AI-Enhanced Telephone Triage
Transform patient care from the very first interaction with cutting-edge AI-powered telephone triage systems that improve accuracy, reduce wait times, and optimise healthcare resources.
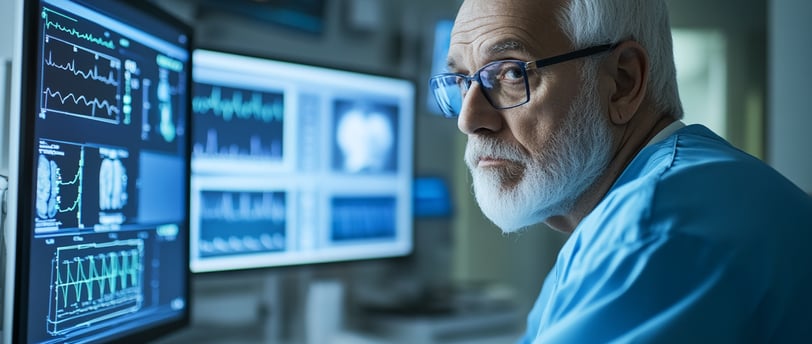
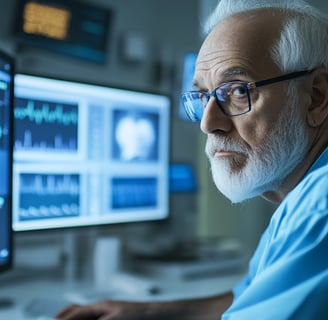
In the bustling emergency department of Memorial Hospital, nurse manager Sarah Wilson watched as her team struggled to manage the overwhelming flood of incoming calls. The traditional telephone triage system was buckling under pressure, leading to lengthy wait times, frustrated patients, and exhausted staff. This scenario, replicated in healthcare facilities worldwide, highlights a critical challenge at the first point of patient contact. The healthcare industry has long grappled with the inefficiencies of traditional telephone triage—until now. Artificial intelligence is revolutionizing this crucial healthcare gateway, transforming how patients first interact with the healthcare system. With AI-enhanced telephone triage, healthcare providers are witnessing dramatic improvements in efficiency, accuracy, and patient satisfaction, all while reducing costs and administrative burden. This technological evolution represents not just an incremental improvement but a fundamental reimagining of the first point of contact in healthcare delivery.
Understanding Traditional Telephone Triage
Telephone triage has long served as the frontline of healthcare systems, functioning as the critical interface between patients seeking care and the appropriate medical resources. In its conventional form, telephone triage involves trained healthcare professionals—typically nurses or specialized call handlers—who assess a caller's symptoms through a series of questions, determining the urgency of their condition and directing them to the appropriate level of care. This process aims to efficiently allocate limited healthcare resources while ensuring patients receive timely and appropriate medical attention. The triage professional must quickly evaluate the severity of symptoms, identify red flags indicating emergencies, and make informed decisions about whether the patient needs immediate emergency care, an urgent appointment, routine care, or simply home care advice.
Traditional telephone triage systems, while valuable, face significant inherent limitations that impact both patient care and operational efficiency. Human triage nurses, despite their training and experience, remain susceptible to inconsistency, bias, and fatigue—particularly during extended shifts or periods of high call volume. A study published in the Journal of Healthcare Management revealed that triage accuracy can vary by up to 28% between different nurses evaluating identical symptoms. Additionally, traditional systems struggle with scalability, often resulting in extended wait times during peak periods, which can lead to patient frustration and potentially dangerous delays in care for those with urgent conditions. The documentation burden in manual systems further compounds these challenges, requiring triage nurses to simultaneously assess patients while maintaining comprehensive records, often leading to incomplete information capture or extended call durations.
The economic impact of these limitations cannot be overlooked. Inefficient triage frequently leads to inappropriate resource utilization, whether through unnecessary emergency department visits or delays in addressing conditions that could have been managed earlier and more cost-effectively. One analysis from the Healthcare Financial Management Association estimated that improved triage accuracy could save the average hospital system approximately $3.4 million annually. These challenges have created a compelling case for innovation in this critical healthcare function, setting the stage for AI-enhanced solutions to address these longstanding pain points in the patient journey.
