AI Triage and Predictive Analytics for Optimized ED Flow
Discover how AI triage systems and predictive analytics are transforming emergency departments, reducing wait times by up to 30%, and improving patient outcomes through data-driven healthcare management.
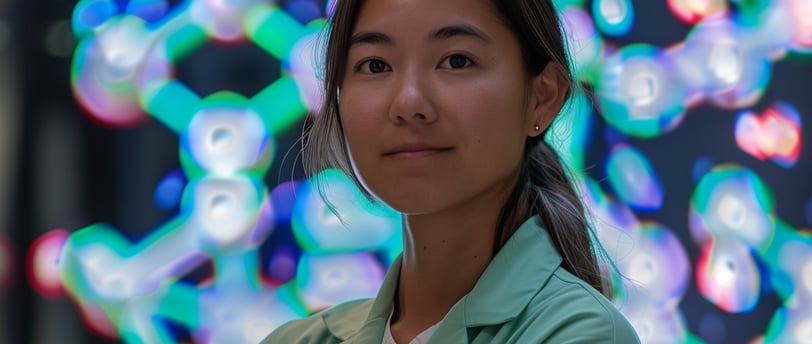
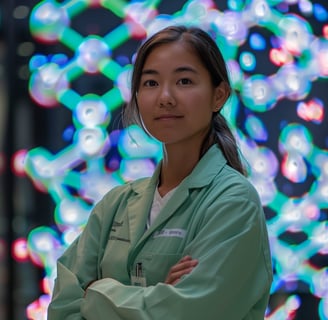
Emergency departments (EDs) worldwide are under increasing pressure, with overcrowding reaching crisis levels in many hospitals. According to a recent Agency for Healthcare Research and Quality study, the average ED wait time in the United States exceeds 145 minutes, with some facilities reporting wait times of over 4 hours during peak periods. These delays not only frustrate patients but can lead to serious health consequences, including increased mortality rates for critical cases that aren't identified promptly. Applying artificial intelligence (AI) and predictive analytics to emergency department triage and flow management represents a revolutionary approach to addressing these challenges. Healthcare facilities can dramatically improve patient classification, resource allocation, and treatment prioritisation by leveraging advanced algorithms and real-time data analysis. This article explores how AI triage systems and predictive analytics transform emergency care, examining implementation strategies, success stories, and the future potential of these technologies to create more efficient, effective emergency departments.
The Crisis in Emergency Department Flow Management
Emergency departments serve as the frontline of healthcare for millions of patients each year, providing critical services for everything from minor injuries to life-threatening conditions. Unfortunately, the increasing demand for emergency services, staffing shortages, and resource constraints have created inefficiency in many facilities. A report by the Institute of Medicine identified ED overcrowding as one of the most significant challenges facing healthcare systems today, with far-reaching implications for patient safety and care quality. Traditional triage approaches rely heavily on manual assessments by nursing staff, often using standardised tools like the Emergency Severity Index (ESI) or Manchester Triage System (MTS). While these systems have proven valuable, they are inherently limited by human factors, including variability between providers, cognitive biases, and the inability to process vast amounts of information simultaneously. The consequences of suboptimal triage can be severe, leading to the undertriage of truly urgent cases or the over-triage of less critical patients, contributing to inefficient resource utilisation. Moreover, the reactive nature of conventional ED management means that facilities often struggle to anticipate surges in demand, resulting in staffing misalignments and treatment delays.
With these challenges in mind, healthcare innovators have begun exploring how artificial intelligence might transform emergency department operations.
Understanding AI Triage Systems
Artificial intelligence triage systems represent a significant advancement over traditional approaches, leveraging sophisticated algorithms to analyse patient data and accurately predict acuity levels. Unlike conventional triage protocols that typically consider a limited set of variables, AI systems can process hundreds of data points simultaneously, identifying subtle patterns that might escape even experienced clinicians. These systems typically integrate with existing electronic health records (EHRs), extracting relevant information such as vital signs, chief complaints, medical history, and demographic factors to generate risk scores. Advanced AI triage platforms employ machine learning techniques, including deep neural networks, random forests, and gradient boosting, to continuously refine their predictions through feedback loops that incorporate actual patient outcomes. Some cutting-edge systems even incorporate natural language processing (NLP) to analyse unstructured data from clinical notes, extracting valuable insights that might remain buried in the text.
