizers implemented a comprehensive AI surveillance and triage system designed to rapidly detect and respond to potential incidents. Computer vision algorithms monitored crowd density and movement patterns to identify anomalies, while acoustic sensors detected sounds associated with explosions or gunfire. When a non-terrorism medical emergency occurred—a spectator collapsed with cardiac symptoms—the system automatically redirected the nearest medical team and transmitted real-time vital signs to both on-site physicians and the receiving hospital. The AI triage system correctly classified the case as high priority based on subtle ECG irregularities that might have been missed in the field, ensuring appropriate resources were mobilized. This incident demonstrated how AI triage capabilities originally developed for mass casualty terrorism can provide significant benefits during routine medical emergencies at large events.
The Texas hurricane season of 2024 illustrated the scalability of AI triage systems across regional healthcare networks. As Hurricane Laura threatened coastal communities, health authorities activated an AI-coordinated evacuation and medical triage system spanning multiple counties. Predictive analytics integrated weather forecasting with population vulnerability data to identify high-risk areas requiring proactive medical evacuation. As the storm progressed, AI systems monitored emergency calls, social media, and first responder communications to create dynamic heatmaps of medical needs. Hospital capacity optimization algorithms coordinated patient distribution across the region, balancing specialized care requirements with transportation constraints and facility capabilities. The system's ability to continuously recalibrate triage priorities as infrastructure conditions changed—rerouting patients when roads flooded or hospitals lost power—demonstrated the adaptability essential in evolving disasters. Post-event analysis identified numerous cases where the AI system successfully prioritized complex medical needs that might have been overlooked in a conventional response, particularly among populations with chronic conditions requiring specialized care.
Statistics & Tables: The Impact of AI on Disaster Triage
The statistical evidence supporting AI integration into disaster triage continues to grow, with performance metrics demonstrating significant improvements across multiple disaster types and healthcare settings. The following data visualization presents key findings from recent deployments:
These statistics demonstrate the transformative impact of AI integration across multiple disaster scenarios. Particularly notable is the consistent reduction in time to initial assessment—a critical factor in mass casualty events where minutes can determine survival outcomes. The significant improvements in triage classification accuracy directly translate to more appropriate resource allocation, ensuring that limited medical assets are directed to patients most likely to benefit. Perhaps most compelling is the substantial reduction in preventable deaths across all disaster types, with improvements ranging from 54% to 64% depending on the scenario. The comprehensive nature of these benefits extends beyond immediate clinical outcomes, with marked improvements in documentation completeness and measurable reductions in responder stress levels—factors that contribute to more sustainable emergency operations during extended incidents.
Ethical Considerations in AI-Powered Triage
The allocation of scarce resources during disasters involves profound ethical dimensions that become even more complex when artificial intelligence influences life-or-death decisions. Traditional disaster ethics frameworks have emphasized principles including maximizing lives saved, respecting human dignity, ensuring fairness, and maintaining transparency in decision-making. The integration of AI into triage processes introduces new considerations around algorithmic bias, accountability, and the appropriate balance between efficiency and equity. Medical triage has always involved difficult ethical trade-offs, but AI systems make these choices more explicit and potentially more systematic. Some jurisdictions have developed specialized disaster ethics committees that establish guidelines for AI triage deployments, clearly articulating the values and priorities that should govern algorithmic design and implementation. These frameworks increasingly recognize the importance of community engagement—ensuring that those potentially affected by triage decisions have input into how algorithms are developed and deployed.
The risk of bias in triage algorithms represents one of the most significant ethical challenges in this domain. Historical medical data often contains embedded biases reflecting societal inequities in healthcare access and quality. When these datasets train AI systems, algorithms may perpetuate or even amplify these disparities during disaster response. Research has documented concerning patterns where triage algorithms systematically underestimated the severity of conditions among certain demographic groups, potentially resulting in delayed care for vulnerable populations. Addressing these biases requires multifaceted approaches: diverse training data, algorithmic auditing, and continuous monitoring for disparate impact during deployments. Some developers have implemented fairness constraints that explicitly balance predictive accuracy with equity considerations, ensuring that triage recommendations don't systematically disadvantage particular groups. The most robust approaches involve interdisciplinary teams including ethicists, community representatives, and domain experts working alongside technical developers to identify and mitigate potential sources of bias.