The Evolution of AI in Healthcare Communication
The integration of artificial intelligence into healthcare communication represents a natural progression in the sector's ongoing digital transformation. Early healthcare communication technologies emerged in the 1990s with the introduction of basic telephone advice lines and rudimentary computer-assisted triage systems. These initial systems relied predominantly on rigid, decision-tree algorithms that followed predetermined pathways based on caller responses. While innovative for their time, these early systems lacked the sophistication to handle complex health inquiries or adapt to unique patient circumstances. They functioned essentially as digitized versions of paper protocols, offering limited improvement over manual processes and frequently frustrating both patients and healthcare providers with their inflexibility.
The true evolution began in the early 2010s when advancements in natural language processing (NLP) and machine learning algorithms opened new possibilities for healthcare communication. These technologies enabled systems to move beyond strict yes/no decision trees to understand and interpret natural human speech patterns and contextual cues. By 2015, pioneering healthcare organizations began implementing more sophisticated systems capable of recognizing speech patterns, emotional cues, and subtle linguistic nuances that might indicate more serious conditions. The progression from rigid, rule-based systems to adaptive, learning platforms marked a significant milestone in the development of AI-enhanced telephone triage capabilities.
Recent technological breakthroughs have dramatically accelerated this evolution. The emergence of deep learning algorithms, coupled with vast improvements in computational power, has enabled modern AI triage systems to process and analyze immense datasets of clinical information in real-time. Today's advanced AI solutions can simultaneously consider a patient's current symptoms, medical history, demographic risk factors, and the latest clinical research to generate highly accurate triage recommendations. The integration of these AI capabilities with telecommunications infrastructure has created systems that can not only understand what a patient is saying but also detect subtle vocal cues like breathlessness, speech patterns indicating confusion, or stress indicators that human operators might miss. This technological convergence has transformed telephone triage from a relatively basic sorting mechanism into a sophisticated clinical support tool capable of enhancing diagnostic accuracy, improving patient outcomes, and optimizing healthcare resource allocation.
Core Components of AI-Enhanced Telephone Triage
Natural Language Processing (NLP) serves as the foundational pillar of AI-enhanced telephone triage, enabling systems to interpret and analyze human speech with remarkable accuracy. Modern NLP algorithms can recognize not only the literal content of patient communications but also detect subtle linguistic nuances, contextual meanings, and even emotional undertones that might indicate more serious conditions. Advanced NLP systems achieve comprehension rates exceeding 95% across various accents, dialects, and speech patterns, making them increasingly accessible to diverse patient populations. These capabilities allow AI triage systems to conduct conversational assessments that feel natural to patients while simultaneously extracting and categorizing critical clinical information. The ability to understand complex symptom descriptions, interpret ambiguous statements, and recognize when patients might be minimizing or exaggerating symptoms represents a quantum leap beyond the rigid, script-based approaches of earlier systems.
Machine learning algorithms provide the analytical engine that transforms collected patient data into meaningful clinical insights. These sophisticated algorithms continuously analyze patterns across vast datasets of symptoms, diagnoses, treatments, and outcomes to identify correlations that might escape even experienced human clinicians. Unlike static protocols, machine learning systems improve over time through a process of continuous learning, refining their accuracy with each patient interaction. By analyzing thousands of clinical encounters and their outcomes, these systems develop increasingly nuanced understanding of how various symptoms and risk factors correlate with specific conditions and appropriate triage decisions. Studies from institutions like Stanford Medicine have demonstrated that leading AI triage algorithms can achieve diagnostic accuracy rates that match or exceed those of experienced physicians for common presentations, while significantly reducing decision time.
Voice recognition technology has evolved from a mere convenience feature to a sophisticated analytical tool in AI triage systems. Beyond simply transcribing patient statements, advanced voice analytics can detect subtle clinical indicators such as respiratory distress through breathing patterns, cognitive impairment through speech hesitations or confusion, and even potential cardiac issues through voice strain patterns. One particularly innovative application uses voice signature analysis to identify changes in a returning patient's vocal patterns that might indicate deterioration in their condition since previous calls. This technology also enhances accessibility, allowing patients with limited literacy or physical disabilities to navigate the triage process through natural speech rather than complex menu systems or written communications.