The technical sophistication of these systems translates into tangible clinical benefits, with studies showing that AI-enhanced triage can improve accuracy by 15-30% compared to traditional methods. Importantly, AI triage does not aim to replace human clinicians but to augment their capabilities, providing decision support that helps identify high-risk patients who might otherwise be missed. This collaborative approach combines the computational power of AI with the irreplaceable clinical judgment and empathy of healthcare professionals. Many leading healthcare systems have already begun implementing AI triage, with notable examples including the University of California San Francisco's deployment of a machine learning algorithm that reduced undertriage rates by 27% within six months of implementation.
While AI triage focuses primarily on patient classification at the point of entry, predictive analytics extends this approach to optimise the entire emergency department workflow.
Predictive Analytics: Forecasting ED Demand and Resource Needs
Predictive analytics represents the next frontier in emergency department management, enabling facilities to transition from reactive to proactive operations through data-driven forecasting. Unlike traditional retrospective analyses that merely document past trends, predictive models leverage historical data to anticipate future scenarios with remarkable accuracy. These systems analyse patterns in ED utilisation across various timeframes—hourly, daily, weekly, and seasonal—identifying correlations with factors such as weather conditions, local events, disease outbreaks, and even social determinants of health. The resulting forecasts allow administrators to optimise staffing levels, anticipating periods of high demand and adjusting resource allocation accordingly. Advanced systems even incorporate real-time data feeds from regional healthcare networks, ambulance services, and urgent care facilities to provide continuously updated predictions.
Predictive analytics applications in emergency settings extend well beyond simple volume forecasting. Sophisticated models can predict patient flow throughout the ED journey, estimate the length of stay, identify potential bottlenecks, and suggest optimal patient pathways. Some systems leverage machine learning to predict specific clinical outcomes, such as the likelihood of admission, ICU transfer, or return visits within 72 hours. These insights enable proactive interventions, such as early initiation of inpatient bed requests for patients with high admission probability or enhanced discharge planning for those at risk of "bounce backs." The financial implications are equally significant, with studies suggesting that effective implementation of predictive analytics can reduce operational costs by 10-15% while simultaneously improving quality metrics.
While the potential benefits of AI triage and predictive analytics are compelling, successful implementation requires careful planning and a structured approach.
Implementation Strategies for AI-Enhanced ED Flow
Implementing AI solutions in emergency settings requires thoughtful planning and a phased approach that addresses both technical and human factors. The foundation of any successful implementation begins with data quality assessment and infrastructure preparation, ensuring that the facility has robust systems for collecting, storing, and processing the information needed to train and operate AI models. Healthcare leaders should conduct a thorough needs assessment, identifying specific pain points in their current workflow that could benefit from AI enhancement. Rather than attempting a complete overhaul, most successful implementations begin with targeted pilot projects focused on well-defined problems, such as improving triage accuracy for specific patient populations or predicting daily staffing requirements. This incremental approach allows for careful evaluation of results and refinement of systems before broader deployment.
Effective change management represents the most critical success factor in AI implementation. Healthcare professionals may initially view these technologies with scepticism or concern about their roles being diminished or replaced. Addressing these concerns requires transparent communication about how AI will augment rather than replace clinical judgment and comprehensive training programs that build comfort and competence with new systems. The implementation team should include representatives from all stakeholder groups—physicians, nurses, administrative staff, and IT specialists—ensuring that diverse perspectives inform the process. Regular feedback sessions during the early implementation phase allow for quick identification and resolution of issues, building confidence in the system. Legal and regulatory compliance must also be carefully considered, particularly to patient privacy, data security, and regulatory requirements for clinical decision support tools.
The theoretical benefits of AI-enhanced emergency departments are compelling, but real-world examples provide the most convincing evidence of their potential impact.
Case Studies: Success Stories in AI-Enhanced Emergency Care
Several pioneering healthcare organisations have already demonstrated the transformative potential of AI and predictive analytics in emergency settings. Johns Hopkins Hospital implemented an AI-powered capacity command centre that integrates predictive analytics with real-time data visualisation, enabling proactive patient flow management throughout the facility. Within 18 months of implementation, the system reduced ED boarding times by 60% and ambulance diversions by 25%, significantly improving operational efficiency and patient experience. The ED team credits the system's ability to predict admission needs 24 hours in advance as a key factor in these improvements, allowing for proactive bed management and smoother departmental transitions.