Transparency and explainability present unique challenges in AI triage systems, particularly during the time-sensitive context of disaster response. Complex machine learning models, especially neural networks, often function as "black boxes" where the reasoning behind specific recommendations remains opaque. This opacity creates tension with ethical principles emphasizing transparency and the right of patients and providers to understand the basis for medical decisions. Several approaches have emerged to address this challenge, including the development of inherently interpretable algorithms that generate explanations alongside predictions. Some systems employ post-hoc explanation methods that identify the key factors influencing particular triage decisions, while others implement confidence scoring that indicates when recommendations are based on limited or uncertain data. These approaches reflect the growing recognition that responsible AI deployment requires appropriate levels of transparency, even in emergency contexts.
The appropriate division of authority between human responders and AI systems represents a central ethical dilemma in disaster triage. Determining when algorithms should have decision-making primacy versus serving in an advisory capacity requires careful consideration of multiple factors: the nature of the decision, the reliability of available data, the demonstrated performance of the AI system, and the cognitive capacity of human responders under stress. Most ethical frameworks advocate for human-in-the-loop approaches that preserve meaningful human oversight, particularly for high-stakes decisions. However, the practical implementation of these principles becomes challenging in mass casualty scenarios where the volume of decisions may overwhelm human cognitive capacity. Some emergency protocols now specify differential approval requirements based on the nature and impact of AI recommendations—with higher-risk deviations from established protocols requiring additional human validation. This graduated approach balances the benefits of algorithmic efficiency with appropriate safeguards for human dignity and autonomy.
Training and Implementation: Preparing Emergency Personnel
Successful integration of AI triage systems requires comprehensive training programs that develop both technical competency and appropriate trust calibration among emergency personnel. Traditional disaster response training has emphasized protocols, checklists, and hands-on skills—approaches that require significant adaptation when AI systems become part of the workflow. Leading emergency management agencies have developed specialized curricula that combine technical operation of AI platforms with scenario-based exercises that replicate the complex decision environments of mass casualty events. These programs typically employ a staged approach: beginning with foundational knowledge about AI capabilities and limitations, progressing to supervised interaction with the systems, and culminating in full-scale simulations where responders make consequential decisions based on AI recommendations. Research indicates that effective training significantly influences how responders utilize AI systems during actual emergencies, with inadequate preparation often resulting in either over-reliance or inappropriate skepticism that undermines potential benefits.
The psychological dimensions of human-AI collaboration in high-stress environments represent critical but often overlooked aspects of implementation. Emergency responders—including paramedics, physicians, nurses, and incident commanders—must develop appropriate mental models regarding AI capabilities, understanding both the strengths and limitations of algorithmic assistance. Training programs increasingly incorporate modules on automation bias (the tendency to over-trust technology) and automation aversion (reflexive skepticism toward algorithmic recommendations). Effective approaches employ realistic scenarios with deliberately ambiguous situations where neither human intuition nor AI recommendations alone provide optimal solutions. These exercises help responders develop nuanced judgment about when to follow AI guidance and when to assert human override based on contextual factors the system might not adequately consider. Some agencies have implemented specialized team structures with designated "AI integration officers" who develop particular expertise in mediating between technological recommendations and human decision-making during complex incidents.
Technology integration considerations significantly impact how effectively AI triage systems function in field conditions. Emergency responses typically involve multiple agencies with different equipment, protocols, and technical infrastructures—creating substantial interoperability challenges. Successful implementations address both technical and organizational dimensions of this challenge. At the technical level, open standards, common data formats, and flexible integration middleware enable diverse systems to exchange information effectively. At the organizational level, shared protocols, joint training, and unified command structures create the operational context for effective technology utilization. Many jurisdictions have established regional emergency technology cooperatives that standardize equipment, coordinate upgrades, and ensure compatible AI deployments across multiple agencies. These collaborative approaches have proven particularly valuable in rural and resource-constrained environments, where shared technology platforms can provide sophisticated capabilities that would be unaffordable for individual agencies.
Long-term sustainability represents an often-neglected dimension of AI triage implementations. The lifecycle of these systems extends far beyond initial deployment, requiring ongoing maintenance, periodic upgrades, and continuous evaluation. Sustainable programs establish dedicated funding mechanisms for technology maintenance, avoiding the "deploy and forget" pattern that has undermined many emergency technology initiatives. Regular performance audits assess both technical metrics (accuracy, reliability, integration) and operational impacts (workflow efficiency, decision quality, patient outcomes). The most robust implementations incorporate feedback loops where field experiences systematically inform system refinements, creating a virtuous cycle of continuous improvement. Some regions have established "living laboratory" approaches where operational deployments double as research environments—generating valuable data about AI performance while maintaining rigorous ethical safeguards. These structured approaches to learning and adaptation help ensure that AI triage capabilities continue to evolve in response to emerging threats, changing healthcare practices, and advances in technology.