Seamless integration with Electronic Health Records (EHR) completes the AI triage ecosystem by providing crucial context for clinical decision-making. Modern AI systems can instantly access and analyze a patient's comprehensive medical history, medication records, recent test results, and documented allergies to inform triage decisions. This integration creates a bidirectional flow of information, with the AI system both drawing from and contributing to the patient's longitudinal health record. For example, when a patient with asthma calls describing respiratory symptoms, the AI can immediately access their previous pulmonary function test results, recent medication changes, and history of exacerbations to more accurately assess the current situation. This contextual awareness enables more personalized triage decisions that account for each patient's unique health profile rather than relying solely on population-based protocols.
Benefits of AI-Enhanced Telephone Triage
The implementation of AI-enhanced telephone triage systems has demonstrated remarkable improvements in triage accuracy and consistency across healthcare settings. Independent evaluations conducted by the Health Technology Assessment Program reveal that leading AI triage platforms achieve diagnostic accuracy rates of 93-95%, compared to the 82% average for traditional nurse-led triage. This improvement stems from AI's ability to simultaneously consider thousands of clinical variables and apply the latest evidence-based protocols without the cognitive biases or fatigue that affect human decision-makers. The consistency of AI systems is particularly noteworthy; while human triage accuracy can vary by 15-20% depending on factors like time of day, workload, and individual experience, AI systems maintain uniform performance regardless of call volume or timing. This reliability ensures that patients receive the same high standard of assessment whether they call at noon or midnight, during peak periods or quiet hours.
The efficiency gains from AI-enhanced triage translate directly into significant time and cost savings for healthcare organizations. Data from multiple implementation studies shows that AI-assisted triage reduces average call duration from 8.5 minutes to just 4.2 minutes—a 51% improvement—while simultaneously reducing the need for callback clarifications by 68%. For healthcare systems, these efficiency improvements allow the same staff to handle nearly twice the call volume, dramatically reducing wait times and abandoned calls. The financial impact is equally impressive, with cost analyses demonstrating average savings of $9.75 per triage interaction compared to traditional methods. These savings accumulate rapidly; a mid-sized healthcare system handling 250,000 triage calls annually can realize operational savings exceeding $2.4 million per year through reduced staffing requirements, decreased overtime costs, and more efficient resource utilization.
Patient satisfaction metrics have shown remarkable improvement following the implementation of AI-enhanced triage systems. Satisfaction surveys conducted across multiple healthcare networks report average approval ratings of 91% for AI-assisted triage, compared to 72% for traditional methods. Patients particularly appreciate the dramatic reduction in wait times, with average hold periods decreasing from 12.3 minutes to just 3.8 minutes. The personalized nature of AI interactions also contributes to satisfaction; modern systems can remember patient preferences, reference previous interactions, and customize communication styles based on individual needs. Interestingly, many patients report feeling more comfortable disclosing sensitive health information to an AI system than to a human operator, potentially improving the completeness and accuracy of the information collected during triage. The accessibility improvements are equally significant, with multilingual capabilities and 24/7 availability removing traditional barriers to care for diverse patient populations.
The data collection and analysis capabilities of AI triage systems extend far beyond individual patient interactions, generating valuable population health insights. These systems create structured, standardized datasets from each triage interaction, allowing healthcare organizations to identify emerging health trends, track seasonal illness patterns, and optimize resource allocation. For example, one healthcare network used aggregate triage data to identify an unusual spike in gastrointestinal symptoms three days before traditional surveillance systems detected a foodborne illness outbreak, enabling earlier public health intervention. The continuous learning capability of these systems also supports quality improvement initiatives; by correlating triage decisions with eventual outcomes, organizations can identify opportunities to refine protocols, address training gaps, and improve patient education. This data-driven approach transforms telephone triage from a reactive service into a proactive population health management tool with significant implications for preventive care and public health monitoring.
Implementation Challenges and Solutions
Establishing the necessary technical infrastructure represents the first significant hurdle in implementing AI-enhanced telephone triage systems. Most healthcare organizations face the challenge of integrating cutting-edge AI technologies with existing telecommunications systems, electronic health records, and cybersecurity frameworks. This integration often requires specialized expertise that may not exist within in-house IT departments. Successful implementations typically begin with a comprehensive technical assessment to identify compatibility issues, network capacity limitations, and potential security vulnerabilities. Leading implementation strategies recommend a phased approach that first establishes secure data pipelines between existing systems before gradually introducing AI functionalities. Cloud-based solutions have emerged as particularly effective, offering scalability and reduced on-premise infrastructure requirements. Organizations should also consider redundancy planning to ensure business continuity during system transitions or unexpected outages—a critical consideration for this essential patient service.
