Advanced Natural Language Processing in Symptom Assessment
Explore the transformative potential of advanced NLP in symptom assessment, moving beyond simple keyword matching to enhance healthcare outcomes and patient experiences.
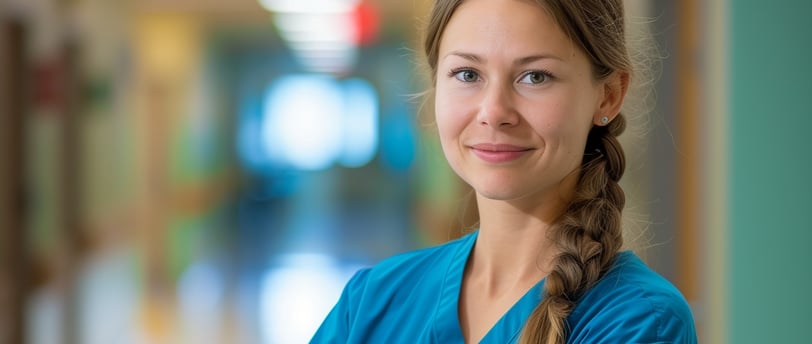
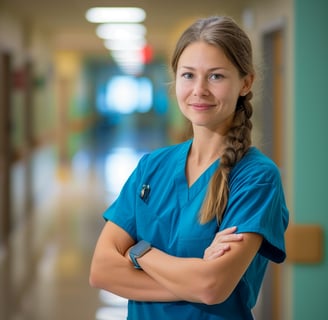
Integrating advanced natural language processing (NLP) is revolutionising symptom assessment in the ever-evolving healthcare landscape. Traditional methods, which often rely on simple keyword matching, give way to more sophisticated techniques to interpret and analyse complex language patterns. This shift is not just about technological advancement; it's about enhancing patient care, improving diagnostic accuracy, and saving lives. This article delves into the fascinating world of advanced NLP in symptom assessment, exploring its applications, benefits, and future possibilities.
Understanding Advanced NLP in Symptom Assessment
What is Advanced NLP?
Advanced NLP uses sophisticated algorithms and models to understand, interpret, and generate human language. Unlike basic NLP, which focuses on simple tasks like keyword matching, advanced NLP can handle complex linguistic structures, context, and semantics. It involves machine learning, deep learning, and neural networks, which enable computers to understand and generate human language in a way that mimics human intelligence.
The Evolution of Symptom Assessment
Symptom assessment has traditionally relied on clinicians interpreting patient descriptions of their symptoms. While essential, this process can be subjective and varied. Advanced NLP brings objectivity and consistency to symptom assessment by analysing patient narratives, clinical notes, and even social media posts to identify patterns and insights that human observers might miss. For instance, NLP can detect subtle linguistic cues that indicate the severity of a symptom or the likelihood of a particular diagnosis.
Applications of Advanced NLP in Symptom Assessment
Enhancing Clinical Decision Support
Advanced NLP can aid clinicians by providing real-time analysis of patient records and symptom descriptions. For example, NLP algorithms can scan electronic health records (EHRs) to identify patterns that suggest a specific diagnosis or predict the likelihood of a particular outcome. This information can then support clinical decision-making, ensuring patients receive the most appropriate and effective care.
In a study published in the Journal of Biomedical Informatics, researchers demonstrated using NLP to analyse clinical notes for signs of sepsis, a severe and potentially life-threatening response to infection. The algorithm accurately identified patients at risk of sepsis, allowing for earlier intervention and improved outcomes1.
Improving Mental Health Assessment
Mental health is an area where advanced NLP can significantly impact. Traditional mental health assessments often rely on self-reported symptoms and clinician observations, which can be subjective and varied. NLP can analyse text data from various sources, including social media posts, clinical notes, and patient narratives, to identify patterns indicative of mental health conditions.
For instance, a study published in npj Digital Medicine used NLP to analyse social media posts for signs of depression and suicidal ideation. The algorithm was able to identify individuals at risk of suicide with high accuracy, demonstrating the potential of NLP to support early intervention and prevent tragic outcomes2.
Supporting Public Health Surveillance
Advanced NLP can also play a crucial role in public health surveillance by analysing large volumes of text data to identify trends and patterns indicative of disease outbreaks or other public health concerns. For example, NLP algorithms can scan social media posts, news articles, and other text data to detect early signs of a disease outbreak, allowing public health officials to respond more quickly and effectively.
In a study published in Scientific Reports, researchers used NLP to analyse mental healthcare text for temporal disease onset information. The algorithm was able to identify the date of psychosis symptom onset with high accuracy, demonstrating the potential of NLP to support public health surveillance and improve outcomes for individuals with mental health conditions1.
Benefits of Advanced NLP in Symptom Assessment
Increased Accuracy and Efficiency
One of the primary benefits of advanced NLP in symptom assessment is increased accuracy and efficiency. NLP algorithms can analyse large volumes of text data quickly and accurately, identifying patterns and insights that human observers might miss. This can lead to more accurate diagnoses, earlier interventions, and improved patient outcomes.
For example, a study published in JAMA Network Open found that NLP algorithms could accurately identify patients at risk of heart failure, allowing for earlier intervention and improved outcomes3.
Enhanced Patient Experience
Advanced NLP can enhance the patient experience by providing more personalised and responsive care. For instance, NLP-powered chatbots can engage with patients in natural language, providing information, answering questions, and offering emotional support. This can help patients feel more supported and engaged in their care, improving satisfaction and outcomes.
In a study published in The Lancet Digital Health, researchers found that patients who used an NLP-powered chatbot to manage their chronic conditions reported higher satisfaction and improved outcomes than those who did not use the chatbot3.
Support for Clinical Research
Advanced NLP can support clinical research by enabling researchers to analyse large volumes of text data quickly and accurately. For example, NLP algorithms can scan clinical notes, research papers, and other text data to identify trends, patterns, and insights that inform research questions and hypotheses. This can lead to more robust and meaningful research findings, ultimately advancing our understanding of health and disease.
In a study published in The Journal of the American Medical Informatics Association, researchers used NLP to analyse clinical notes for insights into managing chronic diseases. The algorithm identified patterns and trends that informed the development of new clinical guidelines, demonstrating the potential of NLP to support clinical research and improve patient care3.
Challenges and Limitations
Data Quality and Availability
One of the primary challenges of advanced NLP in symptom assessment is data quality and availability. NLP algorithms rely on high-quality, well-annotated text data to train and validate their models. However, such data can be difficult and expensive, particularly in healthcare settings where data privacy and security are paramount.
Interpretability and Explainability
Another challenge is the interpretability and explainability of NLP models. While advanced NLP algorithms can achieve high accuracy, they are often viewed as "black boxes," making it difficult to understand how they arrive at their conclusions. This can hinder their adoption in clinical settings, where transparency and accountability are crucial.
Ethical Considerations
Finally, there are ethical considerations when using advanced NLP in symptom assessment. For example, there are concerns about data privacy and security and the potential for algorithmic bias. It is essential to ensure that NLP algorithms are designed and implemented to respect patient privacy, promote equity, and mitigate the risk of harm.
Conclusion
Advanced NLP transforms symptom assessment beyond simple keyword matching to enhance healthcare outcomes and patient experiences. By analysing complex language patterns, NLP can support clinical decision-making, improve mental health assessment, and enhance public health surveillance. However, challenges and limitations include data quality and availability, interpretability and explainability, and ethical considerations. As we continue to explore the potential of advanced NLP in symptom assessment, it is essential to address these challenges and ensure that this powerful technology is used to benefit all patients.