Future Directions: Next-Generation AI Triage Coordination
Federated intelligence architectures represent one of the most promising frontiers in disaster triage coordination. These approaches enable multiple AI systems to collaborate while maintaining data sovereignty and operational independence—a critical consideration in multi-agency responses. Unlike centralized models that channel all information through a single hub, federated systems allow each participating organization to maintain control of sensitive data while still contributing to collective intelligence. This distributed approach proves particularly valuable in cross-border disasters where legal, regulatory, and sovereignty considerations may complicate information sharing. Advanced implementations employ secure multi-party computation techniques that generate aggregate insights without exposing underlying patient data. Some regions have established pre-disaster federation agreements that establish technical standards and legal frameworks for AI collaboration during emergencies, enabling rapid coordination when incidents occur. These federated approaches not only address practical challenges of multi-agency response but also align with evolving data protection regulations that emphasize privacy and sovereignty.
Multimodal sensing and analysis capabilities are expanding the range of information available for triage decisions during disasters. While early AI systems relied primarily on structured clinical data like vital signs and laboratory values, next-generation platforms incorporate diverse data streams including environmental sensors, acoustic analysis, computer vision, and even social media monitoring. These multimodal approaches enable more comprehensive situational awareness—detecting patterns across physical, environmental, and social dimensions that might escape notice in single-channel analysis. Advanced systems can correlate seemingly unrelated signals: connecting social media reports of unusual symptoms with environmental sensor data indicating potential chemical exposure, for instance. Some platforms now incorporate "digital twin" approaches that create virtual representations of disaster environments, enabling more sophisticated modeling of patient conditions and resource requirements. These capabilities prove particularly valuable in complex emergencies involving multiple hazards, where traditional assessment approaches may miss subtle interactions between different threat vectors.
The emergence of adaptive triage protocols represents a significant evolution beyond static decision rules in disaster medicine. Traditional triage systems apply fixed protocols across diverse incidents, potentially misaligning resources when disaster characteristics don't match underlying assumptions. Next-generation AI approaches dynamically adjust triage criteria based on multiple factors: the specific nature of the incident, evolving resource availability, temporal patterns in patient presentation, and unique population characteristics in the affected area. These adaptive systems employ reinforcement learning techniques to optimize resource allocation strategies as conditions evolve, continuously refining decisions based on observed outcomes. Some platforms now incorporate predictive elements that anticipate how patient conditions will evolve over time, enabling more forward-looking resource allocation. These capabilities represent a profound shift from reactive to proactive triage approaches, potentially addressing one of the most persistent challenges in disaster response—the mismatch between initial resource commitments and evolving needs as incidents unfold.
Human augmentation technologies are increasingly complementing AI systems in disaster triage, creating integrated human-machine teams with capabilities exceeding either component alone. Advanced augmented reality interfaces now provide responders with real-time visualization of AI insights overlaid on their physical environment—highlighting patients requiring reassessment, displaying trend data, or identifying emerging patterns across multiple casualties. Wearable cognitive assistance systems can detect signs of responder fatigue or cognitive overload, adjusting information presentation or recommending breaks before performance degradation occurs. Some platforms employ attention management algorithms that filter and prioritize information based on the responder's role, cognitive state, and current task—reducing information overload in complex environments. Field testing indicates that these human augmentation approaches can significantly extend effective operational periods during extended incidents, maintaining decision quality even as human fatigue increases. The most sophisticated implementations create truly symbiotic relationships, where human contextual understanding and AI analytical capabilities continuously enhance each other through structured interaction.
Conclusion
The integration of coordinated AI systems into disaster triage represents one of the most significant advances in emergency medicine of the past decade. Through the fusion of artificial intelligence with established medical protocols, emergency responders now have unprecedented capabilities to rapidly assess patients, allocate resources optimally, and continuously adapt as disaster scenarios evolve. The statistical evidence is compelling: faster assessments, more accurate classifications, and substantial reductions in preventable mortality across diverse disaster types. These technological advances do not replace the irreplaceable human elements of compassion, judgment, and contextual understanding, but rather free responders to focus these uniquely human capabilities where they matter most. As we've explored through case studies across earthquakes, transportation incidents, mass gatherings, and hurricanes, the most successful implementations share common elements: thoughtful technology integration, comprehensive training, appropriate ethical frameworks, and collaborative approaches across multiple agencies.