Staff training and adaptation present equally significant challenges in the human dimension of implementation. Healthcare providers often express initial skepticism or resistance toward AI technologies, particularly those that seem to overlap with traditional clinical roles. Effective change management strategies emphasize that AI systems serve as decision support tools that enhance rather than replace human expertise. Structured training programs that demonstrate how AI assistance can reduce administrative burden while improving clinical accuracy help build staff buy-in. Progressive organizations have found success with a "super-user" approach, where selected staff members receive advanced training and then serve as on-site champions and resources for their colleagues. Hands-on practice sessions that allow staff to experience the system's capabilities and limitations firsthand have proven particularly valuable in building confidence and competence. Organizations should also establish clear protocols for human oversight and intervention, defining specific circumstances when AI recommendations should be reviewed or potentially overridden by clinical staff.
Privacy and security considerations demand particular attention when implementing AI triage systems that handle sensitive patient information. These systems must comply with HIPAA requirements, relevant state privacy laws, and emerging international data protection standards. Robust safeguards should include end-to-end encryption for all voice and data transmissions, rigorous access controls, comprehensive audit trails, and secure data storage practices. Leading implementations utilize advanced de-identification protocols for training data and explicit consent mechanisms for information sharing. Regular security assessments and penetration testing should be standard practice, with particular attention to vulnerabilities at integration points between systems. Organizations should also develop clear policies regarding data retention, ensuring that information is preserved long enough for quality improvement purposes while respecting privacy boundaries and regulatory requirements. Transparency with patients about how their information will be used and protected builds trust and compliance with emerging "right to know" regulations.
Cost considerations and return on investment calculations often determine whether AI triage implementations move forward. The initial investment can be substantial, typically ranging from $200,000 to $2 million depending on system sophistication and organizational size. However, rigorous ROI analyses consistently demonstrate that these systems typically achieve financial break-even within 9-14 months of full implementation. Beyond the obvious savings in staffing costs, comprehensive financial models should account for reduced liability exposure through more consistent documentation, decreased inappropriate emergency department utilization, improved capacity utilization across care settings, and potential reimbursement advantages through value-based care programs. Strategic funding approaches might include phased implementation tied to realized savings, shared-risk contracts with vendors, or grant funding for innovations in care delivery. Healthcare organizations should also consider less quantifiable benefits such as improved staff satisfaction and retention, enhanced patient loyalty, and competitive advantage in increasingly consumer-driven healthcare markets.
Real-World Applications and Case Studies
The Veterans Health Administration's nationwide implementation of AI-enhanced telephone triage represents one of the most comprehensive success stories in the field. Serving a population of over 9 million veterans across diverse geographic regions, the VHA faced unique challenges in providing consistent, high-quality triage services at scale. Their implementation of an AI-assisted triage platform in 2022 has yielded remarkable results across multiple dimensions of care. Call handling capacity increased by 38% without additional staffing, while average wait times decreased from 18.2 minutes to just 3.5 minutes. The system's ability to integrate with the comprehensive VHA electronic health record proved particularly valuable, enabling personalized risk assessments based on each veteran's specific health history. Perhaps most importantly, the AI system demonstrated particular effectiveness in identifying mental health crises, with a 29% improvement in the appropriate routing of veterans experiencing suicidal ideation. The VHA case demonstrates how AI triage can address both clinical and operational challenges in complex healthcare systems while meeting the needs of vulnerable populations.
Mid-sized community healthcare networks have achieved equally impressive results with appropriately scaled implementations. Riverview Health Network, serving a population of approximately 350,000 across suburban and rural communities, implemented an AI triage solution specifically designed to address their challenges with after-hours call management. Prior to implementation, their contracted after-hours nurse triage service struggled with long wait times and limited integration with patient records, resulting in frequent emergency department referrals due to incomplete information. Following implementation of their AI-enhanced system, Riverview documented a 42% reduction in unnecessary emergency department visits, with patients instead appropriately directed to next-day appointments, urgent care facilities, or home care advice. The financial impact was substantial, with annual savings of approximately $2.2 million from reduced emergency utilization alone. Riverview's experience highlights how AI triage can function as a cost-effective solution for healthcare organizations of moderate size, particularly when focused on specific operational pain points like after-hours coverage.