In another compelling example, Kaiser Permanente deployed a machine learning algorithm to enhance their triage process, analysing over 200 variables to identify patients at high risk for critical outcomes. The system demonstrated particular value in detecting subtle presentations of severe conditions such as sepsis and acute coronary syndrome, which sometimes present with atypical symptoms that might be missed in traditional triage. After implementation, Kaiser reported a 12% reduction in adverse events and a 20% improvement in appropriate resource allocation. Smaller facilities have also achieved impressive results; Central Maine Medical Center implemented a predictive analytics system that reduced their average door-to-provider time by 31 minutes through optimised staffing and improved patient flow management. Their approach focused on balancing workloads across treatment areas, using AI to direct patients to appropriate care settings based on acuity and predicted resource needs.
Despite these promising results, implementing AI in emergency settings raises important ethical considerations that must be thoughtfully addressed.
Ethical Considerations and Challenges
Integrating AI and predictive analytics into emergency care raises significant ethical questions that healthcare leaders must carefully navigate. Algorithm bias represents one of the most pressing concerns, as models trained on historical data may perpetuate or amplify existing healthcare delivery disparities. Research has shown that some AI systems demonstrate varying levels of accuracy across different demographic groups, potentially leading to systematic undertriage of specific populations. Addressing this issue requires diverse training data, regular auditing of algorithm performance across population subgroups, and continuous refinement to ensure equitable outcomes. Privacy considerations loom, particularly as systems incorporate increasingly granular patient data to improve predictive accuracy. Healthcare organisations must implement robust safeguards to protect sensitive information while leveraging its analytical value.
The question of transparency and explainability presents another challenge, as many advanced AI models operate as "black boxes" whose decision-making processes cannot be easily interpreted by human users. This lack of transparency can undermine clinician trust and raises questions about accountability when algorithm recommendations influence patient care decisions. Regulatory frameworks for AI in healthcare are still evolving, creating uncertainty about compliance requirements and potential liability issues. Despite these challenges, most experts agree that the ethical implementation of AI in emergency settings is both possible and necessary, requiring thoughtful governance structures, ongoing evaluation, and a commitment to using these powerful tools to reduce rather than reinforce healthcare disparities.
Conclusion
Integrating AI triage systems and predictive analytics represents a transformative approach to emergency department management, offering solutions to longstanding challenges in patient flow, resource allocation, and clinical decision-making. As demonstrated by early adopters like Johns Hopkins, Kaiser Permanente, and numerous other healthcare systems, these technologies can deliver impressive improvements across multiple dimensions—reducing wait times, enhancing triage accuracy, optimising resource utilisation, and ultimately improving patient outcomes. The journey toward AI-enhanced emergency care is not without challenges, requiring careful attention to implementation strategies, change management, and ethical considerations. However, the potential benefits far outweigh these obstacles, particularly as AI technologies continue to evolve and healthcare organisations become increasingly sophisticated in their application.
Looking ahead, we can anticipate further innovation in this space, with increasingly personalised risk models that account for social determinants of health, integration with remote monitoring technologies, and expanded applications throughout the patient journey. For healthcare leaders contemplating this transformation, the evidence suggests that the question is no longer whether to implement AI and predictive analytics but rather how to do so most effectively. Those who embrace these technologies thoughtfully and strategically will be well-positioned to deliver emergency care that is not only more efficient but ultimately more equitable and patient-centred. The emergency department of the future—data-driven, proactive, and precisely calibrated to patient needs—is no longer a distant vision but an emerging reality that promises to benefit patients, providers, and healthcare systems alike.
FAQ Section
Here are ten frequently asked questions about AI triage and predictive analytics in emergency departments:
What is AI triage in emergency departments? AI triage in emergency departments refers to using artificial intelligence algorithms to analyse patient data and determine the urgency and appropriate level of care needed. These systems typically use machine learning to process multiple variables, including vital signs, symptoms, medical history, and demographic information, to predict patient acuity more accurately than traditional triage methods.