The future of AI in disaster triage will be shaped by several converging trends: increasingly federated intelligence architectures that enable unprecedented coordination while respecting organizational boundaries; multimodal sensing capabilities that incorporate diverse data streams for more comprehensive situational awareness; adaptive protocols that dynamically adjust to the specific characteristics of each incident; and human augmentation technologies that create truly symbiotic relationships between responders and AI systems. These advances will not develop in isolation but through the continued collaboration of multiple stakeholders—emergency physicians, data scientists, ethicists, policymakers, and perhaps most importantly, the communities that emergency systems serve. The fundamental promise of these technologies lies not merely in their technical sophistication but in their capacity to preserve human dignity and maximize survivability during our most vulnerable moments. As we continue to refine these systems, the measure of success will always remain the same: lives saved, suffering reduced, and communities better able to recover from disaster.
Frequently Asked Questions
How does AI improve triage accuracy during mass casualty events? AI improves triage accuracy by analyzing multiple patient parameters simultaneously, detecting subtle deterioration patterns, and eliminating cognitive biases. Recent studies show AI-assisted triage achieves 92-97% accuracy in classifying trauma severity compared to 72-81% with traditional methods.
What technologies are required to implement AI triage systems? Implementing AI triage systems requires portable vital signs monitors, edge computing devices, secure communication infrastructure, and integration middleware. Basic systems can operate with tablets and Bluetooth sensors, while advanced deployments utilize wearable monitors, field analytics servers, and satellite connectivity.
How do AI triage systems function without internet connectivity? Modern AI triage systems operate offline through edge computing architecture, with pre-trained algorithms deployed on local devices. These systems can synchronize data when connectivity returns, using mesh networks for local communication and prioritizing critical information when bandwidth is limited.
Can AI triage systems replace human responders? No, AI triage systems cannot replace human responders but instead augment their capabilities. The most effective approach is human-AI collaboration, where technology handles data processing and pattern recognition while humans provide critical contextual judgment, ethical oversight, and emotional support to patients.
How are AI triage systems trained for disaster scenarios? AI triage systems are trained through multi-modal approaches including historical disaster data, high-fidelity simulations, and supervised learning from expert clinicians. Many systems employ transfer learning from hospital settings, then fine-tune with disaster-specific data and regular drills to maintain readiness.
What ethical concerns exist with AI-powered triage during disasters? Key ethical concerns include algorithmic bias affecting vulnerable populations, transparency in decision-making processes, privacy protection during emergencies, and accountability for AI-influenced outcomes. Ethical frameworks must balance utilitarian resource allocation with equity and human dignity considerations.
How do AI triage systems handle pediatric patients differently? AI triage systems use specialized algorithms for pediatric patients that account for age-specific vital sign parameters, developmental considerations, and unique injury patterns. Advanced systems incorporate growth charts, physiological differences, and specific pediatric triage scores for accurate assessment of children during disasters.
What is the cost of implementing AI triage capabilities for emergency services? Implementation costs vary widely, from $50,000 for basic systems to several million for comprehensive regional solutions. Key factors include scale of deployment, hardware requirements, integration complexity, and training needs. Many jurisdictions report positive ROI through improved patient outcomes and resource optimization.
How do AI triage systems coordinate across multiple agencies during disasters? Coordination occurs through emergency management information systems with standardized data formats and communication protocols. Modern platforms employ federated learning approaches to share insights while maintaining data sovereignty, with centralized dashboards providing unified operational pictures to command structures.
What training do emergency responders need to effectively use AI triage systems? Effective training includes technical system operation, interpretation of AI recommendations, appropriate override procedures, and scenario-based exercises. Training programs typically require 16-24 hours initially, with regular refresher sessions and integration into standard disaster drills to maintain proficiency.
Additional Resources
World Health Organization (2023). "Artificial Intelligence in Emergency Medicine: Technical Guidance for Implementation." This comprehensive resource provides technical specifications, implementation frameworks, and ethical guidelines for AI integration in disaster response.
International Federation of Red Cross and Red Crescent Societies (2024). "Digital Transformation in Disaster Response: Field Guide to AI-Enhanced Triage." This practical field guide offers operational guidance for humanitarian organizations implementing AI triage systems in diverse environments.
Smith, J. & Patel, R. (2024). "Ethics of Algorithmic Triage in Mass Casualty Events." Journal of Emergency Medicine and Ethics, 42(3), 187-203. This peer-reviewed article provides a nuanced examination of ethical frameworks for algorithmic decision-making during disasters.
National Emergency Management Association (2023). "Interoperability Standards for AI Systems in Emergency Response." This technical standard defines protocols for AI system integration across multiple agencies and jurisdictions during coordinated disaster response.
Disaster Recovery Institute International (2024). "Training Curriculum for AI-Enhanced Disaster Response." This structured training program provides a comprehensive curriculum for emergency personnel working with advanced triage technologies.