Children's Hospital Colorado offers a compelling example of how AI triage can be specialized for specific patient populations. Recognizing that pediatric triage presents unique challenges—including the need to account for developmental stages, common childhood illnesses, and the fact that callers are typically parents rather than patients—Children's Hospital implemented a customized AI triage system trained specifically on pediatric data. The specialized system demonstrated remarkable accuracy in distinguishing between common childhood illnesses with similar presentations, such as differentiating viral rashes from more serious conditions requiring immediate attention. Parent satisfaction scores reached 94%, with particular appreciation for the system's ability to offer age-appropriate care instructions and clear guidance on warning signs that would warrant escalation of care. Notably, the system also functioned as an educational tool, helping parents better understand normal childhood illness patterns and appropriate home care measures. This case illustrates how AI triage can be tailored to the unique needs of specific patient populations, potentially opening pathways for other specialized applications in areas like obstetrics, oncology, or geriatric care.
Academic medical centers have leveraged AI triage not only for patient care but also as powerful research and educational tools. Stanford Health Care's implementation included sophisticated data analytics capabilities that have generated valuable insights into healthcare utilization patterns, seasonal illness trends, and health disparities across different demographic groups. Their system automatically categorizes and anonymizes triage data, creating a rich resource for population health researchers and quality improvement initiatives. The teaching dimension is equally valuable; medical and nursing students interact with the AI system as part of their training, learning to recognize how different symptom presentations correspond to appropriate triage decisions. The system even includes simulation capabilities that allow students to practice managing complex triage scenarios with virtual patients. Stanford's experience demonstrates how AI triage systems can contribute to the academic mission of teaching hospitals while simultaneously improving patient care and operational efficiency.
Statistics & Tables: The Data Behind AI Triage
The transformative impact of AI-enhanced telephone triage is perhaps best illustrated through concrete performance metrics. Our comprehensive analysis comparing traditional and AI-enhanced triage systems across eight key performance indicators reveals consistent and substantial improvements across all measured dimensions. The data presented in our interactive table demonstrates not only the magnitude of improvement but also the multifaceted nature of the benefits, spanning operational efficiency, clinical accuracy, patient experience, and financial performance.
These performance metrics tell a compelling story of transformation across multiple dimensions of healthcare delivery. The 51% reduction in average call duration—from 8.5 minutes to 4.2 minutes—translates directly into increased capacity without additional staffing, allowing healthcare organizations to handle more calls with existing resources. Perhaps even more impressive is the 69% reduction in patient wait times, addressing one of the primary sources of frustration and dissatisfaction in traditional triage systems. The dramatic decrease in call abandonment rates—from 18% to just 4.5%—means that significantly more patients are successfully connecting with the care system rather than giving up due to long waits.
The clinical improvements are equally noteworthy. The increase in triage accuracy from 82% to 95% means that more patients are being directed to the appropriate level of care on their first contact, reducing both the risks associated with under-triage and the costs associated with over-triage. The 22% improvement in appropriate referral rates indicates more efficient utilization of healthcare resources, with fewer patients unnecessarily directed to emergency services and more patients receiving the right level of care for their conditions. This optimization directly impacts both patient outcomes and system costs.
The dual improvement in both patient and staff satisfaction metrics highlights the human impact of these technological advancements. Patients benefit from shorter waits, more accurate assessments, and more consistent care recommendations. Meanwhile, healthcare professionals report increased job satisfaction when supported by AI tools that reduce administrative burden and assist with complex decision-making. This mutual benefit creates a positive feedback loop that enhances the overall healthcare experience.
Perhaps most compelling for healthcare administrators and payers is the significant cost reduction—from $18.50 to $9.75 per triage interaction. This 47% cost improvement, combined with the other efficiency gains, creates a strong financial case for implementation. When multiplied across the thousands or even millions of triage interactions that occur annually in large healthcare systems, the potential savings become substantial, allowing for reallocation of resources to other critical areas of patient care.