How does predictive analytics improve emergency department flow? Predictive analytics improves ED flow by forecasting patient volume, resource needs, and likely bottlenecks before they occur. These systems analyse historical data patterns to anticipate hourly, daily, and seasonal demand fluctuations, allowing hospitals to optimise staffing, bed management, and resource allocation proactively rather than reactively.
What percentage improvement in wait times can hospitals expect from implementing AI triage? Hospitals implementing AI triage systems typically report average wait time reductions of 20-30%, with some institutions achieving improvements of up to 40-60% during peak periods. The exact improvement depends on factors including baseline efficiency, implementation approach, and how comprehensively the AI system is integrated with existing workflows.
Does AI triage replace human clinicians in the emergency department? AI triage does not replace human clinicians but augments their capabilities. These systems serve as decision-support tools that help identify high-risk patients and optimize resource allocation while healthcare professionals maintain final clinical judgment and provide the human elements of care, including empathy and complex decision-making.
What data is required to implement predictive analytics in an emergency department? Implementing predictive analytics typically requires historical ED visit data (at least 1-2 years), including arrival times, patient demographics, acuity levels, chief complaints, length of stay, disposition, and resource utilisation. Additional valuable data includes staffing patterns, local event calendars, weather data, and regional healthcare utilisation trends.
How long does it typically take to implement AI triage and predictive analytics systems? Full implementation typically takes 9-24 months, with the timeline varying based on organisational readiness, data availability, and implementation scope. Most successful deployments follow a phased approach, with limited pilots demonstrating value before expanding to comprehensive integration across all emergency department functions.
What are the main ethical concerns related to AI triage in emergency care? The main ethical concerns include algorithmic bias that may disadvantage certain demographic groups, privacy considerations related to sensitive patient data, questions about transparency and explainability of AI decision-making, and responsibility/liability issues when algorithms influence clinical decisions. Ongoing monitoring and governance are essential to address these concerns.
How do AI triage systems handle patients with complex or unusual presentations? Advanced AI triage systems are designed to flag unusual or complex presentations that don't fit standard patterns, ensuring these cases receive appropriate clinical attention. Many systems incorporate confidence scores with their predictions and are programmed to recommend higher levels of care when uncertainty exists, minimising the risk of undertriage for atypical cases.
What ROI can hospitals expect from implementing AI-enhanced ED flow optimisation? Hospitals typically report ROI ranging from 200-400% within 2-3 years of implementation, with financial benefits stemming from reduced labour costs through optimised staffing, decreased left-without-being-seen rates, improved billing capture, and reduced liability exposure. Additional value comes from improved patient satisfaction scores and quality metrics.
How does AI triage impact emergency department staffing requirements? AI triage doesn't necessarily reduce overall staffing requirements but helps optimise when and where staff are deployed. Predictive systems allow for more precise staffing alignment with anticipated demand patterns, potentially reducing overtime costs while improving care quality through more appropriate distribution of clinical resources when and where they're most needed.
Additional Resources
For readers interested in exploring AI triage and predictive analytics in emergency departments further, the following resources provide valuable insights:
The AI-Powered Hospital: How Artificial Intelligence is Transforming Medicine by Dr. Eric Topol (2023) - A comprehensive examination of AI applications in healthcare, with detailed chapters on emergency medicine applications and implementation strategies.
Journal of the American Medical Informatics Association Special Issue on AI in Emergency Care (2024) - A collection of peer-reviewed research articles detailing the latest advances in AI applications for emergency medicine, including multicenter studies on implementation outcomes.
Emergency Department Optimization Toolkit by the Institute for Healthcare Improvement—This is a practical guide for healthcare leaders implementing process improvements in emergency settings. Recent additions cover AI integration and change management strategies.
"Implementing AI in Emergency Medicine: Ethical Framework and Best Practices" - A consensus statement from the American College of Emergency Physicians guiding responsible AI implementation with particular attention to bias mitigation and governance structures.
HealthTech Magazine's "Predictive Analytics in Healthcare" Resource Hub - A regularly updated collection of case studies, implementation guides, and technology reviews focused on predictive analytics applications in various healthcare settings, including emergency departments.