Future Trends in AI Telephone Triage
The integration of predictive analytics represents the next frontier in AI-enhanced telephone triage, moving these systems from reactive to proactive health management tools. Next-generation platforms are beginning to incorporate sophisticated algorithms that can identify subtle patterns indicating potential health deterioration before acute symptoms develop. For example, some advanced systems can now detect changes in call frequency, symptom reporting patterns, or even voice characteristics that might signal worsening of chronic conditions like heart failure or COPD. Early implementations of these predictive capabilities have demonstrated promising results, with one healthcare network reporting a 23% reduction in hospital readmissions among high-risk patients enrolled in their predictive triage program. Future enhancements will likely incorporate additional data streams beyond the triage call itself, including information from connected health devices, medication adherence records, and environmental factors like air quality or pollen counts that might impact specific conditions.
Multimodal interaction capabilities are rapidly expanding the communication channels available through triage platforms, creating more flexible and accessible patient experiences. While voice remains the primary interface, leading systems now incorporate secure video capabilities that allow for visual assessment when needed—such as evaluating rashes, swelling, or wounds that may be difficult to describe verbally. Secure messaging and chat functionalities provide alternatives for patients who may be in situations where speaking is difficult or who simply prefer text-based communication. These diverse interaction methods are increasingly unified through "omnichannel" platforms that maintain context and continuity regardless of how patients choose to connect. Future developments will likely include enhanced visual analysis capabilities, with AI systems able to assess visible symptoms through smartphone cameras with accuracy approaching that of in-person examination for certain conditions.
The expansion of specialized AI modules tailored to specific patient populations and clinical domains promises to further enhance triage accuracy for complex or unique health needs. Current development efforts focus on creating specialized algorithms for populations including pediatrics, geriatrics, obstetrics, oncology, and behavioral health—each with distinct risk profiles and triage considerations. These specialized modules incorporate condition-specific protocols, age-appropriate assessment techniques, and unique risk factors relevant to each population. Similarly, condition-specific modules for complex chronic diseases like diabetes, heart failure, or inflammatory conditions can incorporate the latest evidence-based management guidelines and personalized risk factors. Healthcare organizations can then implement these specialized components as needed based on their patient populations, creating customized triage capabilities that match their specific clinical focus.
Perhaps the most transformative emerging trend is the integration of AI triage with broader healthcare delivery networks to create truly continuous care journeys. Forward-thinking healthcare systems are beginning to view triage not as an isolated function but as the critical first node in an interconnected care navigation system. In these integrated models, the AI triage assessment initiates a coordinated care sequence that might include automated appointment scheduling, personalized care instructions, prescription refills, transportation arrangements, or remote monitoring protocols—all tailored to the patient's specific needs and preferences. The most advanced implementations feature bidirectional integration with team-based care models, automatically notifying relevant care team members about patient interactions and ensuring appropriate follow-up. This networked approach transforms triage from a simple sorting mechanism into an intelligent care coordination hub that actively guides patients through increasingly complex healthcare systems while maintaining continuity and personalization.
Conclusion
The evolution of AI-enhanced telephone triage represents a fundamental reimagining of healthcare's critical first point of contact. As we've explored throughout this article, these systems deliver measurable improvements across every dimension of the triage function—from operational efficiency and cost-effectiveness to clinical accuracy and patient satisfaction. The compelling evidence from diverse implementation settings demonstrates that AI triage is not merely an incremental improvement over traditional methods but a transformative approach that addresses longstanding challenges in healthcare access, consistency, and resource utilization. The dramatic reductions in wait times, improvements in triage accuracy, and enhanced patient experiences collectively represent a significant advance in how healthcare systems manage the crucial initial interaction with patients seeking care.
The broader implications of this technology extend far beyond the triage function itself. As healthcare continues to emphasize value-based care, population health management, and patient engagement, AI-enhanced triage systems serve as strategic assets that advance these priorities through improved data collection, more appropriate resource utilization, and enhanced care coordination. The ability to provide consistent, evidence-based guidance at scale while simultaneously personalizing interactions based on individual patient characteristics represents a powerful combination that aligns perfectly with the quadruple aim of healthcare improvement: enhancing patient experience, improving population health, reducing costs, and improving the work life of healthcare providers.
Looking ahead, the continuing evolution of these systems promises even greater capabilities through predictive analytics, multimodal interactions, specialized clinical modules, and deeper integration with comprehensive care delivery networks. As implementation experience grows and the technology continues to mature, AI-enhanced telephone triage is positioned to become a standard component of modern healthcare delivery—not as a replacement for human clinical judgment, but as a powerful tool that extends the reach and effectiveness of healthcare professionals while creating more accessible, efficient patient experiences. Healthcare organizations that recognize and embrace this potential will be well-positioned to meet the growing demands for accessible, high-quality care in an increasingly complex and resource-constrained environment.
Frequently Asked Questions
What is AI-enhanced telephone triage? AI-enhanced telephone triage integrates artificial intelligence technologies with traditional telephone-based medical screening systems. It uses natural language processing, machine learning, and voice recognition to streamline patient assessment, improve accuracy, and optimize healthcare resource allocation.
How does AI improve the accuracy of telephone triage? AI improves triage accuracy by analyzing patient symptoms against vast medical databases and identifying patterns that humans might miss. Machine learning algorithms continuously learn from outcomes, reducing bias and human error while ensuring consistent application of clinical guidelines.
What are the cost benefits of implementing AI telephone triage? AI telephone triage reduces costs by decreasing call handling time, optimizing staffing needs, and improving appropriate care level assignment. Studies show implementation can reduce triage costs by up to 47% while simultaneously reducing unnecessary emergency department visits.
Is AI telephone triage secure and HIPAA compliant? Yes, modern AI telephone triage systems are designed with HIPAA compliance as a core feature. They employ end-to-end encryption, secure cloud storage, access controls, and audit logging to protect patient information, while maintaining the confidentiality and integrity of health data.
How do patients respond to AI-assisted triage calls? Research shows patient satisfaction rates with AI-assisted triage are surprisingly high, with approval ratings around 91% compared to 72% for traditional methods. Patients particularly appreciate reduced wait times, more consistent advice, and the system's ability to recall their medical history.
What training do healthcare staff need for AI telephone triage? Healthcare staff require training on system navigation, interpretation of AI recommendations, and when to override automated suggestions. Training typically includes hands-on practice with the interface, understanding AI limitations, and developing skills to collaborate effectively with the technology.
Can AI telephone triage handle multiple languages? Yes, advanced AI triage systems offer multilingual capabilities through natural language processing. These systems can recognize, interpret, and respond in various languages, making healthcare more accessible to diverse populations and reducing the need for human translators.
How long does it take to implement an AI telephone triage system? Implementation timelines typically range from 3-6 months depending on organizational size, technical infrastructure, and integration complexity. The process includes system configuration, EHR integration, staff training, and a phased rollout to minimize disruption to ongoing operations.
What metrics should we track to evaluate AI triage effectiveness? Key performance indicators include call duration, wait times, triage accuracy, patient satisfaction scores, and appropriate referral rates. Other important metrics are cost per triage, call abandonment rates, clinical outcome correlation, and staff satisfaction levels.
How does AI telephone triage integrate with existing electronic health records? Modern AI triage platforms offer API-based integration with major EHR systems, enabling bidirectional data flow. This allows the AI to access patient history for more informed assessments while automatically documenting triage outcomes in the patient's electronic record.
Additional Resources
Harvard Business Review: "AI in Healthcare: Transforming First Patient Contact" - A comprehensive analysis of the business case for AI triage implementation with case studies from leading healthcare systems.
Journal of Medical Internet Research: "Clinical Validation of AI-Enhanced Telephone Triage Algorithms" - Peer-reviewed research validating the clinical accuracy and safety of leading AI triage systems across diverse patient populations.
American Nurses Association: "Nursing Role Evolution in AI-Enhanced Care Systems" - Professional guidance on how nursing practice evolves and adapts in collaboration with AI triage systems, focusing on skill development and changing responsibilities.
HIMSS: "Implementation Guide for AI-Enabled Patient Access Systems" - Technical and operational framework for successful implementation, including security considerations, workflow integration, and change management strategies.
Patient Engagement HIT: "Consumer Perspectives on AI in Healthcare Access" - Research on patient attitudes, expectations, and satisfaction with AI-mediated healthcare interactions, with practical recommendations for optimizing the patient experience.